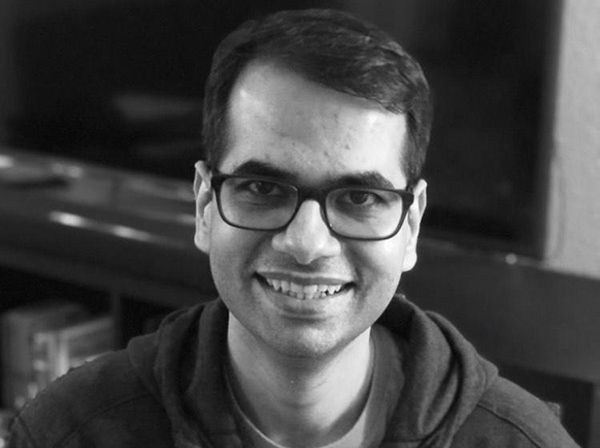
As the economic environment becomes more somber, companies are focusing on achieving profitable growth and not just growth. Data-driven decisions can accelerate this goal, but organizations must develop new data muscles to get them there.
The last few months have been a whirlwind for the business world: slowing growth, bleaker capital markets, layoffs, the possibility of a recession, reduced investor risk appetite, and an overall uncertain economic climate. These have forced companies to rethink their strategies and course-correct their paths. One of the biggest corrections has been to balance growth with profitability. Although this has been most prominent in high-growth tech companies, the implications are broad.
As new realities emerge, the mindset must evolve, and new data muscles must be developed. The frenzied pace of growth in the last decade meant that the primary focus of data analytics was in the areas such as demand generation, upsell cross-sell models, growth marketing, etc. While these will stay important, maybe even more so, new data analytics muscles must be built to complement existing ones – muscles that help drive efficiency and maximize return on investment. Many things were not areas of focus for a long time but now need to be. Specifically, there are five areas that companies would do well to focus on now:
- Lean technology and operations models to weed out inefficiencies: There are several areas where inefficient models have become commonplace. A prime example is the technology model of having multiple software and vendors do very similar things or do things that are not needed. In the data world, it has manifested in the forms of a company having multiple “data platforms,” data warehousing technologies, BI tools, etc. The genesis was that teams had funding to buy and deploy what they felt was the most convenient technology for them. And this has left organizations with multiple stacks that don’t interoperate. Similar themes exist on the resourcing side, where, for example, data engineers and analysts across teams are doing overlapping work. And companies are ending up with multiple versions of “similar but different” data products that then cause confusion. A hard look is needed at what rationalized tech stack and resource deployment optimizes at the company level and not the team level.
- Optimization focus to complement the maximization focus. A cultural change of valuing and celebrating optimization wins is necessary to sharpen this focus. An improved process, a workflow automated, a margin leakage identified, all need to be celebrated just as much as a new customer acquisition. Topline growth is paramount but so is the bottom line today. This means pricing models need to be tighter to ensure revenue generation is profitable, forecasting models need to be more accurate to plan better for operational expenses, etc. Overall, data teams need to develop new skill sets and focus on newer algorithms, e.g., churn vs. demand gen and executives need to have more targeted asks of the data teams.
- Capturing small signals through analytics to identify opportunities. When capital is cheap it makes sense to focus on the largest opportunities and invest behind them. This now needs to evolve to not pursue some bottom-line dilutive opportunities but to identify several smaller opportunities that add up to be greater than the sum of parts. Multivariate machine learning techniques can help identify what humans can miss. An example of this in media would be to not just focus on making deals that sell newer content, but also identify which of the old content will resonate with the buyer’s audience and sell it as a package. Tactically, for example, sales recommendation engines would need to be updated to include older content and not just new.
- Bringing data-driven decisions and outcomes to centerstage. One of the silver linings of the new economic environment is that executives have become more intentional. This provides data teams a great platform to add value by using data to generate insights and then drive to outcomes. Companies that bring the data and business teams together and can devise an effective human-AI interface will have a decided edge against those who do not. One of the most powerful tools for this are meeting rituals, e.g., executives can start all meetings with a metrics review and ask teams to present metrics for any decisions they want supported.
- Finally, a critical muscle needed is comfort with investment in data fundamentals. For several years surface-level analytics and reporting have sufficed in the “rising wave lifts all” environment. Most companies have not invested in fundamental capabilities such as streamlined data tech stacks, data governance, and setting up the right organizational models for data teams. This needs to change to take fragility out of the system and make data a robust differentiator for profitable growth.
Organizational data muscles are much like human muscles: The ones that are not exercised become weak. And a new environment requires new ones to win – akin to a sprinter needing a different training regimen to become a swimmer. The winners over the next few years will be the companies that quickly pivot to doing things in newer ways as they march on their journey to profitable growth.
Views expressed in this blog post are personal and do not reflect the views of the author’s employer.