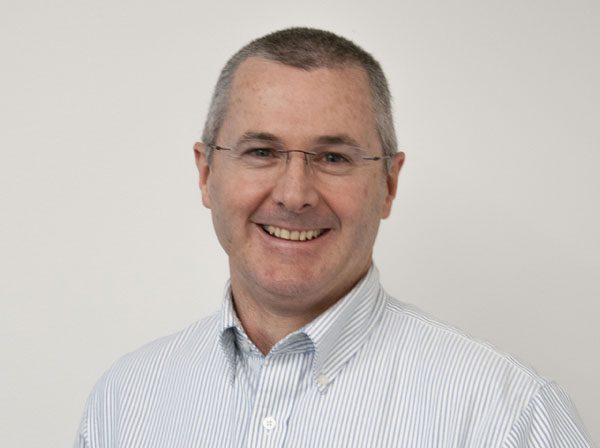
Click to learn more about author Craig Stewart.
In order to be successful in today’s ultra-competitive business environment, companies must be committed to data. Data needs to truly be at the heart of every decision an organization makes, but this is easier said than done.
There is an overwhelming amount of data in every organization. Data is constantly being produced — data about employee efficiency, data about customer usage, data from your applications, data from your software systems, data from your marketing initiatives, external market data, and more. Taken together, your company is producing tons of valuable data. This should be a good thing — it stands to reason, the more data you have, the better your decision-making process should be. But unfortunately, this isn’t the reality for a lot of companies. Just receiving and collecting data isn’t enough. You have to know what data you need and how to access, manage, and analyze it.
Most of all, you need to trust that your data is accurate and can be a solid foundation for important decisions. If it isn’t, then one decision based on bad data can create a snowball effect of bad decisions and actions, critically harming your organization.
The Data Trust Gap
In The State of Data Management — The Impact of Data Distrust, a recent study conducted by independent research firm Vanson Bourne, it was uncovered that data analytics is seen as very important for an overwhelming majority (82 percent) of organizations. Clearly, the importance of data to business success is well-known and well-understood. But knowing the importance of data doesn’t mean that the data your organization produces is data that can be trusted. In fact, that same report found that more than three-quarters (77 percent) of IT decision-makers don’t completely trust the data within their organization for timely and accurate decision-making.
It goes without saying that this is a very large percentage of decision-makers that don’t trust the data their own organizations are producing. The ramifications of this are staggering. In short, it means that (1) there is a massive waste of time, energy, and resources going on — there are mountains of data being produced that executives don’t want, need, or trust; (2) there are problems that need to be repaired with how data is being collected, managed, and analyzed so that executives can trust it again; and (3) decisions are either being made without the appropriate data to inform them, or decisions are still being made but based on bad, incomplete, or untrusted data.
The next logical question is why do so many decision-makers not trust their organization’s own data?
Thankfully, Vanson Bourne took a look at this question as well. Organizational distrust in data can be broken down into three main areas: (1) accessibility, (2) accuracy and timeliness, and (3) strategy and process.
They found that poor Data Quality and formatting, siloed data sources, and delayed analytics projects were the top reasons that decision-makers distrusted data. In fact, in 84 percent of organizations, data analytics projects were delayed due to the data not being in the right format while in 82 percent, the data used was of such poor quality that analytics projects needed to be reworked. Almost all respondents (91 percent) believed that work was needed to improve the quality of the data within their organization.
The Impact of Untrustworthy Data
Clearly, the importance of data is understood — as is the importance of using the correct data. Many decision-makers have realized that their data problems need to be fixed before using that information to power effective decision-making in the organization.
