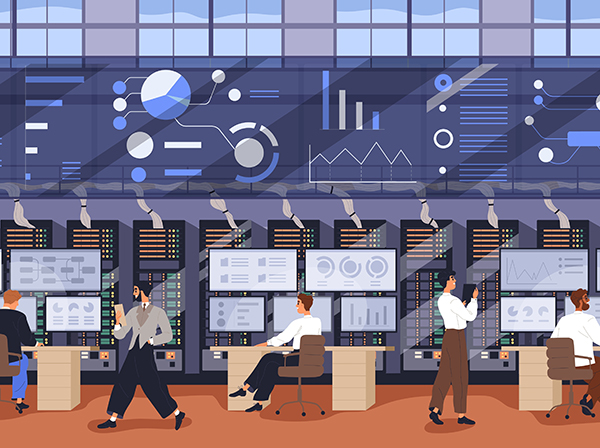
Data Governance applied to analytics, business intelligence (BI), or data modeling is nothing new, but Analytics Governance is somewhat different from Data Governance, says Malcolm Chisholm, president of Data Millenium.
Chisholm spoke at DATAVERSITY’s Enterprise Analytics Online, stating that Analytics Governance is focused within a more centralized unit rather than the distributed model Data Governance requires. “There is an enterprise-wide aspect of Analytics Governance, but it’s not quite as pronounced and fundamental, in the same way that Data Governance is,” he said, and because there are similarities, there is a tendency to unite them. Chisholm thinks Analytics Governance can learn some lessons from Data Governance, “but it’s still going to be its own thing in the end.”
The Emergence of Data Science and Analytics
Analytics Governance is an area that’s evolving. The historical timeline over which Analytics Governance has developed is being driven by technology advances, not just with modeling, he said, but also how data is managed, and the ability to store and process vast amounts of data through things like cloud infrastructure, along with more advanced networking and operating systems. Chisholm outlined the roles of Data Science disciplines covered by Analytics Governance: Business intelligence (BI) insights use existing data to explain something. Descriptive analytics explain what happened, and diagnostic analytics provide understanding about why it happened. Analytic insights help explore something that’s unknown for which there is no data yet. Predictive analytics provides theories about what will happen, and prescriptive analytics suggest how something could be made to happen.
Data Governance vs. Analytics Governance
Because data is found everywhere in the organization, much like people, Chisholm compared the management of a company’s data resources to the management of human resources. A human resources department sets the rules for how people are managed across the enterprise. Data is also everywhere in the organization, but historically it’s been managed like the “Wild West,” where everybody manages the way they want to, and this has caused problems. Similar to HR, the emerging horizontal function of Data Governance imposes a consistency, standardization and a distribution of accountability for managing data through policies, procedures, and data stewardship. “Data is enterprise-wide. It’s not really subordinate on something else.”
Drivers for Analytics Governance
Although there is a tendency to think that analytics consists solely of data scientists producing analytic models, the field of analytics is far more complex, he said, and there are innate drivers for governance:
Analytics Management
How is demand for models rationalized?
How is model development optimized?
Business Users
What should I expect when I ask for a model to be developed?
How do I integrate models into my business processes?
Can I trust models?
Executive Viewpoint
What risks exist in models and how is this being mitigated?
What value are we getting from models?
Data Scientist Viewpoint
How do I get the data I need?
How do I interact with my business sponsors?
Marketing Analytics Governance
Advanced analytics units usually exist to fill a demand generated from other areas of the business, such as forecasting for executive management, and modeling can address that, he said. Compared to Data Governance, there is an element of marketing that is required to be successful with Analytics Governance. Analytics units exist to fulfill demand that’s generated from other areas of the business so advanced analytics units must develop some kind of marketing strategy to market themselves to the rest of the enterprise, he said. The marketing strategy must be coordinated, particularly with the enterprise-wide aspects of Analytics Governance: “Otherwise you’re not going to really fulfill your task of bringing what analytics has to offer to the business to improve the bottom line, improve the top line, and reduce risk.”
Organization of Analytics Governance
Sponsorship
Analytics is specialized, so the sponsor must be the unit responsible for model development, usually an advanced analytics unit. That said, modeling may occur in many units within the organization, so the best sponsor is the unit most clearly identified with the analytics models tied to organizational strategy.
Role of Senior Management
Senior management should be involved from the start with model governance or Analytics Governance so they can acquire an understanding of modeling concepts. They will also need to know how they will interact with models in production and should acquire a level of model literacy.
