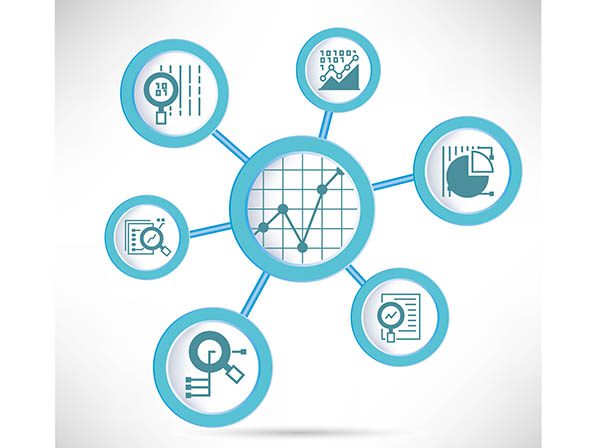
The general pattern of technology upgrades in Enterprise Analytics & Business Intelligence that occurred in 2019 signaled the emergence of some specific types of analytics: smart, real-time, augmented, and conversational. These trends will continue growing well into 2020 and beyond.
Also included in that list are: Smart devices and multi-channel data will require smart analytics tools for improved insights; real-time analytics will be a necessity in the era of sensors and streaming data; augmented analytics will free up Data Science professionals for high-value tasks by enabling citizen data scientists to conduct analytics and BI on their own; and finally, conversational analytics is the new norm in the world of analytics, where NLP’s text capability will be replaced by voice:
“Ecosystem orchestrators use data to connect the dots — by, for example, linking all possible producers with all possible customers, and, increasingly, by predicting the needs of customers before they are articulated. The more a company knows about its customers, the better able it is to offer a truly integrated, end-to-end digital experience.” — McKinsey, Analytics Comes of Age
According to The Global State of Enterprise Analytics, 2020, 65 percent of global enterprises are likely to increase their expenditure on business analytics. Out of the 500 organizations surveyed, 94 percent reported that “data and analytics” are critical to business growth. Forty-seven percent of current analytics platforms are cloud-hosted, and 59 percent of the enterprises have already implemented advanced analytics.
Jim Hare, research vice president at Gartner, said, “As intelligence is at the core of all digital businesses, IT and business leaders continue to make analytics and BI their top innovation investment priority.”
BI & Analytics Trends Forecasted for 2020
Twelve Curated Trends for 2020
- Smart Analytics: Currently, the business IT ecosystem is rapidly embracing smart devices, smart technologies, and smart tools to cut operating cost, enhance business efficiency, and optimize business processes. So it is only natural that the next big transition in the world of analytics will be “smart analytics” to suit the needs of smart (data-technology driven) businesses. The primary driving force behind the smart analytics movement is AI and allied technologies.
- Democratization of Analytics: More and more, enterprise analytics teams will combine experts and non-experts to enable mainstreaming of analytics activities. A few Data Science staff members may team up with ordinary business users with day-to-day business knowledge to encourage wide participation and “diversity of insights” from analytics. Citizen data scientists will surpass the real data scientists in delivering advanced analytics in 2020.
- Augmented Analytics: This type of analytics will chiefly rely on machine learning (ML) for data preparation, data exploration, and automated model development. The insights from these systems may be extracted by business users and citizen data scientists without the help of Data Science staff. Gartner has suggested five major trends are shaping analytics and BI, and that augmented analytics will be the top selling feature of analytics solutions in 2020.
- Increased Data Literacy: The previous trend will push the need for increased data literacy in an enterprise.Ordinary business users will realize the need for personal data literacy.
- Augmented Data Management: With the rapid automation of Data Science platforms due to AI, ML, NLP and other advanced technologies, organizations are finding Data Management the next target for full automation. As data integration, metadata management, MDM, or Data Quality Management become more automated, the lack of Data Science talent, once a threat to global businesses, is no longer a concern. Analytics and BI vendors are increasingly adding AI and ML capabilities to provision “self-configuring and self-tuning” Data Management processes. Now the few data scientists available in a typical data center environment can concentrate on complex technical projects.
- Conversational Analytics: While NLP made asking questions and getting answers from data easier through conversational language, “Conversational Analytics” takes a step forward by enabling verbal question-answer sessions instead of text. It has been predicted that by 2021, NLP and conversational analytics will together enhance BI adoption among employees “from 35 percent to over 50 percent,” which may include many new categories of business users.
- Graph Processing and Graph Databases: Graph processing involves graphically representing how “entities such as people, places, and things” are related to each other. This technology has wide applications — from fraud detection to social analysis to genome research. Gartner predicts that currently graph processing is growing at 100 percent annually and this trend will continue through the next three to five years.
- Continuous Intelligence: In recent times, sensor-driven IoT technology, streaming analytics tools, and cloud platforms have fueled the demand for real-time analytics and now for “continuous intelligence,” which is intelligence gathered in real time. According to Gartner, by 2022, more than 50 percent of new enterprises will implement continuous intelligence for improved decision-making.
- Persistent Memory Servers: With the phenomenal rise of data volumes, the typical size of database memory is posing a challenge to in-memory database processing. The newer servers deliver more speed and better performance, but only if the storage and memory capacities are adequately matched. Persistent memory technology promises to deliver “insights from data,” but the technology may take some years to mature.
- Explainable AI: Explainable AI is the fitting answer to regulatory nightmares in case of model application. To increase transparency and reliability of model-driven outcomes or insights, this technology helps to “describes a model, highlight its strengths and weaknesses, predict its likely behavior and identify any potential biases.” Without the use of this technology, AI- or ML-driven outcomes can cause serious regulatory or reputational headaches.
- Data Fabric: This custom-designed solution delivers usable data services — data access, sharing, or distribution — through a “combination of data integration approaches.” It is meant for a distributed data environment.
- Commercial Adoption of AI and ML Research Platforms: So far, the bulk of AI and ML research have been carried on open-source platforms. Now that is changing. The newest trend in AI is commercial players entering the advanced technology research and application development market with partnership models.
From many more trends published as “Year 2020 Analytics & BI Trends” by industry publications, the author of this post has chosen to share the above 12, based on personal understanding of where the digital technologies are headed next year.
Data Monetization: A Key Motivator for Technology Adoption
While Data Science, Analytics, and BI solutions are constantly evolving — making way for smarter, quicker, and more agile platforms for business analytics — one important thing to remember is that enterprises are always motivated by the thought and prospect of “more revenue.” The broad business expectation is that advanced data technologies, coupled with advanced automation technologies like AI, ML, and NLP will collectively contribute to creating enterprise “Data Assets” leading to data monetization. Now that the cloud is mainstream, businesses of all shapes and sizes will have the option of selling their data or insights to external customers.
Image used under license from Shutterstock.com