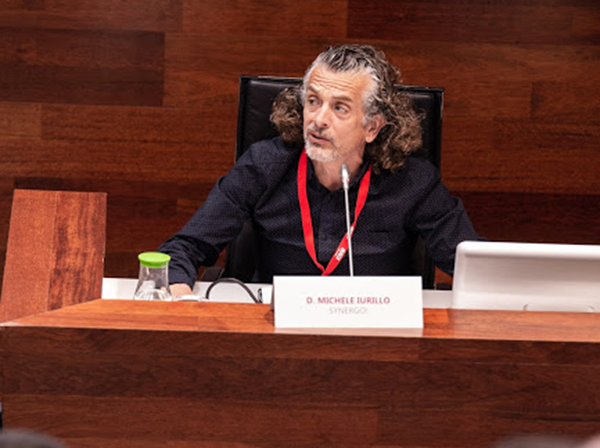
Click to learn more about author Michele Iurillo.
According to Gartner, data fabric is an architecture and set of data services that provides consistent functionality across a variety of environments, from on-premises to the cloud. Data fabric simplifies and integrates on-premises and cloud Data Management by accelerating digital transformation. How are we going to convince enterprises that data is absolutely traversal? How can we perform a solid data valuation? Can data fabric help us in this? Can we subdue the data silos?
Gartner defines data fabric as a design concept that serves as an integrated layer (fabric) of data and connecting processes. A data fabric uses continuous analysis on existing metadata assets to support the design, deployment, and use of integrated and reusable data across environments, and is a must for data-driven organizations: “The data structure approach can enhance traditional Data Management patterns and replace them with a more responsive approach. It offers D&A managers the ability to reduce the variety of integrated data management platforms and offer cross-enterprise data flows and integration opportunities.”
This is why it is necessary to have an all-in-one approach – that is, a platform that can operate across the entire data pipeline, from data ingestion to data exploitation and visualization.
A totally virtual approach (a LDW system based on queries) has the limitation of not being able to materialize all the processes and above all does not allow a complete audit over time and in highly regulated environments such as banking and insurance. Logical data warehouse is an approach that can solve some specific requirement but has no place in structured processes. The regulator may not only ask us how a certain extraction process is performed and its lineage, he may also want to see the replication of a certain process on a specific date to see all the transformations and all the processes that have been involved.
Against Tools Patchwork
Normally, when we approach a company for any type of data project, we find a typically fragmented scenario. Companies often incorporate tools according to a rather commercial logic of the historical moment of the company. So it is normal to find a patchwork of many different tools: We will have data sources, different data warehouses from different vendors, analytical engines, reporting engines, OLAP cubes, and more. In the best-case scenario, they can come from the same vendor, but there are still a few issues to deal with. How do we do workflow automation? How do we manage metadata? How do we document processes? What about accountability? How do we respond to the regulator? That’s when we ask ourselves at the architecture level that maybe we should have done it differently.
An Enterprise Data Management (EDM) approach, where all data assets are concentrated on a single platform, would be the optimal solution. Also, according to DAMA, the elimination of silos and full accountability should be at the core of any data project. Can the data fabric concept be a solution? According to Gartner, data fabrics reduce integration design time by 30%, deployment by 30%, and maintenance by 70%, as technology designs are based on the ability to use/reuse and combine different styles of data integration. In addition, data fabrics can leverage the existing skills and technologies of data hubs, data lakes, and data warehouses, while introducing new approaches and tools for the future. In this sense, although a good approach is to have an all-in-one platform with full interoperability capabilities, implementing a data fabric does not require any of the customer’s technology investments.
In the Name of Metadata
At least three of the closely interconnected pillars identified by Gartner for the data fabric relate directly to metadata:
- Augmented data catalog: a catalog of available information with distinctive features intended to support an active use of metadata that can ensure maximum efficiency of Data Management processes;
- Semantic knowledge graph: graphical representation of the semantics and ontologies of all entities involved in the management of data assets; obviously, the basic components represented in this model are metadata;
- Active metadata: useful metadata to be analyzed in order to identify opportunities for easier and optimized treatment and use of data assets: log files, transactions, user login, query optimization plan.
The metadata-centric data fabric provides us with all the other advantages that are very important when prioritizing measures on data assets. If we focus on being able to give an internal value to our data based on different parameters, we will be able to dedicate resources in a way that is “based” on the intrinsic value of the data. Imagine how much a customer’s data can be worth, when this value grows according to its importance, its portfolio, and its sales history, when this value decreases according to how inaccurate the information we have about it can be, how much poor management of its data can cost according to regulatory obligations.
If we give a value to all the data, and especially to the metadata, we will be able to answer very interesting questions such as: Which are the data owners who manage the most valuable data for the company? How should we prioritize quality actions according to the value that these data assets represent? If a governance tool has the Governance by Design paradigm, it allows us to give an internal (i.e., of the organization) and external value depending on the loss of this asset or the sale of it. How much is the customer’s data worth to our competitor? In the book “Infonomics” by Doug Laney, we find dozens of examples of how we can give value to data. Maybe you should do it from the metadata, so it is necessary that the Data Governance tool or suite can enrich the metadata with other attributes without losing the lineage of the data.
Gartner recommends that data and analytics leaders do not acquire technologies that do not have AI and ML development and inclusion in their roadmap.
Perhaps they should also advise that it’s time to opt for converged technologies – even if this goes a bit against their vision of solutions divided into a “Magic Quadrant.”