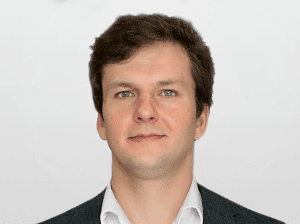
In the face of rising costs and rapid technological advancements, businesses must streamline their operations to remain competitive. An often overlooked area in these cost-cutting initiatives is data management. While many companies focus on reducing expenses related to infrastructure – such as virtual machines and serverless architectures – data management costs frequently go unchecked until they become unmanageable. This oversight can lead to significant financial inefficiencies that undermine overall cost-saving efforts.
One reason is that data teams are typically evaluated on their revenue contributions and the new capabilities they introduce rather than their efficiency in managing costs. This perspective has led to the emergence of “modern legacy” systems: technologically advanced yet operationally burdensome and costly structures that fail to efficiently meet current needs.
Strategic Data Management for Long-Term Success
To tackle this issue, a fundamental strategy for reducing data management costs is to ensure systems are designed to meet actual usage requirements rather than hypothetical future needs. This approach avoids overprovisioning and encourages periodic reassessment of technology and capacity based on real usage patterns.
To implement this strategy practically, businesses must first assess their actual needs. Evaluating the specific data needs of different business units helps align data architecture with these requirements. For example, some teams may require real-time data, while others might only need daily or weekly updates. Avoiding overengineering is crucial; companies should resist the temptation to implement advanced capabilities, such as real-time data streaming or machine learning unless they are fully prepared to utilize them.
Additionally, optimizing expensive or frequently run queries can significantly reduce costs without affecting overall system performance. A practical step is regularly reviewing query logs to identify costly or inefficient queries. Optimizing these queries by refining their structure, such as filtering specific data sets or improving join conditions, can lead to substantial savings. Where feasible, replacing real-time calculations with batch processing can reduce computational costs. For instance, instead of generating customer predictions on the fly, periodically pre-calculating these insights can decrease computational expenses.
Emerging AI copilots can help. These tools assist end-users, who typically lack expertise in data queries and optimizations, in constructing optimized and cost-efficient queries. They can also identify queries likely to incur substantial costs, a task previously manageable by data engineers. This capability makes cost-effective data management more accessible and affordable for a broader range of users.
Exploring new technologies like hardware accelerators such as GPUs or FPGAs can also help reduce data processing costs. These accelerators handle large-scale computations more efficiently than traditional CPUs, cutting processing time and expenses. Furthermore, periodically reassessing the technology stack to incorporate newer, more cost-effective tools and technologies helps avoid the pitfalls of relying on outdated or overly expensive solutions.
Moreover, evaluating and optimizing licensing strategies for business intelligence (BI) tools and other software can lead to substantial savings. Regularly reviewing license usage to ensure it aligns with actual needs and adjusting allocations based on user activity can help. Open-source alternatives, when feasible, offer similar functionality at a lower cost, which is particularly beneficial for large user bases or organizations with significant scaling needs.
Data virtualization and semantic layer technologies are also gaining popularity. They offer a practical way to connect and integrate data across organizational silos, enabling faster data and analytics use cases without the cost and effort of building centralized platforms like data warehouses, lakes, or lakehouses.
Adopting a lean data management strategy involves breaking down large data warehouses into smaller, domain-specific systems. This enhances manageability and performance, aligns with specific business needs, and reduces unnecessary expenses. By tailoring smaller systems to specific business domains, companies can improve performance and cut costs associated with maintaining large, monolithic structures. Regular audits of data systems ensure they remain efficient and effective.
Overcoming Challenges
Organizations face several systemic challenges in optimizing data management costs. A primary hurdle is the lack of a mandate for data teams to focus on cost optimization. Unlike other IT areas where cost efficiency is routinely measured, data management has historically avoided such scrutiny. This oversight often leads to the accumulation of massive, underutilized data sets, driven by a fear of losing potential insights – “sunk cost anxiety.”
To address this issue, regular evaluation of data usage and utility is crucial. Challenging long-standing assumptions and making tough decisions to discard or redesign underutilized or outdated data storage practices can help. Implementing a tiered data storage system, categorizing data into high-, mid-, and low-frequency access tiers, can significantly reduce costs and improve system responsiveness.
Internal capabilities and external expertise are also necessary. Thorough maturity assessments help determine whether current teams possess the skills needed for essential changes. Engaging external consultants can provide specialized knowledge and perspective for modernizing and optimizing data architectures.
Conclusion
Addressing overlooked aspects of data management can help businesses discover new avenues for being more efficient and reducing costs. Transitioning from merely managing data to strategically utilizing it for cost efficiency and improved performance is essential in today’s competitive landscape. Implementing fit-for-purpose designs, optimizing queries, adopting new technologies, refining licensing strategies, and employing lean data management principles can lead to substantial cost savings and improved data utility. Regular audits and critical assessments will ensure data management practices align with evolving business needs, positioning companies for long-term success.