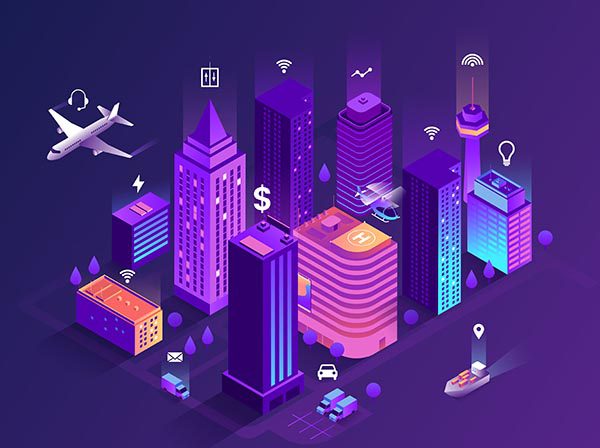
If a picture is worth a thousand words, but still missing valuable location data, then why not use artificial intelligence (AI) and machine learning (ML) to fill in the gaps? Take the image of Perth, Australia, below:
This graphic below shows vast data sets containing buildings, green spaces, roads to travel, and parking lots. Drill down even further and see rooftops, solar panels, fire hydrants, gas lines, and many other objects. And all this data constantly changes over time. City residents move, purchase new developments for their homes, drive roads with new potholes, and build new construction.
How is a person to keep up with it all? In a recent DATAVERSITY® interview, Zach Barney, a Senior Director of Sales at Nearmap, discussed about how machine learning and AI technologies drive advancements in location intelligence, thereby reducing business costs and expanding opportunities.
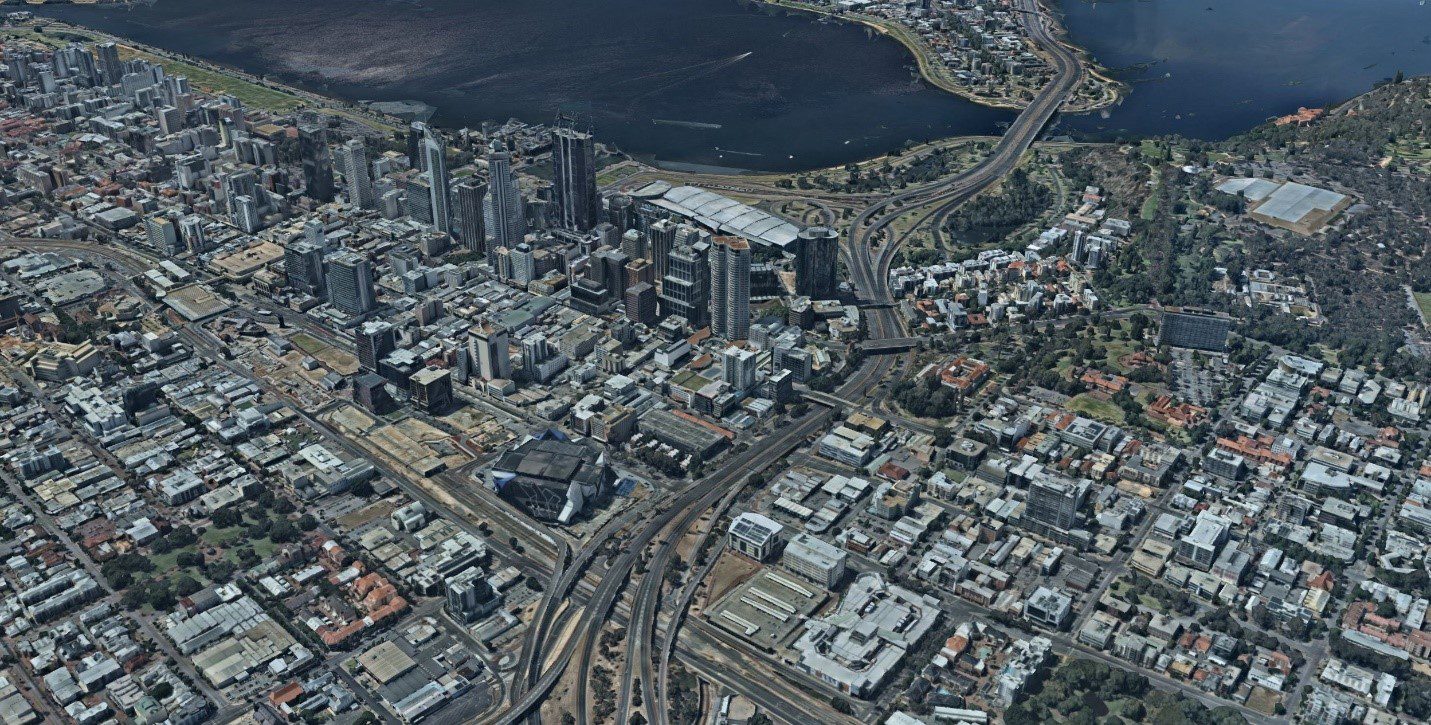
Image credit: Nearmap)
Barney explained that AI-driven location intelligence “gets rid of the manual process to identify important information.” Artificial intelligence provides the engine that makes it possible for machines to learn from experience by adjusting to new inputs and performing human-like tasks.