- Organization of Analytics Governance: Although there is no universal operating model that will fit all situations, there are common elements and best practices that can be used to organize analytics
- Analytics Governance Committee: More oriented facing the business and should include senior management
- Analytics Technical Committee: Should focus on technical aspects of model development
- Specialized Working Groups: Should be developed for particular problems and issues that need to be addressed or solutions that need to be built
Dedicated Analytics Governance Analysts
Typically organizations will start by using volunteers who are required to add governance to their list of duties. “That was a lesson learned very early in the history of Data Governance that never worked,” said Chisholm. A successful program requires several dedicated analytics, governance, and analyst roles, all supporting Analytics Governance.
Communication and Model Literacy
As the use of models becomes more widespread throughout the enterprise, it will become more important for all members of the business to understand models, not just the IT staff or senior management. Leadership should address the following early on in an Analytics Governance communication program to increase the general model literacy of all staff:
- What are models?
- Why are they needed?
- How are they used?
- How to interact with them
- How to request a model
- How to participate in model development
- How to use a deployed model
This communication should be driven primarily by the Analytics Governance Committee, since it is business-facing, he says, but it’s also a good idea to get Corporate Communications involved, because they’re the experts on communicating new ideas across the enterprise and getting buy-in. In this case, it’s best to have a more standard “cookie-cutter” approach to education set up in advance rather than designing something individualized. Those who are actually going to interact with models will need a more specialized program of model literacy to be developed, focusing on how they interact with models.
Trusting Models
“Trusting models is going to be a problem,” he said, not for developers but for the business side. Common perceptions that arise when introducing analytics:
- It’s impossible for a model to accurately predict anything because models can’t predict hurricanes, or COVID, or where the stock market’s going tomorrow.
- Models could be a hidden mechanism of discrimination, opening us up to risk.
- Bad people are using AI to turn us all into zombies.
Communications shouldn’t try to deal with these overarching issues that models have in society today, but instead Chisholm suggests redirecting the focus to trusting models used in the context of the business. Trust in business models comes when leadership is transparent about how models are developed, how they’re deployed,
how risk is assessed in models, and how models are monitored to make sure that they’re not drifting.
The Analytics Life Cycle
- Request Intake: Define how the demand for analytics is managed, using a ticketing system or some other way of managing requests that come though the pipeline.
- Use Case Crystallization: Requests must go through an analyst who can get a minimum threshold of understanding of the request in detail, and who can assess whether or not a solution is feasible. These requests should be handled promptly and fairly.
- Model Prioritization: Rather than delegate prioritization to one person, this process is best handled by an Analytics Governance Committee. When decisions are made by a group, they are more likely to be perceived as fair. Chisholm warned against throwing up roadblocks such as endless forms or allowing requests to accumulate because they have to wait until the next meeting. It’s important to be consistent but also be flexible enough to reprioritize in response to emergencies, such as COVID.
- Third-Party Model Acquisition: Building a model in-house is not always the best option, and the Analytics Governance Committee should consider what steps are necessary for hiring a third party to provide models. In that case, the terms and conditions of a third-party contract should be carefully reviewed as some are quite technical.
- Developing the Model: The minimum viable data needed for the model should be determined: “This is a point where analytics, governance, and data governance meet.” Also to be determined is the minimum viable model – the minimum required to actually solve the use case, he said.
- Data Discovery: A development methodology should be initiated to determine if there is available data that will meet quality needs or whether outside data is needed.
- Model Validation: The validation process determines if the model will generalize adequately or if it is too specific to the data sets it was trained on. The business side can provide “sanity checks” on the results and help with the assumptions to be fed into the model. They can also help with obtaining data.
- Model Inventory: A model is added to the inventory when a modeling request is received and is updated at significant points throughout its lifecycle, such as validation, when a new version is created, etc. Chisholm considers the inventory a strategic requirement for Analytics Governance.
- Migration: “All of the technical aspects are super interesting to data scientists, but this aspect of making it successful in the business context is important too,” and failure at this late stage is still possible, he said. As the model is inserted into the business processes, roles and responsibilities must be clear. People in the business side really have to understand that it does entail some process re-engineering as well as model maintenance.
- Model Operation: Frontline workers using the information need a way to make a report if they think that things are going wrong from a business viewpoint. Because models can drift and lose accuracy over time, a monitoring process should be instituted as well.
Conclusion
Analytics Governance isn’t a one-size-fits-all venture, said Chisholm, and that’s why it’s important to define the lifecycle, then consider what needs to be done in each phase based on the unique needs of the enterprise.
Want to learn more about DATAVERSITY’s upcoming events? Check out our current lineup of online and face-to-face conferences here.

Here is the video of the Enterprise Analytics Online presentation:
Image used under license from Shutterstock.com