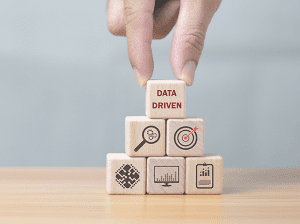
Data-driven decision-making involves utilizing data and analytics to solve business problems. Relying on facts rather than intuition or personal opinions can lead to more accurate and effective decisions.
In business organizations, evidence-based decisions can optimize operations, enhance productivity, and drive growth. By examining trends, patterns, and correlations within datasets, business operators can uncover valuable information that helps identify opportunities for improvement or potential risks. Moreover, data-driven decision-making fosters a culture of accountability within organizations as decisions are based on quantifiable results provided by human analysts.
What Is Data-Driven Decision-Making?
The key components of the process are data collection, interpretation, and analysis. These components work together to deliver valuable insights and guide decision-makers toward making informed choices.
The first component, data collection, refers to the systematic gathering of relevant information. This can include quantitative data from surveys or experiments, as well as qualitative data from interviews or observations. The collected data must be accurate and comprehensive to ensure reliable analysis.
The next step is data interpretation. This involves organizing and structuring the information in a meaningful way. Interpretation helps identify patterns, trends, and correlations within the dataset, enabling decision-makers to extract valuable insights.
Where Do Data-Driven Decisions and Analytics Intersect?
During data analytics, data-enabled insights are extracted through various analytical techniques. In descriptive analytics, historical data is used to gain insights into past trends and patterns.
In predictive analytics, statistical models and machine learning (ML) algorithms are utilized to forecast future outcomes based on historical data.
Organizations use data-driven insights to make more accurate predictions, optimize performance, and develop strategies that align with market demands. This approach ensures that investments are made in areas with the highest potential for return on investment (ROI), improving overall business performance.
What Are the Benefits of Being Data-Driven?
Data-enabled decision-making is the key to fact-based analysis – ultimately leading to measurable outcomes. Here’s a quick roundup of specific business benefits of data-driven decision-making:
- The primary benefit is the ability to minimize risks. By relying on comprehensive data analysis, organizations can identify business risks and mitigate them before they become major issues.
- One of the key benefits of data-driven decision-making is cost savings. This could include identifying bottlenecks in the supply chain or eliminating redundant activities. However, the advantages extend beyond monetary savings.
- The next benefit is that businesses can identify opportunities for growth and innovation. By analyzing patterns or trends in customer preferences or market demand, companies can tailor their products accordingly, resulting in increased customer satisfaction and improved competitiveness.
- Data-driven decision-making empowers businesses to target their customers based on customer preferences and previous purchase patterns. A targeted approach not only enhances customer satisfaction but also increases the likelihood of repeat business.
- The marketing benefit of this process is that businesses can identify the channels with maximum ROI and then engage their customers through those channels. This enables them to allocate resources wisely and optimize marketing efforts.
- Ultimately, data-driven decision-making fosters a culture of accountability within an organization.
What Are the Challenges Involved?
One of the main hurdles is ensuring the reliability and quality of the gathered data. Different departments within an organization may use different systems or tools to collect and store data, making it challenging to consolidate information effectively. This fragmentation can lead to incomplete or inconsistent datasets, which can compromise the accuracy of decision-making processes.
The other potential data-management hazards or challenges may be summed up as:
Resistance to change: Implementing data-driven decision-making strategies can be a challenging process, particularly due to the resistance to change that often accompanies it. Overcoming this resistance is crucial for successful implementation.
Probable solution: The business leadership has to address the fear of uncertainty and job insecurity among employees. Also, a shift in mindset from “traditional decision-making practices” relying on intuition or personal experience is required to overcome resistance. Encouraging open dialogue, rewarding data-backed insights, and sharing solutions can help shift attitudes toward embracing data-driven strategies.
Lack of data accuracy: Data collection process requires overcoming potential biases and errors. Human errors during manual data entry or transcription can introduce inaccuracies into the dataset. Biases might arise due to subjective interpretations or selective sampling methods employed during the collection process.
Probable solution: Implementing automated tools for data integration and cleansing can enhance the reliability of collected information by reducing human errors.
Data decay: This happens when information becomes outdated or irrelevant due to changes in business processes or external factors. Regular updates and maintenance are necessary to prevent such decay and ensure that decisions are based on current and most relevant information.
