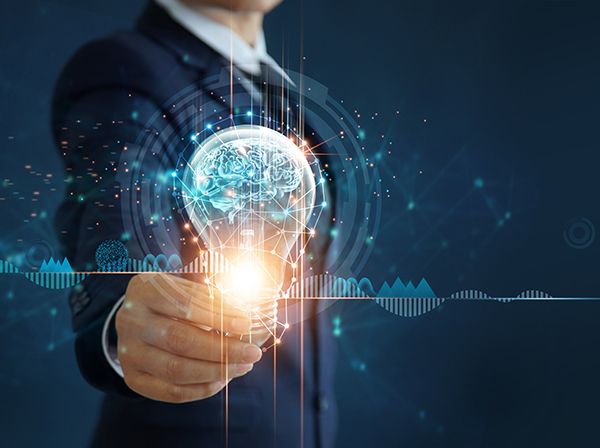
Without a clear understanding of core data concepts, communications around implementing an organizational Data Management initiative can become a muddle. As different teams come together to plan and organize data activities, they must integrate what they mean about data with any technologies.
For example, take the term “Data Governance.” Data engineers building systems and tools to enable data transport probably see Data Governance as an application for delivering data sets to those with access or to clean data. But marketing, sales, and other businesspeople see Data Governance as making customer information more findable and data usable for a campaign.
Both views align with Data Governance as an “organizing framework that pulls these types of discussions and capabilities together for a better decision-making process and supporting capabilities, aligning an organization’s data to execute their business goals,” said Kelle O’Neal, founder and CEO of First San Francisco Partners. However, without everyone on the same page about what Data Governance means, the initiative built for marketing and sales can fall short, or the processes to understand customers seem like a waste of time to IT.
To get everyone in the organization aligned about data concepts before discussing Data Management implementations, O’Neal presented coherent definitions at DATAVERSITY®’s Enterprise Data World (EDW) educational conference and explained the significance of these meanings. Because “digital transformation” – identifying business operations that can benefit from automation and enacting these improvements – emerges front and center for most companies, this article will focus on the definitions that make up its core constructs.
Data Architecture Concepts
As organizations focus on digital transformations, discussions about Data Architecture and how to connect business needs with enterprise data will arise. For example, businesspeople will talk about how to store and compute data or who should take responsibility for different data sets.
In these cases, prominent Data Architecture terms often arise; here are explanations by Kelle O’Neal:
- Data lake: A data lake holds vast volumes of structured and unstructured data. O’Neal added, “Data lakes allow for data processing in one location. They have revolutionized capabilities in accommodating larger data sets.”
- Data lakehouse: She describes the data lakehouse as the best qualities in a data lake and data warehouse. It takes “advantage of some schema and structure provided by the data warehouse with the option of having a tremendous amount of unstructured data, as available in a data lake.” The magic happens in a lakehouse when some schema associated with the structured data applies to unstructured data as users consume or read it.
- Data mesh: A data mesh combines distributed and interoperable technologies around Data Governance and curation methodologies to improve organizational flexibility. Consider a data mesh encompassing four principles and supporting a domain-centric business model, a decentralized and modular data architecture.
- Data as a product: The idea of data as a product came about as a component of data mesh. O’Neal noted that where a data mesh is decentralized, this characteristic leaves accountability to the entity producing the data and the teams focusing on that data’s distribution and usage. So, “data as a product spans the entire process rather than just a sub-set of data activities.”
- Data fabric: O’Neal thinks of data fabric as “integration on steroids” and a “technical overlay to operate a data mesh more effectively.” The data fabric “connects all the components to enable easy access to the data,” she said.
- Data gravity: “Data gravity corresponds to an architectural concept for organizing massive data stores and applications to more efficient integration with these data stores.” This idea comes in handy when discussing “workload performance issues, impacting storage locations systems and applications,” O’Neal observed.
- Data-driven: Data-driven defines the architectural and technical solutions an organization needs to implement for data to inform decision-making and operational processes. “Organizations can quantify business benefits from data-driven behaviors,” said O’Neal.
Data Quality Concepts
Data Quality – “the overall practice of defining expectations for data, monitoring it, and correcting for non-conformance” – makes or breaks digital transformation. As O’Neal stated, Data Quality is an umbrella over several related concepts.
Key components under Data Quality include:
- Data observability: Data observability means an approach to Data Quality focusing on monitoring data flow. Typically, a system is set up for observability and peers into the “different steps along data movement and reports any anomalies and corrective actions as well,” O’Neal said.
- Data prep: She defines data prep as a step in Data Quality to “profile, clean, validate, enrich, and transform data. These activities make it ready for the next step in the larger data preparation process.” Data prep runs more smoothly with good governance and data standards.
- Data wrangling: “Data wrangling occurs after data prep and ensures a correct structure for the analytics use case,” O’Neal said. Data wrangling makes up a Data Quality process like data prep and observability.
