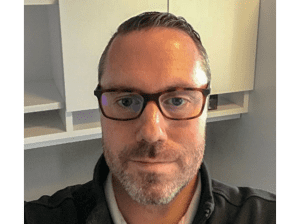
CXOs this year have witnessed a rollercoaster economy amid plenty of turbulent events – from ongoing inflation affecting consumer spending to large stock market swings, major overseas conflicts, and the uncertainties of an election year. Not surprisingly, the economic forecast remains murky at best. According to a CNBC CFO survey, CFOs seem to agree that inflation will remain elevated into 2026 and 54% say the economy is either in a recession or will enter one over the next year.
Corporate leaders have been managing their workforces and business strategies during times of significant upheaval in recent years, starting with the COVID-19 pandemic. The problems that have been simmering beneath the surface for years – such as unsustainable costs in key consumer sectors like healthcare – are now becoming too hard to ignore.
Yet here’s the glitch: Nobody wants to be left behind in the latest wave of technological disruption. Chief officers are looking at AI with a hard eye. They want to leverage AI magic soon for both operational efficiency and competitive advantage. They know that AI will eventually require notable investment across people and technology.
If data is the fuel to power AI, IT leaders must ensure the organization’s data is in the best shape possible. This requires that IT teams clean up the data mess and get control of the unstructured data that is fueling AI and ML. A looming barrier is that today’s data estate incurs high costs to manage, impeding the budget for AI.
Let’s discuss why this is so:
- Unstructured data, which makes up at least 90% of all data being generated in the world, is heavy and hard to manage. This data can be both big and small, including user documents, emails, text and chats, images, audio, video, sensor data, instrument data – anything not stored in rows and columns in a database. Nearly half of organizations are storing more than 5PB of unstructured data and nearly 30% have more than 10PB, according to my company’s recent State of Unstructured Data Management report. The survey, in its fourth year running, has consistently uncovered that most organizations are spending 30% or more of their IT budget on data storage. In a large enterprise, this could be millions of dollars annually. And with data growing so quickly – from roughly 60 zettabytes (ZB) in 2020 to an estimated 180 ZB in 2025 – these costs will keep rising unheeded.
- Data hoarding contributes to the problem. It’s more common to keep all the data year over year than to institute data deletion, or even data archiving policies. Enterprises often retain unstructured data for decades because it contains useful information such as customer insights, potential research intelligence, and machine learning training data for the future. Audits and compliance requirements also support the long-term or never-ending storage of data. Yet with today’s data growth rates, it’s no longer sustainable economically or from an energy standpoint to keep data forever.
- Data storage is only 25-30% of the total cost. Most of the expense of storing unstructured data lives beyond primary storage. IT teams need to protect data with backups and replicate it for disaster recovery and that means creating multiple copies of data to store, manage and secure. Yet most data is not mission critical or active and can be archived at a lower cost and without making multiple copies.
- One-size-fits-all storage: The previous point leads to this one; organizations too often store all or most of their data on expensive network attached storage (NAS) technologies when most of it doesn’t need that level of performance and availability. Years ago, before the Internet and mobile phones had taken off, this wasn’t a problem. Enterprises didn’t have enough data to worry about where it was stored. Today, though, there are many classes of data storage on-premises and in the cloud that help organizations store data in the right tier at the right time to save money. Placing “cold data” that hasn’t been accessed in a year or more to lower cost, secondary storage can save anywhere from 60 to 80% a year on annual data storage, backup and DR costs.
- Lack of visibility: The ability to save on data storage and implement the cost-effective strategies outlined above is impossible without insights on the data such as its rate of growth, how much you have, storage costs, types and sizes of files, top owners, data usage trends and its value to the organization. This lack of visibility also brings data governance and compliance risk: You cannot protect your data if you don’t know what it is or where it lives. Getting this information is possible with an independent, unstructured data management solution that can gather metrics across all storage.
If you can get metrics on your data from the data center to the cloud, create data management policies for different data sets, and automatically move stale or cold data to lower-cost archival storage such as in the cloud, the savings can be astounding. On a 4PB NAS environment with a 30% year-over-year growth rate, your enterprise could save over $2.6 million or more annually with the right cold data tiering and/or archiving strategy alone.
Unstructured Data Cost Savings Decision Tree
Here are three ways to model potential cost savings from unstructured data management:
Cold data potential savings
- How much data is rarely-used or cold?
- How much you can save by archiving it, based on your own cost model?
Orphaned data potential savings
- How much data is orphaned such as from ex-employees?
- How much is this costing us today?
- How can we save either through archiving or staged deletion?
- This also improves security posture and reduces risk.
Duplicates potential savings
- How much data might be potentially duplicates?
- Should we have a process to work with data owners to reduce these?
Unstructured data has been piling up unnoticed for years in data centers. Today, enterprises finally can classify, organize, and move it to affordable AI and ML tools where it can generate new value. But first, business leaders need to understand its costs and risks, and how to reduce both with the right data management strategy.