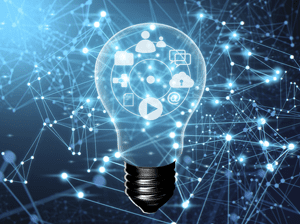
Trusting big data requires understanding its data lineage. Without data lineage, big data becomes synonymous with the last phrase in a game of telephone. The original data from the first person (e.g., “a guppy swims in a shark tank”) changes to something completely different when it ends with the last person (e.g., “The puppy that spins and barks, stank”). Telephone game players look perplexed with no understanding of how the original data came to be something completely different. Such is the case with bad data lineage as well, as an enterprise’s data assets flow through its Data Architecture.
Customers, regulators, and businesses find it less entertaining to play the telephone game upon using a business’ big data. According to Stewart Bond of IDC, businesses need data that is secure and compliant. This data needs to be available when and where it is needed. This need for clean big data becomes further complicated with multiple end-users, platforms, and sources in various formats, such as video, text, images, and audio. By storing big data remotely, in the cloud, it becomes less tangible how it got there. Understanding data lineage addresses these types of problems and more.
What Is Data Lineage?
Data lineage describes data origins, movements, characteristics, and quality. According to Stewart Bond, lineage typically describes where the big data begins and how it is changed to the final outcome. Technology projects have used this traditional approach to data lineage. For example, during the creation of a new clinician/patient system, at a large technology company, project members would refer to a map of tables and joins, to guide what SQL to use for selecting, summarizing, or grouping the data. Programmers would update the code to generate the needed values and QA would read these plans to anticipate ways to break the software. While this method was a start, data lineage needs an expanded definition.
In only applying the traditional approach to data lineage, data encounters roadblocks, especially master data: information about people, processes, and things that form the business core. For example, team members must develop a new checking program for a large bank division handling foreign transactions. QA and software engineers run into issues obtaining a valid set of test data from other bank divisions. Had project managers included additional data lineage facets, such as who uses the big data, what it means, when the data is accessed, why the data is stored, and how the data elements are related, these obstacles could have been mitigated, shortening the time frame for development and testing. Meaningful data lineage needs to contain multiple dimensions: who, what, where, why, and how.
Why Keep Track of Lineage?
Data lineage has many benefits, including:
- Data Governance: According to Christian Bremeau, CEO and president of Meta Integration Technology, Data Governance requires metadata management. This is needed to ensure big data meets business standards: “The mission of a metadata management solution is to go to the absolute source of wherever it’s coming from to the end at the other side,” said Bremeau. A data lineage solution stitches metadata together providing “understanding and validation” of data usage and risks that need to be mitigated.
- Compliance: Multiple different stakeholders, including customers, staff members, and auditors, need to trust reported data while quickly responding to business opportunities and regulatory challenges. They need to know for a report, “How did the information get …[there]?” Tracking data lineage provides proof that the “reports properly reflect the data,” according to Ian Rowlands, former VP of Product Marketing at ASG Technologies.
- Data Quality: Challenges to Data Quality include data movement, transformation, interpretation, and selection through people and processes. “Businesses today are under pressure to reliably demonstrate data’s origin and transformation through the organization,” says Rowlands. A data lineage solution provides the ability to know when “at the end-to-end flow,” encompassing: when data has been transformed, what it means, and how the Data Quality moves from one place to another.
- Business Impact Analysis: As specified by Bond, businesses need to understand how internal departments and users, as well as external customers, share big data, especially master data, and how this data changes. As Bremeau stated, a colleague may ask why a bad decision was made some quarter in the past, e.g., Q4 2005. Likewise, businesses may wish to upgrade the data warehouse and need to know what systems and processes could break doing this. Responding to these types of questions requires going back and forth in time with your data, which necessitates understanding the data lineage.
How to Create and Use Data Lineage in Your Business
To make better decisions and respond more rapidly to business opportunities and regulations, businesses must create and use data lineage effectively. Good strategies include:
- Document the Where and How of Your Data: Break down where data might live in the business including through key business processes and flow between these processes. Also, know the technical lineage or “The flow of physical data through underlying applications, services, data stores,” says Rowlands. Track where data has moved and how it has changed, in a repeatable, defensible, and speedy manner.
- Investigate the 5 W’s: As mentioned above, meaningful data needs to be multidimensional, beyond the where and how. Find out who is using the data, what it means, when it was captured, when it is being used, and why it is stored and/or used.
- Understand Relationships: Relationships between data need to be well understood, including how data originates and moves between people, processes, services, and products. Data managers need to conceptualize this information from the internal entities (such as departments within a business), external players (buyers from and sellers to the business), and the interaction between the internal entities and external players.
- Automation: As Bremeau mentioned, “Maintaining semantic mapping by hand is a nightmare. What you want is a set of tools to do that automatically.” Identifying critical or master data and using an automated metadata application to scan and gather metadata about data lineage becomes essential.
Case Study: The Financial Industry and Data Lineage
Data lineage has become essential to the financial industry, especially since regulatory controls changed as a reaction to the 2007-2008 financial crisis. A case study between a prominent bank and ASG Technologies (now Rocket Software) describes how one bank took a proactive strategy to, “Create a world-class process and strategy to automate the data forensics and resolve regulatory requirements across the organization.” The bank’s Information Architecture (IA) team explored a range of tools and did “proof of concept trials with three vendors, including a portion of the ASG solution,” for the retail banking division.
Approaches explored included mainframe testing, a distributed environment and migrations, and conversions. The IA team concluded that ASG’s solution provided the “speed of results and overarching ramifications” required to meet its goal. The success of ASG’s solution, for the bank included:
- Cost savings in completing data lineage on “10 Key Business Elements (KBEs) in 100 applications, from $1,480,280 to $304,140.”
- Increased efficiency by “80-fold over manual data lineage and analysis processes.”
- Speedier resolution of one “data element in 100 systems (40 simple, 40 medium, and 20 composite) in 180 hours vs. 14,400 hours when performed manually.”
Moving forward, the bank’s IA team planned to continue with ASG’s solution executing data lineage, including a “second implementation stage of 1000 KBEs in 40-50 systems.” As this case study shows, the power of data lineage minimizes doubts, increases trust, and speeds the processes.
Image used under license from Shutterstock.com