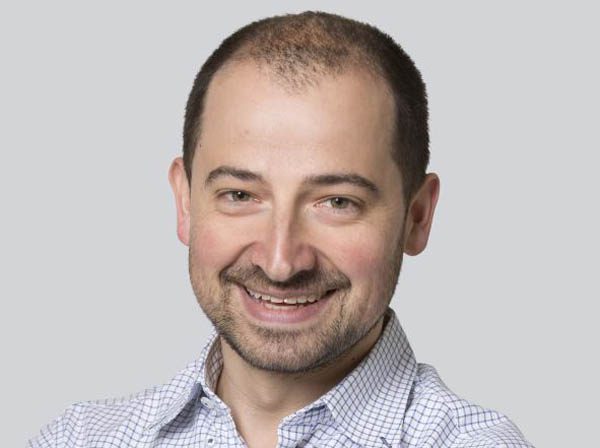
Click to learn more about author Terence Siganakis.
Organizations are constantly coming to us wanting help in becoming more “data-driven.” They want to improve their decision making, shifting the process to be more quantitative and less based on gut and experience. This is a worthy goal but is a little more complex than just putting dashboards in front of managers.
Being data-driven involves more than just shiny new tools. It requires investing in Data Quality, to change behaviors around how data capture systems are designed and used. It also requires investment in cross-team collaboration and in building consistent, enterprise-wide definitions for core metrics to ensure that everyone is on the same page. Investment in these pillars of Data Governance also sets the foundation for even higher-value activities in advanced analytics, machine learning, and automation, where the value add of governance is even more pronounced.
Data Quality Must Be Prioritized
Data Quality is the foundation for all analytics-based activities. As organizations seek to make more decisions based on data, there is a real risk that decisions can be made incorrectly if the data underpinning them is incorrect, incomplete, or inconsistent.
Enterprise applications are notoriously bad in their user experience and the quality of the data they capture. Systems with a poor user experience make it difficult for well-meaning users to enter data effectively. Incentive systems and KPIs often disincentivize time spent on data collection. Customer service representatives are rewarded for quick resolution of tickets, so they jump from one ticket to another without “wasting time” documenting the resolution effectively. A salesperson is incentivized on closing deals, not on accurately and completely capturing the details of the lead or updates on how the process is progressing or details of the sale.
When the primary tasks are incentivized, the quality of data captured suffers. This essentially renders many metrics with real value unusable. How great it would be if finance could make projections on income using accurate estimations of pipeline opportunities in the CRM, or if marketing could judge the effectiveness of its campaigns not just on leads in the door, but how likely they are to convert.
Tips for Incentivizing Data Quality:
- Incorporate Data Quality into performance metrics (e.g., cap commissions to salespeople who have failed to capture data about the sales process effectively)
- Ensure that Data Quality is a KPI for managers
- Ensure that high-quality data capture is a Non-Functional Requirement (NFR) for new system implementations
- Audit Data Quality regularly (e.g., quarterly)
Data Requires Consistency and Clarity for Interpretation
To many, the term “data” is synonymous with “fact,” forgetting that data requires context to be properly interpreted. Context varies by department, business unit, across teams, and even within teams. The differences in context often lead to subtly different definitions of how things are calculated. For example, when marketing talks about “Total Sales”:
- Does it refer to the number of purchases (transactions) or the value of those transactions?
- Does it include returned items? What about when an item is purchased and returned in different periods?
- Does it include shipping costs?
- Does it include sales taxes?
- When a sale is made in a foreign currency, how is the exchange rate calculated?
- Does it include items gifted in promotions?
Many business users who rely on “Total Sales” may not actually know the answers to these questions, which is likely to affect their ability make decisions. Additionally, while marketing may be confident in its definition of “Total Sales,” the finance department may use a subtly different definition, and operations a different one yet again. This differing context across the organization makes it more difficult to collaborate and can leave the executive team scratching their head.
Analytics platforms like PowerBI and Tableau put data in the hands of employees democratizing data across the organization. They also exacerbate the problems of inconsistent metrics. Suddenly it’s easy for Tom from marketing to view Amy from finance’s dashboard, revealing that her “Total Sales” number is different from his. These issues can and do erode trust in these platforms, hurting their adoption and reducing their capacity to add value.
Tips for Consistent Metrics:
- Metrics need to be defined and documented consistently across the organization, with agreement from all organizational units
- Any metric appearing on multiple dashboards should have the same value for the same period
- New and changed dashboards should be reviewed by the analytics team for opportunities to consolidate reports and to check their consistency
- Any changes to calculation methodology of metrics should be widely communicated
- Metric definitions should be easily viewable within any platform that displays them
- Limit the ability for end-users to customize how metrics are calculated
- Periodically review user-created dashboards to ensure that metrics are labelled consistently
Data Governance Is About People and Process, Not Technology
A strong Data Governance framework should be focused on both Data Quality and consistency and clarity. These pillars of Data Governance are firmly rooted in people and process, not technology. The fundamental challenge is in changing their behaviors, be it in how the organization prioritizes Data Quality via incentives, or in convincing a team to use someone else’s definition of their KPI. Unfortunately, no one has yet developed software to manage that!