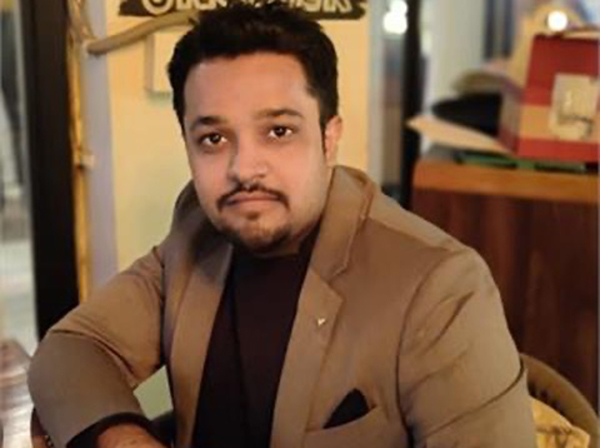
As digital technologies spread their roots in the business world, organizations have access to more data than ever. This data becomes an essential organizational asset when utilized for enriching business strategies. But the process of turning data into insights is as important as the data itself. Hence, more and more organizations across sectors are deploying advanced analytics to grow their business. Utilizing analytics to convert raw data into usable insights requires a well-defined analytics strategy covering people, technology, and execution.
Why Do Enterprises Need an Analytics Strategy?
There are two primary objectives of an analytics strategy:
- To use analytics sufficiently across different business processes
- To leverage analytics to outshine the competition
While most organizations have an analytics strategy, their success depends on aligning people, technology, and execution. To understand this better, let us understand the three key pillars of an analytics strategy.
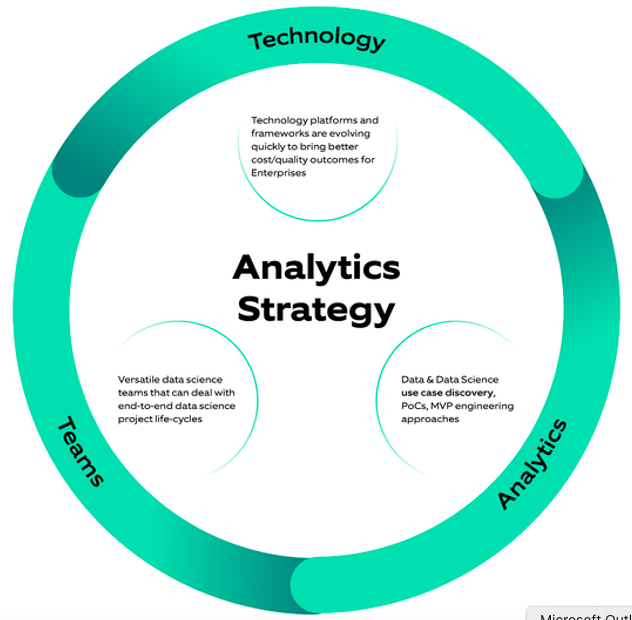
Teams: One of the first few steps would include assessing the nature of analytics and having data science teams that can execute the end-to-end analytics projects completely. An organization with an unfledged analytics strategy generally has one or two people to begin its analytics projects. In contrast, an organization with a matured analytics strategy has bigger and more versatile analytics teams.
Technology Platforms and Frameworks: The right tools, technologies, and frameworks required to ensure smooth analytics project execution infrastructure are crucial. Organizations shouldn’t be restricted to a limited set of languages, tools, or technologies to carry out all important project phases. A matured analytics strategy ensures they have all necessary technologies and frameworks in place.
Analytics Initiatives and Execution: Ensuring that analytics initiatives aim to deliver business value and shouldn’t be limited to just a few use cases or smaller scope projects. Following a complete project, lifecycle ensures maximum value realization for the business. Companies must ensure they have good mechanisms for use case discovery, proof of concepts (PoCs), minimum viable products (MVPs), and support for various advanced analytics projects.
How Do You Develop an Effective Analytics Strategy?
The following are a few tried-and-true methods for creating an effective strategy.
Building versatile teams that drive end-to-end analytics project lifecycle: Many organizations fall prey to the misconception that data engineers, data scientists, and ML engineers are sufficient for delivering end-to-end analytics projects, which is not true. A versatile data science team must have people with varied skill sets.
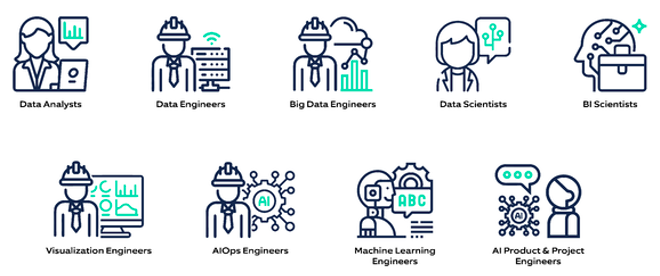
These team members play different roles in different project stages. For instance, a data scientist acting as a consultant will identify the relevant use cases and estimate the expected ROI and its priority. But the same data scientist will not be expected to write code or develop models.
A successful analytics strategy requires teams to work together in the long run. And even though finding people adept at technologies such as cloud engineering and modeling ability is difficult, organizations need to understand that the role of the business personnel is as significant as the technical team members. The team must follow a business-first approach rather than a technical-first approach.
