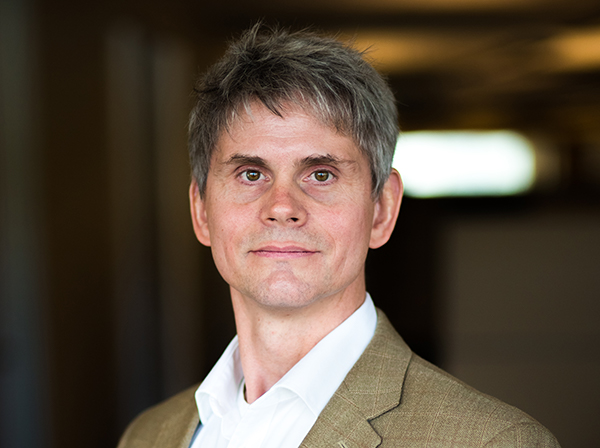
Did you know that only one-third of us can confidently understand, analyze, and argue with data? That’s the essential question posited by the Data Literacy Project, an organization that wants to “ignite discussion and develop the tools we need to shape a successful, data-literate society.”
Achieving consumer Data Literacy at a mass scale is an ambitious and important goal, but in my role as a data scientist, I typically have more tactical conversations on this topic. We need to make sure that the businesspeople charged with digital transformation – the folks on the front lines, with P&L responsibility, under pressure to magically re-orient their companies around data – can communicate about it, and in the right language. I start at a pretty basic level, with what I call the “ABCs of data.”
A Is for Awareness
Data Science and business leaders alike know “garbage in, garbage out,” eruditely defined by the Oxford Reference as a phrase “used to express the idea that in computing and other spheres, incorrect or poor quality input will always produce faulty output.”
Perplexingly, I see business leaders sometimes focused exclusively on the analytic model or artificial intelligence (AI) algorithm they believe will produce the insight they seek, without focusing on the data the algorithm will be fed. Is the algorithm appropriate for the data? Will it meet ethical AI standards? Is there enough data and high-quality data examplars? No matter how innovative the model or algorithm, it will only produce results that are as accurate and unbiased as the data it consumes.
A modern Data Science project, therefore, is a lot like an old-fashioned computer programming project: 80% of the time should be spent on gathering the proper data and making sure it is correct, admissible, and unbiased.
While the 80% yardstick itself isn’t new, data usage and data standards are changing –and they are complicated. Companies should formalize their model governance standards and enforce them ahead of admitting data for a project, because customer data is not free from usage constraints. Companies must conform with regulations concerning customer consent and permissible use; increasingly, customers have an ability to be forgotten, or their data to be withdrawn from future models.
In short, customer data can be riddled with quality issues and biased outcomes, and can’t be used in the freewheeling ways and academic pursuits of decades past. Business leaders must be aware of these important facts, and cognizant of their company’s very strong governance around data and AI. If governance isn’t established, it needs to be.
B Is for Bias
Biased data produces biased decisions – perhaps best paraphrased as “producing the same old garbage.” Organizations and data scientists must recognize that if they build a model to exactly replicate bias, even inadvertently, their work product will continue to propagate bias in an automated and callous fashion.
There are helpful guidelines, for example, to help compliance officers avoid biased and other unethical uses of AI. Because bias is rooted in data, the best default is to treat all data as dirty, suspect, and a liability hiding multiple landmines of bias. The data scientist’s and organization’s job is to prove why their usage of specific data fields, and how the algorithms leveraging them, is acceptable.
It’s not an effortless task. Aside from obvious data inputs, such as race or age, other seemingly harmless fields can impute bias during model training, introducing confounding (unintended) variables that automate biased results. For example, cell phone brand and model can impute income and, in turn, bias to other decisions, such as how much money a customer may borrow, and at what rate.
Furthermore, latent (unknown) relationships between acceptable data can also unintentionally impute bias. These dirty patterns hidden in data are not in full view, and machine learning models can find them in ways that human scientists will not anticipate. This is why it is so important for machine learning models to be interpretable and expose learned relationships, and not rely on the stated importance of data inputs in a model or derived from an arbitrary explainable AI algorithm.
Finally, data that may not introduce bias today might in the future – what is the company’s continual data bias monitoring policy? Today, many organizations don’t have any plan.
Clearly, there are many issues around data to consider, and be understood, by data scientists and business leaders alike. Policies around data usage and monitoring are pillars of a strong AI governance framework, a template for ethical use of analytics and AI by the company as a whole. These policies include establishing methods to determine if data is biased because the collected sample is inaccurate, or the wrong data is being sourced, or simply (and sadly) because we live in a biased world. Equally important, how does the governance framework additionally provide for identifying and remedying bias?
C Is for Callousness
Bottom-line business leaders are looking for the decision an analytic model will make and to automate it in AI. In the rush to seize the business insight from an analytic model and automate it, companies often are not building models robustly. They are neither scenario testing nor bias testing. These mistakes are to the detriment of the customers whom companies are trying to serve, because once the data and analytics are complete, business leaders are presented with a score that will operationalize decision-making. Score-based decisioning enables automation, but also facilitates automated bias at scale. Business leaders must be sensitive to the potential callousness of decisioning based on an abstracted score.
For example, COVID has unleashed some level of economic despair on every corner of the planet. Data has shifted, exposing the fact that many businesses don’t understand the impact of changes in customer data, performance data, and economic conditions have on their model scores, and how to use them in automated decisioning. Callous busines leaders are those who stubbornly continue to apply model scores because “the model told me,” versus looking at how data and situations have changed for groups of customers, and adjusting their use of models in business strategy.
We must also ensure those decisions are properly recorded. For example, a customer may have purchased a new phone from the wireless service provider just prior to COVID. If that customer stops paying, how is that decision recorded, as fraud or credit risk default? Are certain groups of customers during COVID more suspectable to job loss due to their profession? Do we find that socioeconomic, ethnic, or geographic bias is driving credit default or fraud rates due to sloppiness in labeling outcomes, plain and simple?
When bias, carelessness, or abject callousness is employed in dispositioning cases, it results in even more bias as future generations of models are developed. I routinely see this chain of events in situations where credit risk default gets labeled as fraud. Certain groups of customers credit-default more than others due to profession or education; when they are mislabeled due to careless, callous, or biased outcome assignments, entire groups of customers are pigeonholed as more likely to have committed fraud. Tragically, organizations are self-propagating bias in future models through this callous assignment of outcome data.
In short, a model is a tool, to be wrapped in a comprehensive decisioning strategy that incorporates model scores and customer data. “When should we use the model?” and “When should we not?” must be questions understood by business leaders as data shifts. Equally important is the question, “How do we not propagate bias through callous outcome assignments and treatments?” The answers to these questions build a foundation for stopping the cycle of bias.
All Together Now
While the decisions rendered by analytic models are often a binary “yes” or “no,” “good” or “bad,” the issues around the proper use of data are anything but – they are complex, nuanced, and cannot be rushed. As companies increasingly recognize that Data Literacy is the gateway to digital transformation, I am hoping that, over time, data scientists and business leaders can be on “the same (Data Governance) page” of a metaphorical corporate songbook: “Now I know my data ABCs, next time won’t you sing with me?”