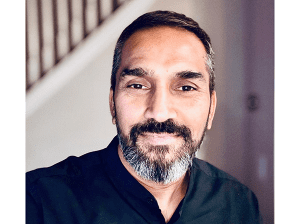
In today’s data-driven world, organizations manage vast amounts of data. As enterprises expand and grow business functions, there’s corresponding linear growth in operational data. This encompasses both master data and transactional data. While master data might change less frequently than transactional data, implementing data governance practices and defining data retention policy is crucial to maintaining data integrity and ensuring systems operate with accurate, necessary, and up-to-date data.
A data retention policy lets you define what and how far back data needs to stay in the transactional processing (OLTP) and outgoing data management systems. While this practice is not limited to OLTP and outbound data, the data retention policies differ from system to system and are not necessarily the same across all the enterprise functions. For instance, promotional data in retail may be needed as far back as three years to support data analytics and audit functions, whereas the order management system (OMS) may hold the orders history for just one year.
A well-defined data retention policy is crucial for ensuring compliance with legal and regulatory requirements, optimizing storage costs, and mitigating security risks. However, striking the right balance between retaining data for business needs and minimizing potential liabilities can be a complex task.
For the rest of this blog post, we will focus on data retention policies in OLTP and outbound data management systems.
Defining OLTP Data Retention
Online Transaction Processing (OLTP) systems are the backbone of any enterprise, capturing transactional data in real time and dealing with data generated via day-to-day business operations. This data is critical for daily functions, but its value diminishes over time. Examples of transactional data include Basic Master Data (BMD), Extended Master Data (EMD), and pure forms of day-to-day operations like typical banking transactions of withdrawal (Debit) and deposits (Credit). The increasing volume of master data and transactional data can negatively impact system performance, resulting in slower transactions and degraded user response times.
While the application or data architect may implement measures to improve overall system performance, data retention is a key consideration for maintaining the health and stability of the transactional system. Lastly, it’s essential to establish a retention policy that aligns with business needs and legal requirements.
Key considerations for OLTP data retention:
- Legal and regulatory requirements: Different industries and jurisdictions have varying data retention mandates. It’s essential to understand and comply with these requirements to avoid legal repercussions. Financial enterprises may need to retain transactional data for several years vs. retail enterprises may consider only two to three years, for example.
- Business needs: Organizations need to retain OLTP data for a sufficient period to support business operations, such as financial reporting, customer service, and fraud detection. In retail, the enterprise may retain online sales transaction data in hot storage to train machine learning models that compute demand forecasts or to generate comparative business intelligence reports.
- Storage costs: Storing large volumes of OLTP data can be expensive. Implementing a tiered storage approach, where data is moved to less expensive storage as it ages, can optimize costs. Consider hot and cold storage options to move the data in frequent use. Use data table compression techniques to minimize storage costs for less frequently used data.
- Data security: OLTP data often contains sensitive information, such as customer details or financial transactions. Robust security measures, including encryption and access controls, are essential to protect this data from unauthorized access. This consideration is most important when there is secondary storage for infrequent data.
- Create buckets: When you are dealing with large troves of transactional data, it’s advisable to divide transactional data into different buckets to group data by retention windows and ease maintenance. For example, creating buckets of reporting and non-reporting data can ensure data is present for future audits.
- Purging and configuration management: Enterprises purge transactional data periodically using automated methods to maintain the ongoing active data window. Creating configurations or parameterizations for each functional domain or type of data can help develop individual or group retention swim lanes. For example, sales, inventory and order data can exist as one group, whereas pricing, cost, and financial data can be defined as another group.
- Retention robustness: Along with the definition of purging and configuration, simplify the data retention process in collaboration with key business stakeholders to ensure governance adoption. By developing user-friendly IT tools, one can ensure long-term maintainability and adaptability to evolving business needs. This approach significantly improves flexibility and effectiveness of the data retention process.
- Governance practice: Establish a regular review process to prevent data retention policies from becoming unmanageable and ineffective. establish a cross-functional governance body responsible for periodic review and making policy amendments. This ensures the enterprise follows current best practices and retires outdated procedures.
- Administration: Finally, all policy amendments should be processed through a single, standardized channel to ensure centralized decision-making and maintain a clear audit trail. The centralized approach facilitates SOX compliance, which is essential for effective auditing.
Outbound Data Retention
Outbound data refers to the data that is shared within the enterprise across different systems and with external parties, such as customers, partners, and vendors. This data can be in various forms, including emails, documents, reports, any incoming or outgoing data. Managing outbound data retention is challenging due to the lack of control over how the data is used and stored by external parties. However, organizations can still implement measures to mitigate risks and ensure compliance.
In the context of this article, we will discuss outbound data elements produced by the OLTP applications and what the retention policies are for outbound data publication.
Key considerations for outbound data retention:
- Contractual agreements: Include data retention and deletion clauses in contracts with external parties to define expectations and responsibilities. Work with data consumers in defining what is desired retention in each business case, as you may integrate with different consumers in the ecosystem. In retail, for example, a business may rely on a third party for UPC data and vendor product supply, so it’s essential to agree on the data shelf life.
- Data minimization: Share only the necessary data with external parties and limit access to sensitive information. Creating a secured abstraction layer on top of your baseline data is desired in such cases to minimize data sharing outside the network. Aspects of “data clean room” should be applied using options such as Habu or Samooha platforms. Your organization can set time limits on data access to ensure updates happen regularly or cut off access when needed.
- Monitoring and auditing: Regularly monitor and audit outbound data to ensure compliance with policies and identify potential risks. Conduct security audits to ensure sensitive information is not shared through shared containers or flat files. This may pose a challenge because data is moved out of the database and encapsulated before it is sent. Defining data encryption and decryption is also a key component to consider.
- Data categorization: Create data categories as the enterprise may share data in different types and forms using short messages, and messaging queues like Kafka or traditional flat file formats. Each data type and form may need a separate setup and data retention policy to support the overall function and audit requirements.
- Data deletion: As established in the OLTP retention above, creating hot and cold storage for outbound data saves infrastructure and storage costs. Establish procedures for deleting outbound data when it is no longer needed or required by law. General industry practice for outbound data retention is seven days but can vary depending on consumer preference. For example, A Kafka topic in a cluster may retain outgoing messages up to seven days before being purged.
Best Practices Summary for Data Retention
- Develop a Comprehensive Policy: Create a clear and concise data retention policy covering all types of data, aligned with business needs and legal requirements.
- Regularly Review and Update: The data retention policy should be reviewed and updated periodically to reflect changes in business needs, legal requirements, and technology.
- Communicate and Train: Ensure that all enterprise teams are aware of the data retention policy and understand their responsibilities.
- Use Technology: Leverage data management tools to automate data retention processes and improve efficiency.
By implementing a well-defined data retention policy and following best practices, organizations can effectively manage their OLTP and outbound data, ensuring compliance, optimizing costs, and mitigating risks.