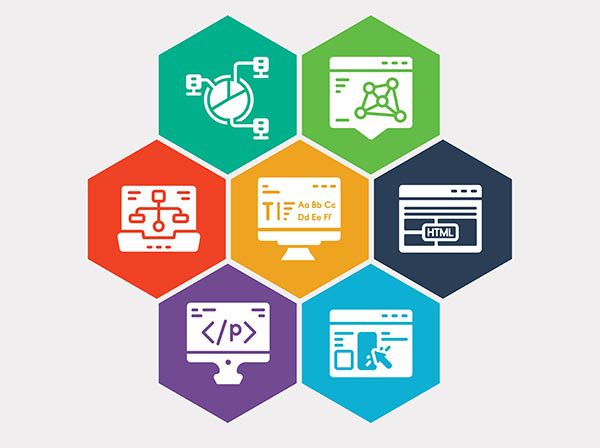
At the heart of things, an enterprise knowledge graph supports decision and process augmentation based on linked data. These models of knowledge domains are created by subject matter experts with the help of machine learning algorithms. They live as virtual data layers on top of existing databases or data sets to link both structured and unstructured data at scale and across disparate data silos. Introduce new data and the graph accepts it without issue, and uses it to grow data relationships that put more data into even greater context to better discover connections that can inform decision-making — faster.
In its Hype Cycle for Artificial Intelligence 2018, Gartner recognized the importance of knowledge graphs to the rise of AI. It explained that:
“The rising role of content and context for delivering insights with AI technologies, as well as recent knowledge graph offerings for AI applications, have pulled knowledge graphs to the surface.”
The knowledge graphs connection to AI comes from better embedding knowledge-driven processes into AI applications. But that shouldn’t be done in a scattered fashion, project-by-project. “Understand that having one particular problem solved with one AI app is a success but not a strategy,” said Andreas Blumauer, CEO and founder of Semantic Web Company.
The company, which provides graph-based metadata, search, and analytics solutions, was named as a sample vendor in Gartner’s report, and it also was ranked as one of the top 100 companies that matter in knowledge management for the 2019 KM World list. The list called the provider of the PoolParty Semantic Suite a leader in the graph-based knowledge technologies space and the most complete semantic AI middleware platform.
Jump into the Pool
The latest version of PoolParty for building and managing enterprise data knowledge graphs as the basis of an AI strategy now includes a graph editor and linked data orchestration to add to its coverage of the linked data life-cycle. Based on agile data integration, machine learning and text mining, or ontology-based data analysis, semantic AI should semantically enrich data so that classification results have a higher degree of precision: Entity extraction comes courtesy of machine learning algorithms with text mining methods based on semantic knowledge graphs.
And semantic knowledge graphs must be readable by both humans and machines from any app or database, Blumauer said, “It’s an asset on own.”
Having a full view of a huge amount of previously unlinked data assets via knowledge graphs to analyze enterprise-wide and heterogeneous data sets, or to link them with the help of artificial intelligence, can’t be underestimated in respect to delivering advanced data models that make data interpretable as well as reusable. “We can finally use a really large amount of data and make complex queries over it, validate different data sources at the same time and put them together,” said Blumauer. A knowledge graph, as he explains, is a multidimensional index for access to both structured and unstructured data.
Importantly, knowledge graph management isn’t something that’s limited to only data engineers or developers running SPARQL queries. “You can’t leave knowledge graph management as an exercise on the technical side,” he said; it also should apply to data scientists, process owners, and SMEs with appropriate governance in place.
Enterprises need governance models in place in order to establish a more collaborative knowledge graph environment where people can work with each other in a trusted way. “You can be more open [with a knowledge graph] but more closed, too. You don’t have to reveal to everyone all secrets within a knowledge graph, but single out one aspect and say whether it is publicly available or not.”
That’s helpful to industries such as banking where knowledge graph transparency isn’t exactly welcome. But they too need to be more transparent with the data and relationships that inform their knowledge graphs without interfering with things like compliance rules.
A W3 standard like SHACL, for validating RDF graphs against a set of conditions, can be used to make it possible for one group in an organization like a bank to work on the data part of a graph and another on business rules around datasets. “You can better execute compliance rules and at the same time control visibility,” Blumauer said.
Who Needs Semantic AI?
To date, the Semantic Web Company has seen its solution deployed in a number of industries Blumauer said — consumer goods, pharmaceutical and healthcare, e-commerce, engineering and construction, HR analytics, and more.
A use case in the field of HR is one where companies with traditional skill management systems are not dynamic. They can’t adapt quickly to the market demands in HR. That includes using semantic AI to learn from users’ work within projects to help with quickly understanding who is needed where and for what.
To address that, PoolParty employed NLP and text mining to dynamically match skills to profiles. This goes to a knowledge graph for skills management by linking together data objects to help with finding the appropriate people to take on certain tasks.
Another case is within the pharmaceuticals industry, where there is a large amount of structured and unstructured data. “Industry clinical trials produce a lot of structured data but also unstructured information in things like PowerPoint slides,” he said. “Then a year or so later the same people ask whether their company hasn’t already done some trials that deal with the same genes and proteins, but they can’t find it.”
One R&D department at a pharmaceutical firm found itself in that situation, doubling the amount of research in the same domain. With PoolParty Semanic Suite, it was able to transform a manually-edited database into an automatic tracking service with thousands of documents. The semantic AI tracking service now drastically improves R&D efficiency.
PoolParty Semantic Suite was also used in the development of the Noam Chomsky Knowledge Graph, the first knowledge graph for a public figure that should enter beta stage this year.
The challenges to adopting semantic AI and knowledge graphs in the not-so-distant past have often related to not understanding different use cases. Now, potential users have a variety of use cases to explore and can do so with a new case study booklet recently been published by the Semantic Web Company, so they can learn more about what knowledge graphs can do in their enterprise.
Image used under license from Shutterstock.com