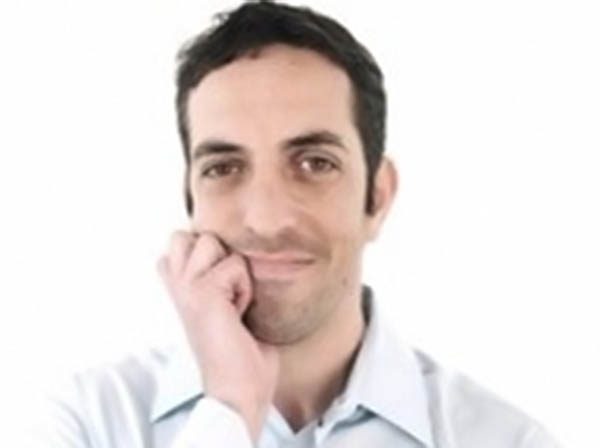
Click here to learn more about Amit Levi.
We’ve come to rely on business intelligence (BI) tools as an essential part of business decision-making. From dashboards to automated reports, BI tools allow us to cash in our data for top-level insights.
We won’t deny that using traditional BI tools can make tasks such as reporting much more efficient, but the reality is that they can create a false sense of security surrounding data awareness. BI has several critical shortcomings that prevent businesses from optimizing business monitoring and incident remediation.
- BI Tools Don’t Dive Down into the Details: One of the main limitations of BI tools is the lack of granularity for the information parsed. For example, a case study from adtech giant Rubicon Project found that their analysts had to discern trends in their data through guesswork and intuition.
- Alert Storms Divert Users from Actual Business Incidents: Alert storms refer to the time that BI operations teams spend sifting through countless alerts, which are often just symptoms of one core issue. These alerts tend to make it more difficult to pinpoint the issue amongst the constant noise on dashboards. They also can lead to a cry-wolf situation where users stop paying attention to the storms, thereby missing a critical incident.
- False Positives and Reactive Management: Another issue with setting recurring alerts is that calibrating these static thresholds manually can often trigger too many anomalies (false positives) or not enough (false negatives).
- They Can’t Account for Seasonality: Seasonality refers to changes that we can expect periodically. Traditional BI tools are generally calibrated with static thresholds that often don’t account for seasonal patterns in the data.
- Business Insight Latency Delays Problem Discovery: As you probably know, a business event requires a real-time response. This means that you need to process a high volume of data in real-time. Many traditional BI dashboards have a data latency, which means they don’t have the capacity to process data in real-time. Consequently, businesses often discover issues after the fact and find themselves taking a reactive approach to remediation.
In short, the shortcomings of traditional BI tools are that they’re static, retrospective, and reactive. In our fast-moving, data-centric world, we need more than traditional approaches to detect and respond to business events.
The Benefits of AI Over Traditional BI
Now that we’ve discussed the shortcomings of traditional BI, let’s look at a few of the benefits of integrating artificial intelligence into your analytics stack.
AI Powers Modern Decision-Making
One of the realities of BI dashboards is that important business incidents that only affect one segment of the business can easily get lost in the sea of statistics. The fact that key metrics are being summarized into single, top-level KPIs means that small anomalies, which often represent the largest untapped opportunities, can easily go overlooked.
An automated AI analytics platform, on the other hand, can monitor millions of metrics at the most granular level, so you can respond to even the most subtle revenue leaks and business incidents. In addition, the fact that machine learning can eliminate business insight latency means that you can make better business decisions when it matters most.
AI Insights are Better Than Ever (and Improving Exponentially)
The 2010s saw incredible advances in AI, particularly in the area of deep learning. As the slide below from renowned AI expert Andrew Ng highlights, one unique aspect of deep learning is that, in general, as you feed artificial neural networks more data, the performance often continues to improve (although this isn’t the case 100 percent of the time).
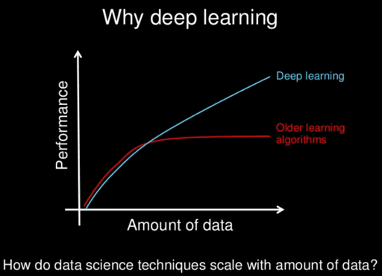
As organizations keep generating more data, it is easy to understand why AI-powered insights are so valuable for decision-making.
Traditional BI Dashboards are Not Enough
When traditional BI dashboards entered the business world, they were intended to help streamline KPI reporting and provide visibility into a company’s performance. This has certainly helped the business analysis process as a whole, but visibility falls short of intelligence.
In reality, KPI dashboards with nice visualizations for business intelligence only scratch the surface. AI analytics, on the other hand, are more valuable for delivering actionable insights. Using machine learning algorithms to alert you to anomalies in real-time, for example, gives your team the information they need to respond intelligently to business incidents without their having to keep checking dashboards.
