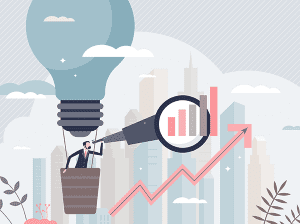
The use of Data Governance metrics as a measurement system promotes the efficient use and management of data.
Establishing key performance indicators (KPIs) is normally the first step in monitoring and measuring the effectiveness of a Data Governance program. These indicators allow organizations to assess and adjust their Data Governance strategies. A measurement system allows a business to develop a strong data-based culture and maximize the value of its data.
Data Governance deals with Data Quality, compliance, security, and accessibility. Data Governance metrics improve efficiency in these areas.
Effective Data Governance strategies are necessary for organizations to maintain compliance with various regulations, protect their customers’ data, and promote the use of reliable data. The use of Data Governance metrics allows businesses to ensure regulatory compliance and high Data Quality. Key performance indicators are put in place to measure how effective an organization’s Data Governance program is, and how well it is being supported.
Data Governance programs are affected by three primary influences: business processes, people, and software. Because Data Governance is a complex system that can be difficult to measure, it is important to consider each of these influences when using performance indicators. Measuring the progress of a recently implemented Data Governance program is important in determining the effectiveness of the training and changes in business practices, and how well the program is working.
When you focus on KPIs for Governance and compliance, you are able to determine how effective your processes are and how they may need to be adapted over time. After all, creating a Data Governance policy and hoping for the best is not a recipe for success, as you can never address issues that you are not aware of. Data Governance metrics can be used to:
- Identify software, business processes, or individual behavior needing changes
- Highlight the program’s effectiveness in areas like regulatory compliance and Data Quality
- Uncover areas needing improvement in the Data Governance program
- Show the success of the Data Governance program to stakeholders
Without the use of Data Governance metrics, a business can allow best practices and strategies to flounder and fail. Metrics and performance indicators can help to ensure an organization maintains the standards needed for developing a successful Data Governance program.
Data Governance Metrics and Key Performance Indicators
There are several areas that can be measured, ranging from regulatory compliance to data consistency to data accuracy. Typically, a sample of the data is examined for problems. The same sample can be used to measure different aspects of the data’s quality. But what is being measured does vary.
Finding patterns within the data having low quality can be worthwhile. If one or two individuals are responsible for poor Data Quality, this can be discovered, and those people can be retrained. The commonly used key performance indicators for Data Quality are listed below.
- Data accuracy: A measure of the data’s accuracy. A sample of the data (say 20%) is compared to a trusted source to determine what percentage of the sample is faultless and what percentage contains errors.
- Data completeness: An assessment of how complete the data is. Using standards and requirements the organization has decided are appropriate, the sample is examined for missing bits of information (partial addresses, missing email addresses, etc.).
- Data consistency: A measure of the data’s uniformity (or lack of uniformity) from different data sources – department to department, file to file. For example, if old, no-longer-accurate data has been saved (or never updated in a different department), goofy mistakes can be made, damaging customer relations.
- Data timeliness: Tracks the amount of time the computer system takes in capturing and processing data. This inspection is concerned with data/information that is outdated and no longer valid. While some data can remain valid for years, other data can become outdated (and potentially dangerous, if used) in a few hours. Data timeliness is normally used to ensure the data remains up-to-date and valid.
- Uniqueness: This process ensures that there are no unnecessary duplicate records in storage. Uniqueness is one of the most important features used to ensure Data Quality. Data uniqueness can be measured by querying a certain number of file names and discovering how many copies exist and where they are located.
Additionally, there are less commonly used key performance indicators. These indicators cover areas such as security, regulatory compliance, and other less obvious concerns. They are available below.
- Security
- Data encryption: A measure of how much (the percentage) of confidential or sensitive data in storage that is encrypted, safeguarding it from unauthorized access.
- Data breach incidents: The number of data breaches can be used as a crude measure of the security program’s efficiency. (If management is being approached for improved security, the number of data breaches can make for a very good argument.)
- User access monitoring: Access logs and user activity are monitored and measured to make sure only authorized users have access to sensitive data and can be used to identify potential security risks.
- Data Compliance
- Data access controls: The effectiveness of access controls is monitored to ensure personal and sensitive data can be accessed only by authorized individuals.Regulatory compliance: The organization’s compliance with data privacy regulations, such as GDPR or CCPA, is measured.
- Data retention: Some data/information must be stored for a certain period of time, per legal requirements or the organization’s own policies. This is referred to as data retention, and monitoring it can ensure the data is saved for the necessary time period.
Other Data Governance Performance Metrics and Performance Indicators
With a little imagination, the use of performance metrics can be applied to a variety of situations. The trick is figuring out what to use as performance indicators. The following list provides examples.
- The use of data assets and adoption rate: A measurement of how people are using data assets over a period of time. This metric focuses on the effectiveness of the strategies being used to promote a data-driven culture as part of implementing a Data Governance program. It can be measured by tracking how many data assets have been accessed and how many times they have been accessed. It’s also possible to track who is using it, and who isn’t. Data lineage software can be used for this purpose.
- The rate of data incidents: Data incidents include data losses, data breaches, data inaccuracy incidents, and any other data event having a negative impact on the organization. A low rate of incidents is the goal, and indicates accurate data and good data security. This minimizes business risks that are associated with mishandled data. Measurements are based on the number of incidents occurring over a specified period.
- Data compliance with the organization’s standards: Data uniformity supports the easy use of data throughout the organization. This can be measured by performing audits or using automated checks on various datasets to find and identify non-conforming data.
Conclusion
In her article What Is Data Governance? Definition, Types, Uses, Michelle Knight writes, “Data Governance (DG) is a business program and bedrock that supports harmonized data activities across the organization. It accomplishes this goal as a formalized framework implemented to the specifications of a corporate Data Strategy.”
An intelligent, well-maintained Data Governance program streamlines data business processes and promotes success. However, a poorly maintained program will not protect data, nor will it assure that laws and regulations are followed. The use of metrics helps in developing that intelligent, well-maintained Data Governance program.