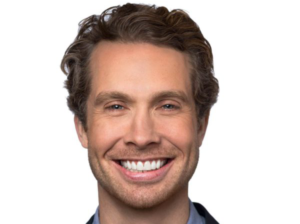
According to a recent McKinsey study, the impact of generative AI (GenAI) impact on productivity could add trillions of dollars in value to the global economy which they estimate will add the equivalent of $2.6 trillion to $4.4 trillion annually across the 63 use cases analyzed. About 75% of the value that GenAI’s use cases could deliver falls across four areas: customer operations, marketing and sales, software engineering, and R&D. The report also stated that the estimate would roughly double if they include the impact of embedding generative AI into software that is currently used for other tasks beyond those use cases.
While some organizations rushed out to learn how they could embrace this new technology, others were more conservative opting to take a “wait-and-see approach.” Whatever the posture, enterprises can expect to see the following generative AI trends in the coming year:
1. Generative AI and large language model (LLM) hype will start to fade
Without a doubt, GenAI is a major leap forward; however, many people have wildly overestimated what is actually possible. Although generated text, images, and voices can seem incredibly authentic and appear as if they were created with all the thoughtfulness and the same desire for accuracy as a human, they are really just statistically relevant collections of words or images that fit together well (but in reality, may be completely inaccurate). The good news is the actual outputs of AI can be incredibly useful if the end user fully considers all of their benefits and limitations.
As a result, 2024 will usher in reality checks for organizations on the real limitations and benefits GenAI and LLMs can bring to their business, and the outcomes of that assessment will reset the strategies and adoption of those technologies. Vendors will need to make these benefits and limitations apparent to end users who are appropriately skeptical of anything created by AI. Key elements like accuracy, explainability, security, and total cost must be considered.
In the coming year, the GenAI space will settle into a new paradigm for enterprises, one in which they deploy just a handful of GenAI-powered applications in production to solve specific use cases.
2. Natural language interfaces will become ubiquitous
Imagine this scenario: you walk into a brick-and-mortar retail store. When you ask the store assistant a question, instead of a verbal response, they point at a display with a list of options or rush over to a whiteboard to sketch an illustration that includes minimal text. In this silent exchange, the richness of human-level communication is replaced by a menu of options or a group of visuals. Odd, right? Yet, this has been the paradigm for most websites for the past 25 years.
There is already a race to create “intimacy at scale on the web” enabled by GenAI and large language models. It is complicated to attain, and the challenge to achieve this personalization is well understood. A small number of vendors have worked out how to overcome these issues in a production environment to enable accurate and trusted interactions with these language models.
As a result, and as these positive experiences multiply in 2024, more individuals will become comfortable with leveraging and maximizing their use of natural language interfaces.
3. Businesses will learn that adding GenAI to existing tools will not address foundational weaknesses
While GenAI can provide valuable assistance, it cannot miraculously solve foundational issues related to volumes of information and relevance of searches through that data. If an existing tool was unable to reliably surface relevant information immediately ten months ago, bolting GenAI onto any of these offerings will fail to make them work better. Similarly, if a solution did not effectively answer questions previously, the mere addition of GenAI would not change its performance. Put simply, when it comes to GenAI, garbage in produces garbage out.
In 2024, a few implementations of Retrieval Augmented Generation (RAG) will emerge as the only possible way to successfully eliminate hallucinations. RAG is an AI framework that attempts to provide a narrow and relevant set of inputs to GenAI to yield an accurate and reliable summary. However, the successful execution of this framework is no easy task and consequently not all instances of RAG are created equal. For instance, if RAG yields pages of results that may or may not be accurate and defers the task of deciphering the correct answer to GenAI, the outcome will once again be subpar and unsuitable for business use.
GenAI faces the same challenge as a human would in trying to summarize ten pages of relevant and irrelevant data. In contrast, both GenAI and humans do a much better job synthesizing ten relevant sentences. Furthermore, RAG alone can still fail to surface highly accurate answers when it comes to answering questions containing domain-specific context. Boosting the result’s relevance requires last-mile fine-tuning of the LLM. The combined RAG + fine-tuning approach will help achieve production-level performance of the GenAI solution for companies next year.
4. Generative AI initiatives will be driven by line of business, not IT
Executives traditionally require organizations to adopt new tools to enable new (and better) business practices and save money, even if the users prefer to stick with what they already know. IT supports the rollout while implementation teams debate change management procedures, conduct extensive training for potentially reluctant users, and stamp out any continued use of the older tools. However, ensuring compliance and achieving the desired benefits quickly is no easy feat.
GenAI will be the opposite in 2024. The enthusiasm among users for GenAI-enabled solutions is palpable, as many have already tried these tools in various forms. The user-friendly nature of GenAI, with its natural language interfaces, facilitates seamless adoption for non-technical stakeholders. However, technical teams are left grappling with inherent challenges, including hallucinations, the lack of explainability, domain-specific knowledge limitations, and cost concerns.
In some organizations, the use of GenAI is forbidden until their technical teams come up to speed. Detecting shadow usage, where individuals become suddenly hyper-productive after a brief period of quiet, adds an additional complication to the implementation challenges. Next year, organizations will work out a process to evaluate the myriad of options available and allow the business to use the few tools that are capable of addressing all of GenAI’s challenges in an enterprise environment.
5. GenAI will streamline new employee onboarding
Organizations continually cope with employee turnover and retirements in a labor-constrained environment. As a result, they are constantly working to hire and onboard new employees. The problem is that these new hires often struggle to navigate complicated and confusing company processes, policies, and specific language used throughout the organization. Existing learning systems frequently fail to surface the right information to answer new hires’ questions. Simultaneously, new employees will likely not know the right way to phrase a question to obtain the information they need when they search for answers hidden in the training materials. In many cases, domain-specific jargon can be non-intuitive, making it difficult for newcomers to communicate their inquiries effectively. This hurdle often leads to longer learning curves and reduced productivity early on.
GenAI, coupled with answer engines, are emerging as a solution to accelerate this process significantly. In 2024, organizations will increasingly leverage GenAI and answer engines to dramatically improve this process. Using these technologies, employees can ask questions in their own words, eliminating the need to master keywords and domain-specific terminology upfront.
These solutions also provide relevant information pertinent to organization-specific services and programs, ensuring that newcomers have the knowledge they need to perform their tasks competently. Moreover, the analytics generated by these systems enable trainers to tailor content, addressing specific learning needs and information gaps. Incorporating answer engines into the onboarding process ensures that individuals become productive contributors to the organization at a much faster pace. By harnessing the power of AI and natural language processing to facilitate learning and knowledge retrieval, new employees can be more excited to provide an impact immediately in their new posts in the coming years.