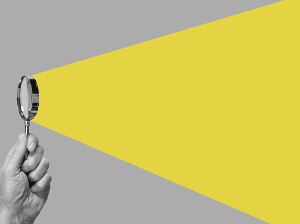
Data discovery deals with extracting useful information from data and presenting it in a visual format that is easily understood. The types of useful information discovered during the process range from finding patterns in human behavior to gaining insights about data glitches to answering highly specific business questions. Using data taken from a variety of sources, data discovery allows organizations from essentially every industry to identify useful patterns, insights, and trends.
What Is Data Discovery?
Data discovery is a process, not a specific tool. The process of data discovery normally involves collecting data from several different sources, data preparation, the use of advanced analytics, software translating the data into visual presentations, and the finding and identification of certain patterns or themes.
The process of data discovery can be described as data analytics with a visual presentation layer thrown in for nontechnical users and upper management.
Software that supports visual presentations, typically by way of a dashboard, are becoming extremely popular. The use of visual presentations takes advantage of the human brain’s ability to recognize patterns and digest visual information at a glance, as opposed to working with data tables spread out over multiple pages. The use of visual presentations is a remarkably useful tool during the data discovery process.
The visual presentations supported by data discovery have become an important trend for businesses as they strive to understand the potential of their data.
The challenges of data discovery include siloed data, a lack of data structure, disorganized data, and inefficient data searches. These issues can result in confusion, misinformation, and poor decision-making.
What Are the Benefits of Data Discovery?
The primary benefit of using data discovery is the recognition of information that improves the organization and increases profits. Other, more subtle benefits are:
- Users can quickly get answers to ad hoc questions
- The ability to customize solutions, rather than relying on traditional standardized solutions
- Easy access to visual “snapshots” for reuse and specific business situations
- It can be used to provide a form of Data Governance to business intelligence environments
Laws, such as the GDPR (General Data Protection Regulation) and the CPRA (California Privacy Rights Act), require the personal information collected on individuals be updated, deleted, or altered upon their request.
Data discovery can be used to locate an individual’s personal information, allowing the data to be altered, or to delete the data entirely. (The previous link leads to an article that also describes setting up a data discovery program.)
What Is the Difference Between Data Analytics and Data Discovery?
The data analytics process is part of the data discovery process, and the goals of the two processes are similar. Data analytics is the science of studying raw data to form strategies that optimize the organization’s performance and help to maximize profits. It requires several sophisticated data analysis skills, including data modeling and guided analytics.
Data discovery is essentially data analytics that uses specialized software to provide nontechnical users and upper management with a visual model that supports quickly understanding and absorbing key trends and insights.
What Are the Key Components of Data Discovery?
Data discovery helps organizations turn large amounts of data into useful insight without the need for a deep understanding of information technology. Modern data discovery does not require extensive or complicated models. It can be reduced to three basic components, which are listed below.
- Data collection, preparation, and integration: Data from a variety of sources is gathered and prepared for use. This includes integrating the collected data.
- Guided advanced analytics: This combines descriptions with visuals to present a complete picture of the organization’s company’s data. Modern data analytics have evolved into a self-service, cloud-based process that is supported by machine learning and artificial intelligence.
- Visual presentations: Modern data analytics (with AI and ML) supports the use of dashboards/visual images with charts, diagrams, and other forms of media. (Data groups can be manipulated while viewing the dashboard.)
Visual Presentations of Data
The data discovery software supporting Interactive and visual presentations allows decision-makers to quickly understand major trends and internal problems. The use of visual analysis has become an important cutting-edge tool for decision-makers to act on data. Additionally, data discovery software can be used to provide statistical information in a visual format, supporting a more sophisticated data analysis by the humans.
Visual presentations allow large and complicated datasets to be transformed into a visual format, in turn making the data easier to interpret and understand. It allows humans to view data in a more comprehensible and accessible way. Charts, graphs, and other types of visual formats can reveal patterns that might not be noticeable in a raw, numerical format.
The ability to identify and understand patterns through the use of data discovery can result in humans making faster, more trustworthy decisions.
