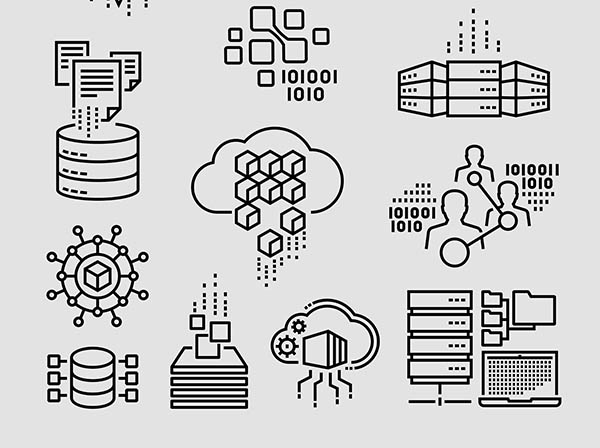
In 2019, automation frameworks designed to process big data made it much easier to go from the start of a new analytics project to the production phase. Additionally, per the requirements of GDPR, many more businesses now have Chief Protection Officers (and likely Chief Data Officers), which has, in turn, resulted in their shifting from ad hoc analytics to more efficient, streamlined big data platforms.
It is predicted that data volumes will continue to grow ever larger in 2020 as well. A report from IBM said there would be as many as 2.72 million data science jobs available by 2020 to help organizations deal with such data volume and that is proving to be true. The continuing use of big data will impact the way organizations perceive and use business intelligence. Some big data trends involve new concepts, while others mix and merge different computer technologies that are based on big data. For example, machine learning is being merged with analytics and voice responses, while working in real time. Another example is combining blockchain with the internet of things (IoT).
Machine Learning
Automation and machine learning tools help in developing insights that would be difficult to extract by other methods, even by skilled analysts. The combination provides faster results and boosts both general efficiency and reaction times.
In 2020, the IDC has predicted the IoT will merge machine learning with streaming analytics. Sue Green’s blog provides an update.
Big Data Analytics
Analytics provides a competitive advantage for businesses. Gartner is predicting that companies that aren’t investing heavily in analytics by the end of 2020 may not be in business in 2021. (It is assumed small businesses, such as self-employed handymen, gardeners, and many artists, are not included in this prediction.)
The real-time speech analytics market has seen its first sustained adoption cycle beginning in 2019. The concept of customer journey analytics is predicted to grow steadily, with the goal of improving enterprise productivity and the customer experience. Real-time speech analytics and customer journey analytics will gain significant popularity in 2020.
Continuous Intelligence
“Continuous intelligence” is a system that has integrated real-time analytics with business operations. It processes historical and current data to provide decision-making automation or decision-making support. Continuous intelligence leverages a variety of technologies (optimization, business rule management, event stream processing, augmented analytics, and machine learning). It recommends actions based on both historical and real-time data.
Continuous intelligence promises to provide more effective customer support and special offers designed to tempt specific customers. The technology has the potential to act as a “core nervous system” for organizations such as trucking companies, airlines, and railroads. These industries could use continuous intelligence to monitor and optimize scheduling decisions. Continuous intelligence is a fairly new technology, made possible by augmented analytics and the evolution of other technologies.
Gartner predicts over 50 percent of new business system will be using continuous intelligence by 2022. This shift has started, and many organizations will incorporate continuous intelligence during 2020 to gain (or maintain) a competitive edge.
Augmented Analytics
Augmented analytics automates the process of gaining business insights through advanced artificial intelligence and machine learning. An augmented analytics engine automatically goes through an organization’s data, cleans it, and analyzes it. As a last step, it converts the insights into actionable steps with little supervision from a tech person. Augmented analytics can make analytics available to smaller businesses by making it more user-friendly.
In 2020, augmented analytics will become the primary purchase of businesses dealing with analytics and business intelligence. Internet businesses should plan on adopting augmented analytics as their platform capabilities mature (or finding a cloud that offers augmented analytics). The technology has disrupted the analytics industry by merging artificial intelligence and machine learning techniques to make developing, sharing, and interpreting analytics easier.
On the Edge
As stated before, the IDC has correctly predicted the IoT will combine streaming analytics and machine learning by 2020 – this trend will continue to grow.
Analytics Insight predicts the internet of things will be merged with data analytics in 2020. Gartner is predicting the automotive and enterprise IoT market will expand to include 5.8 billion endpoints during 2020, rising by 21 percent from 2019. In large technical organizations already using IoT devices, intelligent business leaders are implementing the assisting technology needed to run data analytics for maximum efficiency.
The primary goal of combining the IoT with machine learning and data analytics is to improve the flexibility and accuracy of responses made by machine learning, regardless of the situation. Additionally, this kind of system is being fine-tuned with the hopes of improving interaction with human beings.
In-Memory Computing
“In-memory computing” describes the storage of data inside the random-access memory (RAM) of specific dedicated servers, instead of being stored in complicated relational databases running on relatively slow disk drives. In-memory computing has the added benefit of helping business customers (including banks, retailers, and utilities) to detect patterns quickly and analyze massive amounts of data easily. The dropping of prices for memory is a major factor in the growing interest of in-memory computing technology.
In-memory technology is used to perform complex data analyses in real time. It allows its users to work with large data sets with much greater agility. According to Analytics Insight, in 2020, in-memory computing will gain popularity due to the reductions in costs of memory.
The problems of using in-memory computing are becoming fewer and fewer, the result of new innovations in memory technology. The technology provides an extremely powerful mass-memory to help in processing high-performance tasks. It offers faster CPU performance and faster storage, while providing larger amounts of memory.
The GDPR and other Regulations
GDPR went into full effect in May of 2018. The California Consumer Privacy Act is scheduled to go into effect in January of 2020. Many American corporations try to avoid dealing with the new regulations and have made successful efforts to block and delay similar American legislation. These regulations have a significant impact on how data is processed and handled, as well as security and consumer profiling. Many organizations who sell their data to others are not thrilled with these new regulations designed to protect consumer privacy. Trends of improving consumer privacy are not based on corporate profits, but on the desires of internet users to maintain their privacy.
The GDPR and the California Consumer Privacy Act are designed to place power back in the hands of the consumer. This has been accomplished by recognizing consumers as the owners of information they create. The GDPR gives consumers the right to remove their data from an organization’s control.
Organizations that apply privacy regulations — rather than focusing on the short-term profits earned from sales of private information — will not have to pay fines to a European country, or California, for breaking privacy regulations. (And if they advertise their respect for privacy, they might increase the loyalty of their customer base.)
Cloud Usage
The “public cloud” is a computer processing service offered by third-party contractor, for free or for a fee. The public cloud is available to anyone willing to use it. Public cloud usage continues to grow, as more and more organizations turn to it for services. 41 percent of businesses are expected to start using public cloud platforms in 2020.
The hybrid cloud and multi-cloud strategies are becoming increasingly popular solutions. Often, organizations will choose to adopt multi-cloud and hybrid strategies for handling a variety of different cloud computing projects, depending on the project needs. Taking advantage of the various best-suited tools and solutions available at different clouds allows organizations to maximize their benefits. Despite the benefits, using multiple clouds can make monitoring expenses, governance, and cloud management more difficult.
Michael Warrilow, a Gartner analyst stated:
“Most organizations adopt a multi-cloud strategy out of a desire to avoid vendor lock-in or to take advantage of best-of-breed solutions… We expect that most large organizations will continue to willfully pursue this approach.”
Image used under license from Shutterstock.com