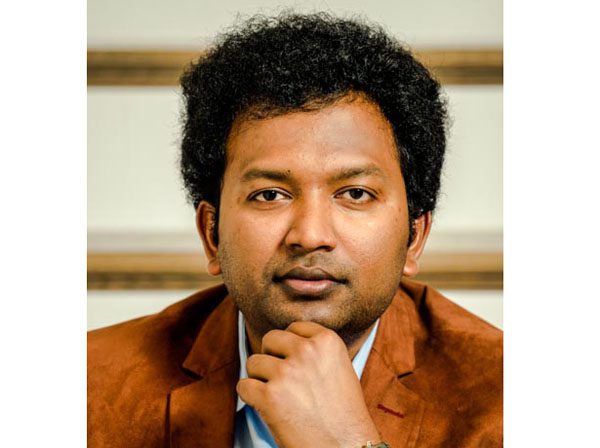
Click to learn more about author Sowmya Tejha Kandregula.
According to IDC, the size of the global datasphere is projected to reach 163 ZB by 2025, leading to the disparate data sources in legacy systems, new system deployments, and the creation of data lakes and data warehouses. Most organizations do not utilize the entirety of the data at their disposal for strategic and executive decision making.
Identifying, classifying, and analyzing data historically has relied on manual processes and therefore, in the current age consumes a lot of resources, with respect to time and monitory value. Defining metadata for the data owned by the organization is the first step in unleashing the organizational data’s maximum potential.
The numerous data types and data sources that are embedded in different systems and technologies over time are seldomly designed to work together. Thus, the applications or models used on multiple data types and data sources can potentially be compromised, rendering inaccurate analysis and conclusions.
Having consistency across the data is the only way to ensure that the conclusions reached upon by analysis are actionable and accurate regardless of the structure or location of data. In addition, the policies and processes designed to manage information and its metadata in defining and controlling the access to the data are critical for the protection of sensitive data.
Basically, metadata can be defined as data about data. It gets generated every time data is captured at a source, moved across an organizational structure, integrated with other data from other sources, profiled, cleansed, analyzed, or accessed by users. Metadata is valuable as it captures information about the attributes of data elements that can be used to guide strategic and operational decision-making. Typically, Metadata Management helps users:
- Discover and define data
- Accumulate and consolidate metadata from various Data Management repository into a single source
- Structure and deploy physical metadata to specific data models, business terms, and reusable design standards
- Analyze the data relating to the business and its metadata
- Identify where to integrate the data and track its trajectory and transformation
- Govern data by developing standards, policies, and best practices and associate them with data assets
- Empower stakeholders to manage and analyze data in one place and in the context of their roles
Metadata Management is the administration of data that describes the data within an organization, emphasizing associations and lineage. It involves establishing policies and ensures proper information management and maintenance. Metadata Management answers a lot of important questions about the data including:
- What data can we utilize?
- Where did it come from?
- Where is it now?
- Has it transformed since it was originally created or captured?
- Who owns the data and who is authorized to use it?
- Is it sensitive and what are the key risk indicators associated with the data?
- Is the data of any critical use to the organization and what quality constraints need to be applied to it?
Classification of Metadata
The nature and value of metadata can be viewed as complex, preventing organizations from realizing its importance. One major roadblock is the variation in perceptions of metadata within the same organization. This often leads to incomplete visibility and access into the data stream of the organization. A broad classification of the different types of metadata would provide a macro-view of the information that populates the organizational data universe.
Structured Metadata: This includes information about the organization of the data and is most often associated with structured and semi-structured data assets like databases, CSV Files, and XML Objects. Structured metadata documents what the data looks like, including data elements names mapped to columns, descriptions of data elements, the data types, the length of each data element, and the file layout. Structured metadata also includes tags, primary keys, foreign keys, as well as value domains for the data elements.
Supplier Metadata: Supplier metadata is information associated with the sources and providers of organizational data assets. It registers data’s origination point, directives, and constraints lacing the data prior to its use. It also defines the data owner, service level agreements with regards to the consumption of data, and whether there are any data consumption requirements. Supplier Metadata could also capture demographic information about the data asset like its size, number of records, date of production, and source of origin of the data asset.
Processing Metadata: Processing metadata describes the data production processes in the organization. It captures the data lineage which details the data hops within the organization, including the third-party sources of data, set of transformations applied to the data across the lineage, derivations of the data elements, and the process flows in terms of data pipelines.
Query Metadata: Query metadata entails information associated with the context and classification of the data asset and incorporates a business glossary listing business terms and their definitions. Importantly, query metadata also includes categories, classification taxonomies, reference data, and master data that can be used to build a semantic index searchable by a variety of terms. This type of metadata may incorporate historical usage data as well that tracks the types of queries data consumers have performed and how they selected and subsequently used selected data sets.
