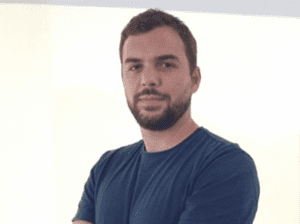
Building custom generative AI (GenAI) technology solutions is the best way to gain a competitive edge by leveraging GenAI tools and services tailored to your business.
On the other hand, building GenAI models from scratch is a costly and complicated endeavor – which is why many businesses instead settle for a genAI strategy wherein they buy off-the-shelf GenAI services that are easy to deploy, but that don’t deliver any unique competitive advantages.
Smart businesses, though, use a combination of both approaches. They buy GenAI solutions when it makes sense to do so, but they also build – or, at least, customize – some GenAI tools in ways that allow them to do things their competitors cannot.
To explain why a combination of building and buying is often the best foundation for a GenAI strategy, let’s explore the pros and cons of each approach, and how to use them both simultaneously.
GenAI and the Build vs. Buy Question
As with most novel technologies, businesses have two basic approaches available for implementing generative AI.
One is to build their own solutions. This generally means developing a generative AI model, supplying it with high-quality training data, performing training, and deploying the model. From there, the business is also responsible for monitoring and securing the model once it’s in operation. It may also need to update the model periodically by, for example, training it on additional data so that it can support a broader range of use cases. (As I explain below, there are other ways to build GenAI technology without actually creating a model totally from scratch, but they still require significant effort and expertise.)
The other approach is to buy a GenAI tool or service from a third-party vendor. This is what many companies are already doing as they integrate tools like Microsoft Copilot, Google Gemini, or OpenAI’s ChatGPT into their workflows. These solutions are easy to deploy because the models are pretrained and readily available.
The downside, though, is that they’re generic models that have no special insight into a particular business’s needs. Therefore, the models are typically not adept at supporting highly specialized use cases, such as generating content related to a proprietary product or helping create marketing content tailored to the business’s unique brand. Generic GenAI tools may help to accelerate workflows, but they don’t provide any advantages that are not also readily available to a business’s competitors.
Using off-the-shelf GenAI tools can also present some security and data privacy issues because it involves sharing your business’s data with the third-party organizations that own and manage the models. Although generative AI vendors are increasingly rolling out features to help protect sensitive data, there are no hard guarantees that models won’t “leak” proprietary business information to competitors or otherwise place private data at risk. This is especially true because AI models typically use client data for training, so even if no direct leakage of training data occurs, the model could still share a company’s internal knowledge with third parties.
Best of Both Worlds: Building and Buying GenAI at the Same Time
Clearly, both the build and the buy approaches to GenAI have distinct drawbacks, which can make it hard to opt for one or the other.
The good news is that businesses don’t have to choose just one strategy. They can opt to build some GenAI solutions while buying others.
The solutions that your business might choose to buy are relatively obvious. In general, it makes sense to use an existing, generic GenAI service to help support routine tasks, like writing emails or programming code. Chances are that your organization approaches these tasks in the basic way as almost every other company, which means that generic tools will support them well.
But when it comes to more complex use cases, or ones that are specific to your business, it may make more sense to adopt a build approach. This can entail creating, training, deploying and managing a custom foundation model entirely from scratch, as I noted above.
A Hybrid Approach: RAG and Fine-Tuning
It’s also possible to gain some of the benefits of a GenAI “build” strategy without developing a totally custom model. You can instead “buy” an existing model, then customize it through processes such as retrieval augmented generation (RAG) or fine-tuning.
RAG allows organizations to control the behavior of a pretrained AI model by controlling the types of responses the model can give to various queries. Using RAG, it’s possible to take a generic existing model that was not designed for any particular use cases, then tailor it to behave in particular ways that align with a company’s needs. For instance, a business could use RAG to help ensure that a chatbot based on a generic model responds to questions about the company’s products in certain ways.
Fine-tuning provides another way to customize the behavior of a pretrained model. Fine-tuning typically involves feeding a pretrained model additional data (such as information that helps it understand products or processes unique to your business) and/or modifying parameters that control how the model behaves. The extent to which models can be fine-tuned varies from one model to the next; open-source models are typically more flexible. But even proprietary models, such as those behind ChatGPT and Gemini, support some fine-tuning functionality.
The important thing to keep in mind, however, is that RAG and fine-tuning are both complex and intensive processes. They require less time and effort than building a model from scratch (and RAG in particular tends to require fewer resources to implement), but they still require deep expertise in how GenAI models work, as well as a well-designed data platform that supplies the right types of data – and data of sufficient quality and quantity – to enable effective model customization.
Otherwise, businesses can end up shooting in the dark by playing around with model data inputs or parameters, or attempting to feed in additional training data, without actually making the augmented or fine-tuned model any more effective for their needs than the generic model they started with.
Conclusion: Build and Buy, but Don’t Estimate the Challenge of Building
The bottom line is that the typical organization will be best served by a GenAI strategy that includes buying some solutions and building others. In many cases, organizations may opt to build and buy at the same time by fine-tuning an existing model.
However, it’s important not to underestimate the challenge of this process. Fine-tuning is a lot easier than building a model from the ground up, but it’s a lot harder than deploying an off-the-shelf solution that requires no customization. You’ll need deep expertise in both GenAI and data management at your disposal if you want to enjoy the best of both worlds.