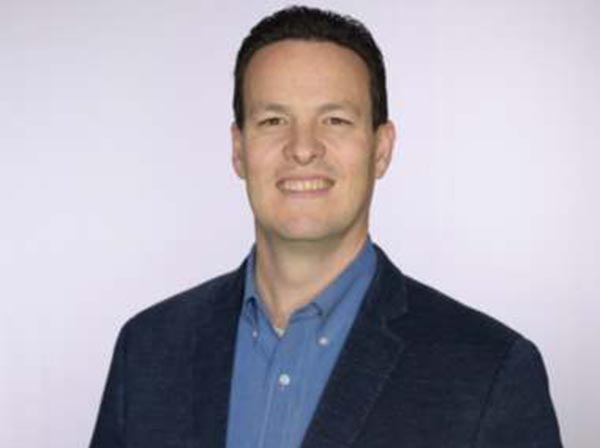
Click to learn more about author Scott Shadley.
While the development of autonomous vehicles (AVs) has been impacted by some PR problems and regulatory challenges over the past couple of years, the technology itself has continued to advance swiftly. As is often the case with any hot new category of tech, prognosticators overestimated how quickly AVs would reach mainstream adoption. But all credible research makes this clear: Autonomous vehicles will redefine mobility in the next decade and beyond.
RethinkX, a think tank that focuses on disruptive next-generation technology, published an in-depth analysis and estimated autonomous vehicles will account for 95 percent of U.S. passenger miles traveled by 2030. Meanwhile, Allied Market Research forecasts the worldwide AV market will grow from $54.23 billion in 2019 to $556.67 billion in 2026, a CAGR of 39.47 percent during that period. Regardless of which way the market heads, there’s one major infrastructure challenge automakers face as they look to ramp up AV testing and prepare for consumer and government adoption in the coming years.
Autonomous vehicles are a perfect illustration of edge computing in action. AVs collect massive amounts of real-time sensor data, some of which need to be analyzed immediately so the vehicle can make critical decisions within fractions of a second. Consider a self-driving car that encounters an object in the road. Is this object a person? — in which case the car should stop or change lanes — or is it merely a small piece of garbage? — in which case the car can drive over it without harm. On top of this, the amount of data generated and processed increases exponentially when there is more traffic or in bad weather conditions.
Since these decisions must be made in real-time, the data can’t be sent to the cloud for analysis and then sent back to the car. Moving data over such a distance would be far too slow where milliseconds in latency makes a significant difference in reliability and safety. Self-driving cars today can generate anywhere from 10 to 40 TB per day. Although a 5G network may be fast enough to move data back-and-forth in less than one millisecond, moving this much data overall is costly and inefficient. A better option is to analyze the data locally in the car itself. This requires a platform that can rapidly process data right where it’s created.
Computational storage is a unique technology that addresses this problem head-on. Computational storage minimizes data movement and supports edge computing in ways traditional approaches to compute and storage cannot. In-situ processing is the key to computational storage. It creates data processing capabilities within storage devices, like NVMe SSDs, eliminating the need for most data movement. In-situ processing brings compute capabilities right to where the data resides rather than keeping the two separate in legacy platforms. As a result, data collected by AV sensors can be analyzed immediately, and important safety decisions can be made on the spot. Without these capabilities, AV infrastructure would never be efficient enough to support the mainstream adoption of the technology.
Some automakers have tried to mitigate the challenge of data movement by leveraging GPU and FGPA accelerators. GPU and FPGAs do add speed to the equation, but there’s not enough room, physical space, or power envelope for them in autonomous vehicles. Space and power are very limited in these environments. There simply isn’t enough room in a vehicle, especially a self-driving car, to house the traditional compute and storage infrastructure needed for real-time analytics at such scale in addition to extra GPUs and FPGAs. On the other hand, by bringing compute and storage together, computational storage supports much smaller form factors that can deliver the required capacity and processing power while easily fitting into AV platforms. Computational storage delivered in the M.2 SSD form factor, in particular, is gaining momentum for use in self-driving car platforms.
Another hurdle for AV infrastructure is power consumption. Autonomous vehicles will all be electric or hybrid, so power consumption is a critical concern. Again, the key is data movement. Moving large volumes of data long distances for processing eats up a lot of power. Eliminating most data movement significantly reduces power consumption. However, even when AVs bypass data movement and use the main CPU to perform real-time, localized analytics, it’s possible that data processing can still cause too much drain on the battery. This can be mitigated by as much as 30 percent today with doing the compute in parallel in computational storage drives, allowing manufacturers to extend driving distance while achieving the needed analytics.
Computational storage can be very seamless to deploy. It does not require a ground-up approach and instead can be implemented by minimizing modifications to existing AV platforms. In essence, the concept is to take a host-driven and memory-limited application and execute that workload in each device installed on the storage bus. The ability to move compute into storage, where the data resides, saves host CPU and memory from the traditional round-robin (data from storage into memory, analyze, dump, repeat) Data Management. Instead, the host CPU simply must aggregate the results from all the parallel paths.
Autonomous vehicles do not just include self-driving cars. Shuttles, buses, trains, and other vehicles are also a critical part of the equation. And the infrastructure for these vehicles faces the same challenges that are seen in cars — the need to limit data movement, accommodate size constraints, and minimize power consumption. Computational storage provides the answer in each case. By solving these problems, this emerging approach to compute and storage mitigates the major bottlenecks that have vexed autonomous vehicle manufacturers for some time. Computational storage will help make the widespread adoption of autonomous anything a reality.