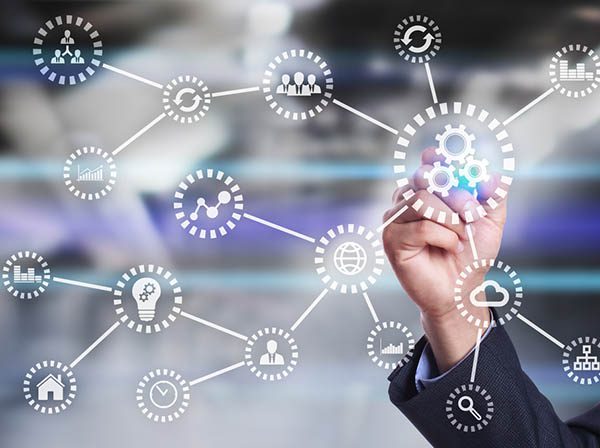
“Why?” a chief technology officer (CTO) may ask when the subject of automated Data Management tools arises. After all, their organization has probably already been storing, archiving, and backing up enterprise data day after day with success.
For example, setting up a Database-as-a-Service (DBaaS) from a reputable cloud computing provider, with appropriate access to data, enough storage, suitable integration, and strong enough security should be reasonable and keep the business running. Having a self-configuring and self-tuning database does save time and money by automating technical Data Management tasks. But this represents only a sliver of what is possible. To miss the full potential of automated Data Management is to remain mired in time-consuming, menial, manual business tasks.
Business forms the core of why organizations do Data Management. Data Management ensures “an organization’s entire body of data is accurate, consistent, readily accessible, and properly secured.” While backing up and keeping data safe – with performance humming among different data systems – maintains the data already there, these activities alone do not address the suitability of that data content or data quality. When the data is not fit for consumption, data scientists and business analysts spend 80% of their time each day cleaning and preparing data. As a result, these analysts have less time to gain potential business insights.
It may not seem critical to automate redundant business Data Management work as the reports generate, analysis is executed, and the database systems remain active. But over some time, the combined slowness can be costly – and less able to handle dynamic and quickly changing data. Some firms realize this and are jumping on the automated Data Management bandwagon.
Gartner has predicted that 69% of routine work done by managers will be fully automated by 2024. Executives would benefit from learning how automated Data Management tools save time and money. These managers need to understand how automation does repetitive business Data Management chores, what tasks still need humans, and how to evaluate automated Data Management capabilities.
What Are Automated Data Management Tools?
Think of automated Data Management tools as mechanisms to streamline enterprise-wide Data Management tasks. They fall under a technical practice called robotic process automation (RPA), which speeds up handling business operations and reduces costs. In the context of Data Management, RPA handles tasks like “data extraction and cleaning via existing user interfaces,” as well as technical DBMS tasks such as backup and storage. Implementing RPA through automated Data Management tools allows businesses to make sense of lots of data by mechanizing redundant work and leaving high-level tasks to humans.
Automated Data Management tools simplify operations through machine learning (ML) and artificial intelligence (AI). Both ML and AI technologies have become quite sophisticated in identifying data patterns and adapting to business rules by matching data, detecting and correcting errors, and mapping different data elements. Because of this, automated Data Management tools do tasks like evaluating unique data points or self-correcting bad data, (e.g., merging duplicate records). Through ML and AI, which continue to advance, organizations can quickly increase their ability to manage dynamic data, as both technical and business Data Management tasks complete in real time.
Business Data Management work, when automated, leverages on-hand changing information better and includes the following:
- Data Quality: Profiling, cleaning, linking, and reconciling data with a master source, a standard for formatting master data or the data to do business. In addition to making corrections to the data, these types of algorithms ensure organizations keep to well-defined rules and have logs detailing the processes used to make the data consumable.
- Metadata Management: Metadata describes information about the data on hand and its context, crucial to finding needed information within millions of records. Automated metadata management platforms prioritize data and quickly identify records to remain private to comply with regulations. They also speed up tracking data assets during use, making Data Quality and Data Management that much easier.
- Master Data Management: Master data describes essential data about people, places, and things needed to do business. Standardization makes master data stable through a centralized reference point, validating that one person’s record is indeed unique. Automating Master Data Management keeps this kind of data consistent and trustworthy across systems and up to date.
