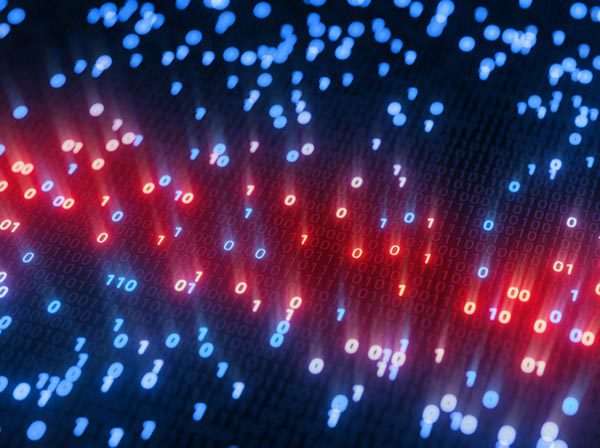
Top management are pressuring their organizations to adopt advanced AI-enabled solutions, so Data Management challenges continue to mount on technology teams. The technology decision-makers are increasingly realizing that without a firm Data Strategy provisioning enterprise-wide supply of data on demand, machine learning and AI adoption will remain just a buzz. For most businesses today, this means a Data Governance overhaul.
However, according to How Can Machine Learning Affect Your Organizational Data Strategy, machine learning (ML) solutions can deliver “the intended business outcomes” only when the organizational Data Management landscape—at the core of which is the Data Governance—is solid and transparent.
Is Machine Learning Important to Organizational Data Strategy?
Data Strategy and Machine Learning: How Do They Intersect?says that organizational Data Strategy rests on four pillars: Data Quality, Data Security, Data Stewardship, and Data Governance, without which even the best of ML technologies or platforms cannot deliver the intended business outcomes.
The recent technological advances like Big Data, IoT, and machine learning have necessitated further tuning of Data Governance practices throughout global businesses. As the trend toward self-service analytics and BI continue, it will become apparent that Data Quality and Data Governance (DG) will occupy critical places in the organizational Data Strategy framework.
As observed by this KD Nugget author, ML-driven analytics and BI systems will reduce or remove the need for in-house Data Science teams, thus increasing the workload for Data Governance systems. To ensure that data is of the highest quality to work in automated analytics and BI environments, future data strategists will have to align the Data Management goals with advanced technology (machine learning) goals and practices.
According to a Survey conducted by Forbes Insights on C-Suite Executives:
- 70 percent of the surveyed C-Suite executives stated that their top management is advocating increased AI use
- Over 90 percent of the surveyed C-Suite executives agree that AI adoption will help their businesses stay ahead of competition
- 80 percent of the surveyed C-Suite executives believe that only 40 percent or lower amounts of enterprise data are actually available for sharing.
The general mood of this survey report indicates that only advanced AI and machine learning systems, such as Dell Technologies Digital Transformation solutions powered by Intel® can greatly aid businesses in achieving their Data Management goals.
How Important Is Data Governance to Machine Learning?
The permanent problem: enterprises will continue to acquire high volumes of data—from traditional sources as well as digital channels such as mobile, social, and IoT.
A recent article on governance and machine learning aptly describes how the varied data sources and data types have added to the data mess already created by massive volumes. The article claims that in today’s competitive business world, a strong Data Governance will help “strike a balance between Data Governance and the ML capabilities.” As the typical data invading advanced AI systems is viewed as being “disruptive,” Data Governance is critical for the success of any Data Management project.
The skepticism around ML “black box” is still there, and recent regulations like GDPR raise suspicions about the lack of transparency in the ML world. The good thing is that such regulations are tightening the noose around Data Governance models, and only highly effective ones will help achieve the needed Data Strategy.
The Q&A on 2019 Trends in Data Governance captures these observations:
- Model governance needs to ensure that ML models adhere to same guidelines in terms of roles, responsibilities, and rules as other DG entities
- DYI open-source solutions will be available for model governance, which organizations will be able to use without external support.
- The future success of DG will depend on data lineage, metadata, and compliance.
Big Data of the future will require a centrally governed Data Management solution to provision ML-enabled decision-making solutions.
Machine Learning Seen as an Ultimate Solution to Data Governance
While various U.S states are focusing on data privacy laws to aid businesses, the technology sector is exploring other ways of enabling Data Governance in industry sectors.
The advanced AI system providers seem to think that only ML-powered solutions will ultimately satisfy both the regulatory and compliance requirements. Let’s take the example of the banking sector. Currently, the lack of consistency in data definition and quality is a serious deterrent to business operations across the enterprise. ML can help solve regulatory and compliance issues, specifically those related to Data Governance and data security and privacy, faced by different divisions within an enterprise.
Now with General Data Protection Regulation (GDPR) requirements in most parts of the world, the advanced technologies are viewed as welcome transitions in global businesses.
The recurrent theme in Data Governance in recent conferences has been the role of metadata in DG. The future of Data Governance, according toMetadata and Machine Learning in Data Governance, is a centralized Data Strategy to promote democratic decision-making across the enterprise.
Gartner believes that by 2020, at least 50 percent of Data Governance policies will be driven by metadata. The greatest strengths of metadata are the implementation of accountability at every step, a common vocabulary, and an auditable process for compliance. Then ML technologies can move from the science labs to business halls.
The DATAVERSITY® webinar on automated Metadata Management goes into this in more detail.
Data Strategy Forms the Core of Advanced AI Systems
Advanced technologies need a well-defined Data Strategy to deliver benefits to businesses.
A Forbes post explains how a “data strategy drives every AI strategy.”
Surprisingly, during a survey of business executives, only 12 percent of the respondents acknowledged the presence of a defined Data Strategy in their enterprises. Given the phenomenal importance of data in all competitive intelligence practices, it is shocking that such a small percentage of businesses have actually implemented the fundamental data management practices, the most important of which is Data Strategy. Without a sound Data Strategy, these business operations will fail to reap the benefits of advanced AI technologies.
Data Strategy Case Studies: Getting Your Data House in Order
In any enterprise Data Strategy, it is imperative that the organizational “operating structure” is aligned with the data model. This McKinsey Report discusses the transformative Data Strategy of a major U.S. bank. The implemented Data Strategy, along with the Data Governance model, ensured improved human capital management, budgeting, and overall governance. This scalable technology solution is expected to help the bank gain $2 billion in benefits.
In another instance, an oil and gas company achieved about 12 to15 percent increase in profit margins, simply by segregating critical data channels and streamlining the flow of data in the supply chain. Data lakes and flexible storage models, for example, are eliminating the cost of normalizing data for central storage
With collaborative technologies like Big Data, data lakes, and IoT, business leaders and operators in the retail, telecom, insurance, and pharmaceutical sectors want to embrace “high-performing” data management solutions to increase revenue and reduce risks.
Data Governance and Machine Learning: The Inseparable Duo
A modern enterprise cannot claim to be data-driven unless it has implemented the right Data Management infrastructure to support advanced AI systems. All technology-supported decision-making and competitive insights can be extracted from advanced technologies, if and only if the basic, end-to-end data flow is controlled, monitored, and then delivered to the analytics or BI systems.
Image used under license from Shutterstock.com