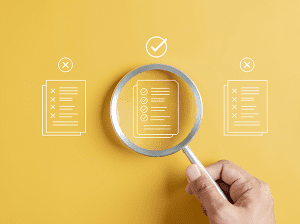
Data-driven organizations are in a race to collect the information that modern analytics techniques rely on to generate insights and guide business decisions. The ever-growing flow of data into business systems challenges companies to devise new techniques for ensuring the quality of the data as its quantity skyrockets. Data quality tool vendors are rising to the challenge by enhancing their products so they accommodate innovative data collection and analytics techniques. Within the broad category of data quality are software tools used for data testing, data discovery, data observability, and other measures. The variety of data systems, data applications, and data management approaches complicates the process of choosing the best data quality tool for your company’s needs.
Once you’ve defined your data quality requirements, you’re ready to start evaluating the tools you’ll use to achieve the optimum level of data quality. Options include commercial and open-source products designed for data testing, data discovery, data observability, and other data quality measures.
The Six Dimensions of Data Quality
Data quality describes the ability of a set of data to meet six dimensions of reliability: accuracy, completeness, consistency, timeliness, validity, and uniqueness. In addition, it ensures that the data is fit for its intended purposes.
- Accuracy is measured by how well the information represents the objects and events it describes. Data that is accurate today may not be accurate tomorrow, however, because the conditions the data relates to are constantly changing.
- Completeness determines whether all pertinent aspects of the data are present and identifies missing elements. Analytics performed on incomplete data is likely to be biased or fail to address all required areas.
- Consistency ensures that the data is uniform and coherent wherever it appears in the system, including databases, applications, and information systems. Data consistency confirms that the data maintains its state and aligns with business rules throughout its lifecycle.
- Timeliness establishes that the data represents reality at the relevant point in time. In data processing, timeliness relates to availability when needed. It is affected by data lag (the period between an update and its availability), information float (the time between a fact’s discovery and use), and volatility (the likelihood of the data changing over time).
- Validity measures how well the data conforms to the standards, rules, and constraints set by the business to ensure that its use will lead to trustworthy insights that meet the needs of the intended application.
- Uniqueness confirms that the attributes and traits of each data element appear only once in systems. This makes processing more accurate and efficient by removing duplicate instances, which is especially important for data being used to train machine learning models.
Implementing a data quality plan entails seven steps:
- Extract the required data to perform the task from internal and external sources.
- Evaluate the data to ensure it meets all task requirements and is relevant to accomplishing the task.
- Assess the quality of the data using various data quality management techniques.
- Clean and enrich the data after identifying any issues from the quality assessment using such error-correcting methods as type casting, outlier capping, and missing value treatment.
- Report the findings of the quality assessment, cleaning, and enrichment to document the data quality metrics.
- Remediate all problems identified and take steps to prevent them from recurring.
- Review and monitor the firm’s data quality management practices and anticipate any gaps or shortcomings that may arise in the future.
The Relationship Between Data Quality and Data Observability
Data quality describes a characteristic or attribute of the data itself, but equally important for achieving and maintaining the quality of data is the ability to monitor and troubleshoot the systems and processes that affect data quality. Data observability is most important in complex, distributed data systems such as data lakes, data warehouses, and cloud data platforms. It allows companies to monitor and respond in real time to problems related to data flows and the data elements themselves.
Data observability tools provide visibility into data as it traverses a network by tracking data lineages, dependencies, and transformations. The products send alerts when anomalies are detected, and apply metadata about data sources, schemas, and other attributes to provide a clearer understanding and more efficient management of data resources.
Aspects of data observability include drift detection, which maintains consistency of data in machine learning models and pipelines, and root cause analysis, which identifies the source of data-quality problems and assists with their resolution.
Data Quality Tool Buyer’s Guide: Features to Consider
A company’s data quality efforts are designed to achieve three core goals:
- Promote collaboration between IT and business departments
- Allow IT staff to manage and troubleshoot all data pipelines and data systems, whether they’re completely internal or extend outside the organization
- Help business managers manipulate the data in support of their work toward achieving business goals
Software designed to monitor and promote data quality fall into two broad categories: source-level tools and downstream tools:
- Source-level products check the quality of data to confirm that the data meets all quality requirements and is well understood. The quality checks are performed at the source and each time the data is transformed or relocated to ensure the data remains accessible and problem-free.
- Downstream products are used to verify data quality that can’t be checked at the source or that is altered during transformation. They include data cleansing tools and master data management products that make sure an organization’s shared data is accurate and consistent.
A comprehensive data quality toolkit covers eight specific functions:
- Contextual workflow management. Potential data quality anomalies are reported to the stakeholders with responsibility for maintaining the data assets involved. Directing the report to the appropriate parties requires understanding the context of the data.
- Data quality dimensions. The tools facilitate compliance with the data quality dimensions described above by helping stakeholders understand how the dimensions affect their data-driven decision-making processes.
- Collaborative workflows. Fostering collaboration between technical and non-technical stakeholders who share a data pipeline makes it easier to anticipate data quality problems and respond quickly and effectively. Workflow management tools must overlap departments, roles, and responsibilities that extend throughout and beyond the organization.
- Proactive alerting. Data operations teams require tools that allow them to implement a system of alerts that let appropriate parties know promptly when an issue is detected. Fast responses prevent a small, localized problem from propagating throughout the company’s data operations.
- Investigative tools. When alerted to a potential problem, data operations teams need visibility into data pipelines, data lineages, and other processes to track down the source of the anomaly, determine its cause, and fix the problem.
- Data profiling. This technique verifies the data’s characteristics and confirms that it complies with statistical standards and the company’s own business rules. Three types of data profiling are structure discovery, content discovery, and relationship discovery.
- Anomaly detection. The products apply machine learning and other AI techniques to scan data proactively to look for and address glitches in data operations automatically. Any patterns in the data that don’t conform to what the models expect based on historical data are tagged as outliers.
- Data cleansing. Once a problem has been identified in the data, data quality tools provide mechanisms for returning the data to a pristine state in preparation for its use in business applications. Steps in data cleansing, or cleaning, include exploration, filtering, regular expression (RegEx) and string manipulation, date and time parsing, merging and joining, transformations and data-type conversions, deduplication, sparse data, normalization, and standardization.
How Data Quality Tools Promote Your Business’s Trustworthiness
Data quality is a core component of all four trends identified by Gartner as having the greatest impact on data and analytics in 2024.
- Business investments in AI increase the value of the data that powers AI systems, so protecting the quality of the data becomes more important.
- Data systems continue to grow in size and complexity, which makes it imperative to pinpoint and react quickly to potential compromises in data quality.
- The people within your organization are most effective when they trust the data that underlies their management, planning, and decision-making. As partnerships with other businesses become more common, the need to trust the quality of your company’s data extends beyond your premises.
- The boom in AI use by businesses will require training workers in how to apply new AI-driven products and processes so they enhance their work lives and make their daily routines more efficient. The data serving as the basis for that training will need to be vetted for quality to ensure that the technologies deliver the benefits they promise.
Perhaps the greatest return businesses will realize from their investment in data quality tools is measured by how well their customers are being served. High-quality data helps keep businesses in touch with their customers and in tune with changing market conditions and consumer preferences. A company’s investment in data quality tools is ultimately an investment in its employees and clientele.