Skills
Data discovery requires an understanding of data relationships and data modeling, as well as sophisticated data analysis skills. The goal is to maximize the value of the data to improve decision-making and to optimize the organization’s processes and help in developing new business models. Other important data discovery skills are listed below.
- Pivoting: Data pivoting allows humans to rearrange the rows and columns in a report so the data can be viewed from different perspectives.
- De-identification: This deals with laws regarding personal privacy. It is the process of removing or hiding personally identifiable information (PII). It reduces the risk of individuals being identified and connected with data.
- Data tracing: The tracking of data flows and transformations as it moves across systems and applications. The process shows how the data moves through a business’s infrastructure.
- Data transformation techniques: These techniques include data manipulation, normalization, generalization, attribute construction, discretization, smoothing, and aggregation can help to resolve various problems.
- Data Quality analysis techniques: This involves testing and improving the quality, or accuracy, of the data. Software tools are normally involved, and a familiarity of those tools can be quite useful.
What Are the Challenges of Data Discovery?
Gathering data of high quality – data that can be trusted – is the first challenge. This data can come from a variety of free sources or be purchased. If data is being gathered from outside sources, selecting reliable data sources is the simplest solution. Government and university sources are often reliable, as accurate information is a high priority.
Purchasing bulk data is a little riskier, because the businesses selling the data often prioritize profits over accuracy. (Manually double-checking small amounts of the data for accuracy by comparing it to alternative sources can be done, but because of the volume of data that has been purchased, double-checking it all is unrealistic.)
When the data that has been collected isn’t relevant or is an overwhelming amount, it is referred to as “inefficient information access.” It is important to know the specific parameters of the research to minimize the collection of unnecessary data. For data collected from free sources, this may involve narrowing the data discovery search queries. For purchased data, the specifications have to be planned, and limited, in advance. (No free re-does.)
Gathering and using high quality data from inhouse resources relies on staff developing the habits that support high quality data.
Different departments within a business sometimes save their data in separate storage systems, typically referred to as data silos.
Data silos are inhouse repositories that represent missing information. They are typically controlled by an individual department that has isolated its data from the rest of the organization, treating it as “their” data. Siloed data is normally stored in a standalone storage system and is sometimes incompatible with the data normally used by the business.
On the surface, data silos may seem to be harmless, but this siloed data prevents accurate data analytics, data discovery, and organizational collaboration across departments.
A lack of data organization can also cause problems in the data discovery process. Data content that has not been organized into clearly divided categories can be difficult to navigate. Additionally, documents coming from multiple sources, and in multiple languages, only worsen the difficulties of being able to locate data when needed. Organizing the data streamlines the data discovery process.
What Are Data Discovery Tools?
There are a number of software solutions available that support one, or all, of the data discovery process. When researching data discovery tools, make sure the tools have the characteristics listed below.
- Supports visual representations of data
- Provides a code-free environment for the data discovery process
- Supports access to appropriate data sources (important)
- Provides data preparation and modeling capabilities
- Supports interactive navigation within visual presentations
Data discovery tools are used to find and understand the needed data quickly and efficiently. These tools can help in developing and refining models, and analyzing structured and unstructured data. Normally, data must undergo several transformations before it can be understood and interpreted. The use of data discovery allows researchers to discover insights from the data without being experts in the fields of data preparation and analytics. Some of the tools currently available are listed below.
- The organization OpenDataDiscovery offers an open source data discovery platform.
- Xoserve offers a data discovery platform, but my somewhat limited research suggests access is complicated by the association of at least two other organizations. Working with them may be complicated.
- Atlan has published a fairly good list of data discovery tools – with dashboards.
What Is the Future of Data Discovery?
Early versions of data discovery (from the 1990s) were based on data mining with lots and lots of manual labor. Modern data discovery tools are still in the early stages of development, but advancing quickly. The use of these tools, primarily because of the visual presentations, has increased significantly in the last few years, in turn promoting a large shift in the use of data discovery to develop business intelligence.
In the near future, data discovery will become even easier to use. As artificial intelligence advances, and large language models are applied to data discovery, we can expect the data discovery process to become both quick and efficient. (However, the use of poor-quality data will remain an issue.) This should lead to more discoveries being made – in medicine, cosmology, business – and new insights.