User Metadata: User metadata includes information about data consumers, the groups to which they belong, and the types of roles they play. In addition, actor metadata lists the data owners and data stewards tasked with overseeing the quality and usability of the data asset.
Governance Metadata: Governance metadata incorporates rules and policies for data retention and data quality, as well as the regulations used to implement data protections, manage access and use, and observe obligations assigned to the data set.
Metadata Management Basics
Although there are many definitions of Metadata Management, its core functionality is to enable a stakeholder to search and identify the key attributes of data assets in a data cataloging interface.
With a proper Metadata Management system in place, business users will be able to understand the source of the data attribute and the calculated measure of the attribute. They will also be able to visualize which enterprise systems in the organization the attribute is being used in (Lineage) and the impact (Impact Analysis) of modifying the attribute such as the length of the attribute to other systems.
Technical users have a need for Metadata Management as well. By mapping business metadata with technical metadata, a technical user will be able to find out which ETL job or database process is used to load data into the attribute. The end result of Metadata Management can be in the form of another ‘database’ of the metadata of key attributes of the company. The industry term for such a database would be called a Data Catalog, or a glossary or Data inventory.
Role of Metadata Management
A strong Data Management strategy enables the Data Quality rules for the business requirements, data cataloging (integration of data sets from various sources), mapping, versioning, business rules and glossaries maintenance, and Metadata Management (associations and lineage).
An accurate representation of the corporate metadata landscape mitigates friction in data accessibility and utility, improves overall information quality, and expedites digital transformation as more individuals from across the organization become adept at reporting and data analysis.
This can only be possible with Metadata Management tools that can record and identify data from different systems across the organization, including data at rest like databases, data warehouses, and data lakes and data in motion as it is integrated into applications.
The following capabilities are essential in creating a real-time data repository so that all stakeholders of the data assets can access the relevant data authorized for their usage and generate the desired outcomes:
- Reference Data Management for capturing and utilizing the shared reference data domains.
- Data Profiling for data assessment, metadata discovery, and validation.
- Data Quality Management for maintaining data integrity and assurance.
- Data mapping for capturing data flows and lineage and reconstructing data pipelines.
- Data Lineage to analyze impact analysis
- Data Cataloging to capture object metadata for identified data assets.
- Data discovery to help users understand the use of data across the sources.
Advantages to Metadata Management
Experience of an Enterprise Data Governance
With the exponential rise in data, Data Governance has become a necessary ongoing initiative that requires everyone including executives to redefine their responsibilities towards data and assume regulated levels of cooperation and accountability. With business stakeholders aligning Data Governance to strategic enterprise goals and technical stakeholders handling the technical aspects of Data Management, Metadata Management helps in finding, trusting, and utilizing data to effectively meet the objective within the stipulated timeframe and resources.
Attested Data Quality
With Metadata Management getting automated, Data Quality is assured as the data is regulated and operationalized to the benefit of all its stakeholders. Data inconsistencies and errors can be identified in real-time to improve the overall quality of the data by optimizing time to insights and repair. Based on the data types and its usage, quality rules can be set for the data to maintain its integrity.
Regulatory Compliance
Regulations such as the General Data Protection Regulation (GDPR), The California Consumer Privacy Act (CCPA), Health Insurance and Portability Accountability Act (HIPAA), and Basel Committee on Banking Supervision (BCBS) particularly affect sectors such as finance, retail, healthcare, and pharmaceutical/life sciences. If critical or sensitive data isn’t identified, defined, and standardized as per the regulatory norms, audits may be flawed. Such data is tagged, its lineage is documented, and its flows are depicted so that it can be easily identified and its workflows can be seamlessly traced.
Faster Speed to Insights and Quicker Project Delivery
Before the advent of Data Governance, data analysts and data scientists used to spend around 80 percent of their time identifying and understanding the source data and resolving errors and inconsistencies, rather than analyzing it for its worth. Automated enterprise Metadata Management provides greater accuracy and up to 70 percent acceleration in project delivery for data movement and/or deployment projects, harvest metadata from various data sources, and maps any data element from source to target and harmonize data integration across platforms. With Metadata Management and Data Governance structures in place, technical resources are open to focus on the highest-value projects while business analysts, data architects, ETL developers, testers, and project managers can collaborate more easily for faster decision-making.
Greater Productivity and Reduced Costs
Automated and generalized Metadata Management processes have resulted in greater and cost-effective productivity. The costs of data generation, migration, and consumption have reduced substantially along with the time to implement these processes.