Probable solution: To overcome this challenge, organizations must invest in robust data governance (DG) frameworks that establish standardized procedures for collecting, validating, and managing data.
Complex data sets: Big data, characterized by complex datasets, can be a key challenge faced in data-driven decision-making. As modern organizations collect vast amounts of structured and unstructured data from various sources, managing this data becomes a series of challenges, one of which is the sheer volume and variety of data.
As organizations accumulate more and more information, the challenge lies in filtering through this overwhelming amount of data to extract relevant insights. Moreover, organizations need skilled professionals who can navigate through complex datasets using advanced analytics techniques.
Data security and privacy: Data security involves safeguarding sensitive information from threats such as cyberattacks, hacking attempts, or insider threats. Organizations must take measures to protect sensitive information from unauthorized access or breaches while complying with regulatory requirements.
Probable solution: It requires implementing robust security measures such as encryption techniques, firewalls, and access controls to prevent unauthorized individuals from accessing or tampering with the data.
Finally, organizations should adopt transparent policies regarding how they handle personal data and provide individuals with options for controlling their own information. Regular audits and assessments should be conducted to ensure compliance with privacy regulations and identify any gaps that need attention.
What Are Data-Driven Decision-Making Best Practices?
Today, organizations acknowledge the importance of making decisions based on solid evidence rather than intuition or guesswork. However, process implementation requires strict adherence to some best practices for data-driven decision-making to be successful.
Best practices for implementing the data-driven decision-making process:
- Organizations need a culture that values data and encourages its use in decision-making. This includes fostering a mindset where employees understand the significance of data, are comfortable working with it, and have access to relevant tools and resources. Additionally, leadership plays a vital role in promoting data-driven decision-making by setting clear expectations and providing the necessary training to all employees.
- Every organization needs to create a solid Data Strategy for implementing data-driven decision-making. The strategy roadmap usually includes the most important key performance indicators (KPIs). By defining measurable objectives, businesses can track progress and evaluate their performance accurately. It is important to select KPIs that are relevant, attainable, time-bound, and align with strategic objectives.
- Organizations should invest in robust data collection methods and technologies to ensure accurate information gathering. Without proper data integration processes in place, data from disparate sources may be fragmented or incompatible, leading to unreliable insights and flawed decision-making. Regularly reviewing data integration and Data Quality processes is essential for maintaining reliability throughout the decision-making process.
- The organization’s leaders need to leverage predictive modeling and ML algorithms to extract accurate insights from vast amounts of data. By utilizing ML algorithms, businesses can uncover hidden patterns in big datasets that might not be apparent through traditional analysis methods.
- Organizations can uncover actionable insights through data visualization techniques. When data is viewed in visual formats such as charts, graphs, or interactive dashboards, complex information becomes understandable. It is also easier to share data among business peers when data is presented in clear, visual formats.
What Are Data-Driven Decision-Making Tools?
Big data tools play a crucial role in managing and analyzing vast amounts of structured and unstructured data. These tools enable organizations to gather, store, process, and visualize large datasets efficiently. By harnessing the power of these tools, decision-makers can gain a comprehensive understanding of their business landscape.
In the age of data-driven decision-making, organizations are increasingly turning to predictive modeling tools to gain valuable insights. By leveraging historical data and advanced statistical techniques, these tools enable businesses to forecast future outcomes and make informed decisions.
Currently, organizations are increasingly relying on AI and NLP to enhance their decision-making processes. AI algorithms are designed to analyze complex datasets, identify patterns, and predict outcomes. By applying AI to decision-making, organizations can automate repetitive tasks, reduce human biases, and uncover hidden relationships within the data.
NLP processing complements AI by enabling machines to understand and interpret human language. With NLP algorithms, organizations can extract meaning from unstructured text such as customer feedback, social media posts, or industry reports. By analyzing this textual information in conjunction with quantitative data, businesses gain a holistic understanding of their customers’ sentiments and preferences.
Reporting software enables organizations to collect and analyze data in a single location, and that too, in real time. These advanced reporting tools provide real-time access to KPIs, allowing decision-makers to monitor progress and identify areas for improvement. With customizable dashboards and interactive visualizations, reporting software empowers users to quickly identify trends, patterns, and anomalies that may impact decision-making.
Image used under license from Shutterstock