Avoid Big Data Overload
It’s now reported that we generate over 2.5 quintillion bytes of new data per day. On top of the sheer quantity of data generated, the types of data, and how we store it are changing as well. As described in a Datamation article on shifting from BI to AI:
“Older BI tools can’t cope with larger volumes of data, and they also find it difficult to process data from new applications; it often takes a lot of manual adjustments to make an old BI tool fit a new app.”
Instead of traditional BI, the article suggests that with data metrics now reaching into the millions, each with its own unique behavior, it becomes impractical for teams to monitor without the added cognitive power of machine learning.
AI Offers Real-Time Actionable Insights
One of the fatal flaws of big data dashboards is that the visualizations aren’t actionable. BI visualization can certainly give you a general idea of what has already happened, but they miss the ever-important question of why an incident is happening.
How AI is the Next Evolution in Business Analytics
Now that we’ve discussed the need to look beyond traditional BI and reviewed a few of the benefits of AI analytics, let’s look at real-world examples of ways many forms of AI are changing the face of business in industries such as finance, eCommerce, telecommunications, and healthcare.
Finance
With the sheer amount of financial data generated every day, AI in finance is a match made in heaven. As described in this Towards Data Science article, a few examples include:
- Credit Decisions: From credit scoring to loan assessment, AI provides a framework for more nuanced analysis compared to traditional credit scoring systems.
- Risk Management: The combination of enormous processing power, vast amounts of data, and cognitive computing all allow AI to analyze the history of risk management and identify potential future issues in real-time.
- Trading: Now that at least 70 percent of trading is attributed to algorithmic or high-frequency trading, AI is commonly used to analyze structured and unstructured data in order to improve forecasting systems.
- Revenue and Cost Monitoring: Autonomous business monitoring can be used, for example, to monitor marketing costs over various channels and campaigns. In terms of revenue, AI can be used to monitor income streams across segments, plans, products, and payment providers.
eCommerce
eCommerce sales are expected to double by 2023, and AI in eCommerce is growing right along with it. In particular, a few applications of AI in eCommerce include:
- Chatbots: Love them or hate them, Gartner estimates that 85 percent of customer interactions will occur without human intervention by the end of 2020.
- Personalized Search: Offering contextualized and personalized search to each and every customer is exactly what AI excels at.
- Product Recommendations: On top of search, machine learning powers personalized product recommendations to fit each customer profile. In fact, McKinsey & Company estimates that Amazon’s recommendation engine drives 35 percent of total sales.
- Customer Experience Monitoring: AI for customer experience monitoring means that the solution is monitoring the audience’s journey, experience, and engagement within the app. Autonomous monitoring can improve customer satisfaction, reduce churn, and ultimately increase revenues.
Telecommunications
AI has many applications in the telecommunications industry, for example, in operations support system (OSS) and business support system (BSS) monitoring:
- Telco Monitoring: AI can be used to constantly monitor and correlate business and network performance, providing real-time alerts and forecasting.
- OSS Monitoring: OSS monitoring has traditionally been handled by a rules-based system, although as they become increasingly complex, AI is the logical evolution. In particular, using AI to automatically update and respond to real-time changes in the network is crucial to OSS automation.
- BSS Monitoring: As Ericsson’s CTO highlights, examples of AI already being used in BSS software include customer retention, chatbots, as well as revenue and cost forecasting.
Healthcare
Many hospitals are sitting on years worth of data and are just starting to realize that this is an asset they can leverage to build machine learning models. As highlighted in this article on revolutionizing healthcare analytics, a few of the applications of AI in healthcare include:
- Drug Discovery: As traditional drug discovery often requires up to 12-15 years of research and development, using machine learning to accelerate pharmaceutical research is expected to make drug development faster, cheaper, and more effective.
- Efficient Diagnostics: AI is currently being used extensively for skin cancer diagnosis. In particular, Stanford has created algorithms that can visually diagnose a potential cancer cell with a database of 130,000 images.
- Treatment Using Technology: Now that video conferences and smartphones have drastically improved the ability to provide remote treatment, AI can help telemedicine by improving diagnosis and resolving the logistical challenges associated with long hospital wait times.
- Optimizing Duty Allocation: One of the biggest challenges in healthcare is allocating the optimal number of staff on duty at a given time. AI and big data analytics help solve this by efficiently predicting the adequate number of staff required.
Summary: Transitioning from BI to AI
As we’ve discussed, traditional BI tools have certainly helped make the reporting process more efficient, although when it comes to handling the speed and volume of data generation today, they have serious shortcomings.
As we saw, AI is effecting significant change in practically every industry, especially in the case of AI analytics.
Using AI for real-time, scalable analytics can secure a competitive advantage for today’s online business by improving customer experience and product performance and preventing revenue loss.