However, according to the Vanson Bourne study, about half (54 percent) of organizations are still using poor quality or untrustworthy data to make decisions. Using what they feel is bad data has pushed many to not use their organization’s data until it’s more trustworthy, but it seems not enough of those in a decision-making role understand the problems that bad data can cause:
- Flawed Decision-Making: Bad data risks support flawed decisions and hinder the organization from achieving its goals. For organizations where data silos exist, it is possible that data that should be used in analytics is missing or incomplete or that business analysts and decision-makers aren’t even aware that they are missing data, making it even harder to judge the reliability of decisions. When data analytics is delayed, the potential of data to inform timely decisions and actions is negatively impacted, with market opportunities missed and competitive advantages quickly lost.
- Waste: In addition to organizations not being able to make timely and well-informed decisions, both time and money are being lost by organizations where data analytics have to be reworked due to Data Quality and formatting issues. Currently, an average of four working hours is being lost per employee per week due to the need to resolve issues related to preparing data for analysis.
- Lost Revenue Opportunities: In addition, Vanson Bourne reported that more than three quarters (76 percent) believed that revenue opportunities had been missed in their organization due to a lack of data insights. If organizations aren’t able to trust data, they are less likely to take calculated risks based upon it, causing them to move forward too cautiously and, in turn, miss opportunities that may have put them ahead.
- Negative Customer Experiences: A negative customer experience is another way that bad data can affect an organization. 72 percent of decision-makers surveyed said that they’re seeing a negative impact on customer engagement and satisfaction due to missing or incomplete data.
Earning Data Trust
So what can an organization do to rebuild trust in its data?
In order to capture the trust of your organization’s decision-makers, you need to re-establish the foundation that your data and analytics functions are built on. Once executives and decision-makers see that the correct processes are being followed and proper analysis is done, then they will once again have faith in the conclusions being produced — and will embrace them wholeheartedly to power their decisions.
There are a few key ways that this can be accomplished: by improving the data analytics process, integrating disparate data sources, and investing in data automation:
Process Improvements: To close the data trust gap, one of the first places you need to focus on is how data is collected, stored, and analyzed. Better data cleansing and quality management around the sourcing, capturing, and collecting of your data is a great place to start.
Integration: Data that is left disconnected and in its own organizational silo is a large cause of data distrust. By integrating data together across the organization, executives and decision-makers will immediately face fewer issues accessing, analyzing, and utilizing data effectively. Analytics can now be based on full datasets instead of partial data.
Modern integration systems also enable easier migration of data held in legacy infrastructure over to modern data warehousing platforms. These systems can also help to cleanse, standardize, and de-duplicate the data through the integration process, which means that far less time needs to be spent refining the data before it can be used to generate reliable insights.
Automation: Automation also plays a key role in better enabling analytics success and improving data trust within organizations. If teams are able to better automate the tasks involved in Data Management, they will avoid the time lost by employees and be able to put it towards more strategic projects. This process will go a long way towards building more trust in the overall data analytics function. When asked by Vanson Bourne, 94 percent noted that existing processes surrounding their data storage, management, and analytics systems could be more automated, which would free them up to apply their hard-earned skills elsewhere.
In addition to saving employee time, automation enables your data to be loaded into systems more efficiently and with less error. This means that accurate, quality data can be ready for analytics faster and be prepared for use by decision-makers in a quicker and more efficient way.
Automation can also help to address challenges in the identification and integration of new data sources, as well as the migration of data from legacy systems. It can automatically detect duplicate, erroneous, or missing data or identify structures or formats that don’t match the data model. Doing this means the delivery of data for analytics is more reliable and efficient, ultimately enabling a better data-to-decision process.
Conclusion
It is clear that the difficulties organizations have in locating, integrating, and moving their data is causing them to lose trust in the data itself. If data isn’t well integrated into an enterprise storage or warehousing solution, employees won’t be able to find the valuable data they need in order to conduct proper analysis on it — meaning they will be frustrated and quickly seek out other, potentially even worse solutions to address their need for information.
Better awareness and integration of data sources raises the standard of actionable decisions taken in an organization, and consequently, the trust and confidence in them. Trust that the decisions being made are the correct ones will increase once trust in the data on which they are based has been established.