For example, consider home inspection, which usually requires an extensive site visit. The inspector will glance up at the roof and estimate if it is good or needs to be repaired. AI studies these kinds of physical tasks and asks what machines can do virtually, i.e., through a manual photo. Barney added, “Rather than having to manually go through and look at the imagery to identify whether a roof is damaged, artificial intelligence, in theory, can identify that change, off-site.”
Machine learning, a subset of artificial intelligence, uses algorithms to parse data, learn from those sets and decide about something. In the case of AI-driven location intelligence, ML can search through billions of data sets from aerial photos taken now, several months ago, a year ago, and 10 years ago. Machine learning identifies patterns from these vast data sets, labeling all the roof tops in an area, such as Perth. What is the benefit? Barney clarified:
“Imagine a roofer who wants to see every roof that has had damage within the past year in five zip codes, say in Perth, Australia. The search engine goes through the hundreds of thousands of damaged roof top tags, identified by ML, and return the results. Another type of computer code puts the results in an easy-to-visualize format.”
The roofer user retrieves an image such as:
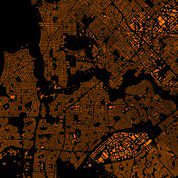
Image credit: Nearmap)
Barney added, “Rather than having to scrutinize the image and manually identify each roof one by one, ML did that task for the roofer.” The same idea could work in a home inspection.
How does AI-driven Location Intelligence work?
Barney explained AI-driven location intelligence processes:
- Get the Images: Capture aerial images that have the best data sets; good AI and ML require great data sets. To do this using aerial imagery requires an excellent process that is scalable. Historically, aerial imagery has been captured orthographically, which displays vertically top down. A firm like Nearmap needed a different approach, and found that oblique imagery works. Barney noted: “Oblique imagery uses a 45-degree angle, birds’ eye view, captured and published from all four cardinal directions, north, south, east, and west. This aspect provides abilities to see sides of buildings and measure the height of objects, etc.”
In other words, better detail can be gathered from an oblique image as compared to an orthographic image.
- Digitize the Image: One oblique image provides only limited information, especially where a location’s surroundings change over time. The oblique imagery needs to be stitched together, consolidating millions of aerial images over an extended time period, e.g., 10 years. Barney said:
“Think of it as a really, really highly detailed terrain map of an area, but more than just mountains, hills, rivers, and valleys. It also includes fire hydrants and cars and small trees. It captures that level of detail to show you the elevation of every single thing in the area.”
Barney thinks that such a model needs to be visually appealing to help the end user. See below for an example:
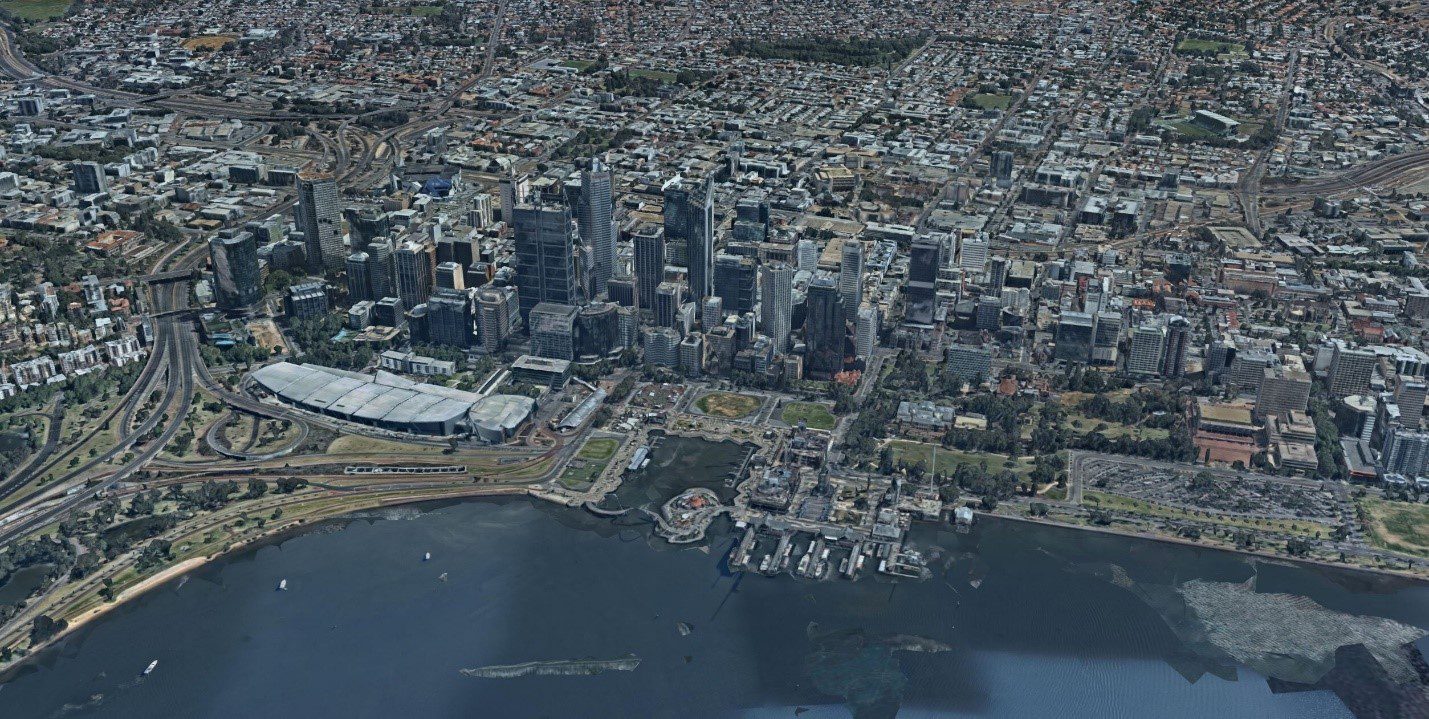
Image credit: Nearmap
- Use AI/ML to Analyze, Learn and Label Patterns: Machine learning steps into the digital landscape, identifying and tagging objects from the digital surface model. The high resolution allows software to detect all sorts of things on the ground and to see these changes over time and update results with information from new surveys. These abilities combine to form a library of searchable living datasets, automatically updated. Results are presented in an easy-to-see manner, thanks to the image digitization, so that users can measure changes and quantify attributes.
Developing such AI-driven location intelligence is massive and iterative. A system collects more aerial data, continuously digitizes it and labels more and more items. As Barney remarked:
“AI-driven location intelligence technologies have lots of different verticals. Cities, countries, or states provide reams of public-domain data. Residents of such communities want to know where certain facilities are located. They want to know details around their property boundaries, utilities, all of those things, garbage disposal routes for their truck. To get this information, citizens need to have accurate maps that line up with one another.”
When information from a lot of different sources is not correctly geo-located, then it provides misinformation which can lead to results that are, on the low end, annoying, and on the high end, catastrophic, for example, when a utility line is not mapped out properly, and a construction worker cuts into a utility line, causing a delivery disruption, or even worse, injuries or casualties.
Data accuracy and quality are essential. So, what are a few success stories where AI-driven location technology can be used with huge location data sets, scaled to the user while maintaining accuracy?
AI-driven Location Intelligence Case Studies
Nearmap uses its patented camera systems and AI/ML processing software to create a 3-D product accessible via the web. “The company takes AI-driven location intelligence technologies, making the information scalable and affordable,” Barney stated. The company’s applications promise to improve urban planning, architecture, and engineering. Three use cases show success in AI-driven location intelligence:
- Solar Paneling: Auric Energy hired Nearmap’s software as a service (SaaS) to speed up initial assessments and research properties for solar paneling. The traditional satellite imagery, e.g., from Google maps, was blurry and missed details. Auric Energy sales and analytic teams use Nearmap’s web portal to accurately measure coverage, identify roof obstacles, and craft proposals. Since Nearmap provides historical location data, the solar business could analyze shade to place panels for maximum sun exposure. As a result, change orders were reduced and more customers qualified for solar paneling.
- Public Safety: Shelby County is the largest county in Tennessee, by both population (927,644) and geographic area (785 mi). To dispatch fire, rescue, police and/or emergency medical, Shelby County provided each center a secure database, also known as ALI (Automatic Location Identification). Previously, the county relied on contracted flight to photograph the county. Not only was this costly but there were issues with image quality and timeliness. Nearmap integrated with Shelby County’s Geographic Information System, allowing dispatchers to stay on top of new developments, roads, and addresses. This allows emergency workers to be more responsive in arriving at and addressing a crisis.
- Site Development Management: HNTB Corporation, an employee-owner infrastructure solution looked to improve processes around architecture, construction, and engineering. This meant speeding up accuracy and efficiency in modeling projects’ sites. Using Nearmap technology, HNTB could easily identify terrain textures, curbs, sidewalks, and buildings. Team members could figure out measurements and get more precise estimates for clients. This resulted in better planning for when and where to move construction vehicles, a clearer picture on how a project will meet its timeline and a projected visual of the building or construction within the context of the area. In other words, more ground- work was figured out ahead of time without having to visit the site, and better engineering and construction decisions were made.
AI-driven Location Intelligence in the Future
Roofing, solar paneling, dispatching emergency services, and planning construction represent the tip of the iceberg in AI-driven location intelligence. Innovation will lead to more robust AI/ML algorithms, better 3-D models and more coverage of different locations. Dr. Michael Bewley, Director of AI systems at Nearmap, said, “This technology is going to profoundly change the way that cities are built. Our plans include delivering AI results in many forms, much the same way we deliver our imagery today.”
Image used under license from Shutterstock.com