- Synthetic data: Data sets with artificially generated values used in development or testing make up synthetic data. That way, development and testing teams can see if an application behaves and transforms data, resulting in good Data Quality. At the same time, real-world data remains unchanged, protected, and consistent.
- Bad Data Quality: Discussions across organizations sometimes focus on the perils of having poor Data Quality. Several data concepts come into play in these conversations:
- Data debt: O’Neal said, “Data debt grows from bad decisions around managing data.” Organizations need to efficiently and effectively clean data, execute Data Quality practices and standards, and manage metadata to avoid data debt. The greater the debt, the more problems to address. Data trash: Businesses continuously keep collecting, storing, and supporting the data they ingest, which comprises data trash. O’Neal noted that data trash happens freely with limitless storage capability, where the company can spin up additional cloud instances. However, problems may arise in ensuring compliant data trash usage and protections with data regulations. Dark data: Dark data comprises the existing data that nobody has considered or used. She stated that dark data may have value or become data trash. It is unknown because it just sits in storage.
Data Enablement Concepts
Many organizations want to give their workers and customers the access they need to do business well, which is the essence of data enablement. This term, essential to digital transformation, “empowers and supports businesspeople to leverage data for their outcomes,” O’Neal observed. Data enablement forms a Data Governance objective, spills into many other organizational services, and contains related ideas, such as:
- Data literacy: According to O’Neal, data literacy describes processes around assessing people’s capabilities and how well they understand data. She said, “Data literacy is a way to pull together training, communication, governance, metadata, Data Quality, and other services to support data users.” The advantage of calling out data literacy when this idea has existed before, communicates some “specialization and best practices around it.”
- Data democratization: A data democracy is the philosophy that everyone in the organization, regardless of job title, should be able to work comfortably with and feel confident about using data. O’Neal said that the idea that anyone can “identify and source the data as they need to do their job” centers around data democracy.
- Organizational change management: Organizational change management includes Data Management and all other business activities and processes. O’Neal described it as an “overarching capability and the discipline of driving business results by changing behaviors.” This kind of evolution is situational. Additionally, organizational change management guides companies on the steps to take.
- Data-informed: Someone who is data-informed uses data when evaluating decisions. O’Neal noted that that an individual may make a gut decision, even after assessing the data.
- Data-centric: Data-centric individuals put data first. O’Neal explained that data-centric represents the ultimate objective for an organization’s workers, where data-informed is the starting point.
- DataOps: DataOps defines a “group of collaborative support functions in Data Management,” stated O’Neal. DataOps provides an “operational understanding from a business perspective with assistance from technology to ensure sustainability so that data remains available and is used appropriately.”
Conclusion
Data Management implementations carry different meanings across the organization, depending on the role and activity with that data. Businesspeople and technical people have different ideas, even when using the same terminology.
So, organizations need to ensure alignment and a consistent understanding of data concepts. This shared knowledge comes in handy when discussing Data Architecture, Data Quality, and data enablement, fundamental data transformation components. O’Neal spent an EDW session gathering and clarifying the underlying data concepts to help make discussions more straightforward. See the bingo sheet to review and refer to the terminology in this article.
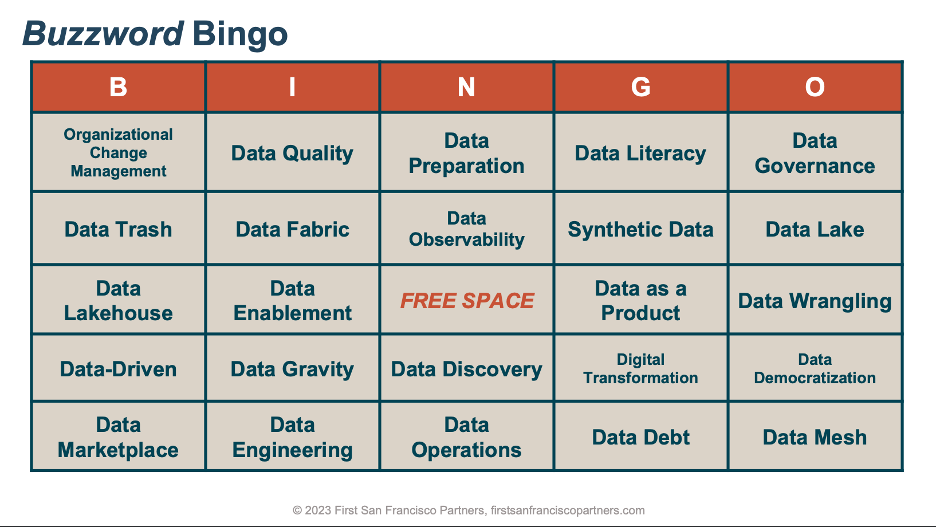
Want to learn more about DATAVERSITY’s upcoming events? Check out our current lineup of online and face-to-face conferences here.

Here is the video of the Enterprise Data World presentation:
Image at top used under license from Shutterstock.com