- Data Integration: Many firms have been dealing with multiple database systems with different standards. The end-user, whether human or AI, needs this mishmash of data floating through the pipelines to be unified. Integration processes allow consistent data quality when a user recalls this information.
Automating these business Data Management components saves the manual labor needed to make the data usable, either at the beginning or end of the DBMS pipeline. Consequently, businesses reduce costs and optimize data scientists’ and analysts’ talents.
Know the Business Reasons for Automating Data Management
Automated routinized Data Management helps if business reasons have been made clear at the outset, and algorithmic strengths are known. Each automated Data Management platform has a different focus. For example, Talend excels at maintaining clean and reliable data upon integrating different DBMS, while Informatica specializes in Data Quality and Master Data Management. Other Data Management platforms work only with specific applications, such as Cloudingo does with Salesforce. Because of these differences, blindly choosing a platform without understanding the business needs and aligning these with a good data strategy is counterproductive.
Throwing together a bunch of automated Data Management tools of any kind without a Data Strategy risks cost overruns, and not just because each platform differs. Preparation must be done before using any automated Data Management tool, as IBM learned while trying to use Watson, its artificial intelligence platform, as a clinical diagnostic tool. Many of these initiatives languished due to poor strategizing, as found by the University of Texas’ MD Anderson Cancer Center. This institution used older data sets, not the information needed for Watson to learn to diagnose.
Arvind Krishna, IBM’s CEO, has said that 80% of the “work with an AI project is gathering and preparing data.” Data Management automation tools “cannot fix completely broken, incomplete, missing” or poorly managed data. Automated tools find that work just too complex. Feeding the wrong data to automated Data Management tools sets them up for failure. So, some prep and cleaning will be necessary to ensure an automated Data Management tool works with the correct data.
Evaluating Tools
As shown above, automated Data Management tools have different capabilities. Even the most sophisticated platforms reach limits running Data Management tasks, like defining how metadata from various data sources should be categorized. Is there a way to assess automated Data Management capabilities among different applications? Also, can we compare these to human ones, just as the automotive industry has done for the self-driving car?
The DMM Capability Maturity Model levels, used to evaluate enterprise-wide Data Management, show promise and apply to both automated tools and human performance.
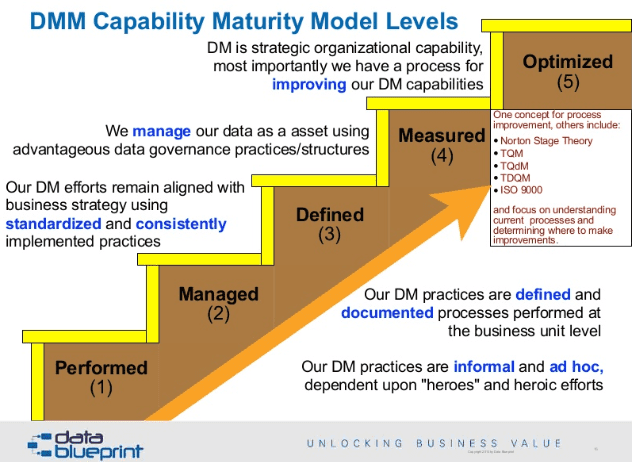
This schema emphasizes behavior and work products, regardless of where they originate.
For example, take data quality. Excellent data preparation and cleansing platforms can perform some business-level processes by matching alias to a master record. But this type of ability occurs between a level 2 and level 3 above. Such a platform cannot measure and review whether a database record is indeed an alias of a master record or a unique record. That has to be set by a human operator. This example demonstrates that the DMM Capability Maturity Model levels can help leaders see how software and employee data quality capabilities can be best leveraged.
Why consider a full range of Data Management tools? They automate not only technical Data Management tasks, such as backing up and optimizing DBMS, but also business Data Management tasks. Both kinds of automation are needed to manage dynamic data most effectively. Progress in artificial intelligence has made repetitive business Data Management less cumbersome and time-consuming.
With a robust Data Strategy and helpful automated management tools, organizations can better keep up with the marketplace and expand opportunities. While automated Data Management tools do not solve every Data Management business need, the tasks they do accomplish make a significant difference to the bottom line.