It is equally important to educate people about analytics and inspire and motivate them with successful analytics examples. Organizations can do this by conducting regular workshops.
- Inspiration workshops for C-level executives: These workshops are aimed at helping executive teams to understand how they can deploy analytics to beat the competition. And understand the most effective ways an organization can use advanced analytics.
- Analytics and AI workshops for middle management: Meant for managers, these workshops ensure that the management teams are familiar, comfortable, and well-trained in data engineering, data science, machine learning, and AI. These workshops will enhance their understanding of these technologies and the best practices in the respective industry.
- Workshops for data citizens: These workshops enable access to transparent and trustworthy data within an organization. Conducting exercises utilizing data mining techniques, data science methods, and visualizations can help build a data-driven culture within an organization.
Selecting tech platforms and frameworks for enterprise analytics: There are three major approaches that an organization can adopt when selecting technology platforms and frameworks for analytics:
Traditionally, IT teams ran the analytics programs, extracted data from various sources, fed that into a data warehouse (DWH), and then ran reporting over it. They either did this directly or with the help of some external vendor. Then came the significant data era where the IT teams realized working with DWHs was insufficient. They also needed to leverage data lakes/scale storage and run various analytics initiatives.
However, the business teams own the data, not the IT teams (they own the infrastructure). Hence, IT teams became the default data and analytics operations custodians. As organizations grew bigger and better, they realized the mistake and brought data meshes, fabric, and virtualization to address the problem.
A data mesh involves IT teams creating a library of tools that would make it simple for a business team to have a small data lake (logical data lake) and ultimately build a data product on top of it. It gives them a mechanism to extract, store and expose outcomes from data analysis and helps create designs that decide how data lives in an organization. We believe that data will live as a data product inside businesses rather than with the IT teams.
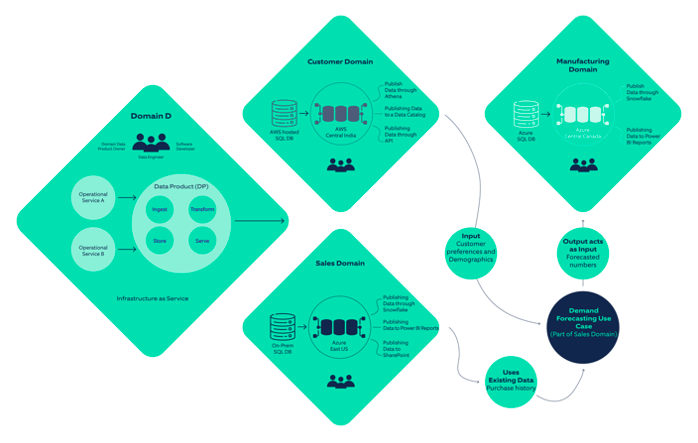
Another important factor is enterprise AI. Different teams within an organization build individual data science and AI models, each working with multiple technologies. Consequently, organizations end up with numerous tech stacks. Therefore, enterprise AI becomes important. AI enterprise platforms like H2O.ai, DataIQ, and DataRobot offer simple and common platforms to design workflows leading to common work culture, codebase, and training pipelines. These platforms drive synergy and efficiency in organizational working. The market is flooded with enterprise AI platforms, and organizations must choose the platform that meets their requirement best.
Organizations increasingly prefer smaller and simpler code for each use case. They want to use previously written codes and leverage them going forward. Low-code platforms make this possible; even business teams can create apps without extensive coding knowledge and potentially bridge skills gaps in their organizations.
There is a host of tools and technologies that organizations can use to improve their data-driven initiatives. These include data mesh, data fabric, data lakehouse, feature engineering platforms, AI workflow management tools, machine vision libraries, NLP libraries, and enterprise AI platforms.
Executing the analytics strategy with synergy between teams: Once you have the right team and tools in place, it is important to have an effective execution strategy.
Let’s understand execution as a complete workflow:
- Identify the business problem and ideate to build a use case
- Frame the use case in analytical form
- Design algorithms
- Build a proof of concept to evaluate the ROI
- Create a minimum viable product (MVP)
- Deploy MVP at scale – as a product and manage it from an operational perspective in the longer run
Business teams are responsible for identifying problems and use cases and prioritizing them. They then frame the use cases in an analytical form with help from the data science team. The data science team also builds algorithms and executes the proof of concept. Once the PoC is ready, the engineering teams come in and assess how they will use this model, develop data and inference pipelines, see what the MVPs would look like, and deploy them. Lastly, the operations team comes in and supports the product operations.
The business and tech teams must have synergy throughout the entire analytics journey.
- Business teams must share details on operational analytics (KPIs, metrics, and measures) with the data science team to enable visualization around a business process. This will help investigate performance and opportunities. For instance, the supply chain team can engage with the data science team to build interactive dashboards that help understand where data science interventions can help improve supply chain efficiency.
- The data science and engineering teams must be aware of the enterprise platforms and accelerators being used by each other so that they can leverage them for other similar use cases. For example, a code developed in the early stages of a PoC/ prototype can also be reused in the MVP phase.
Advanced analytics can help extract the actual value of your organizational data. But it is crucial to have the right strategy to leverage analytics for business growth.