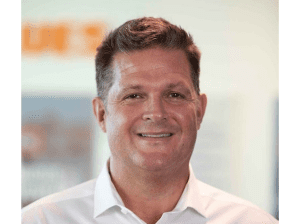
As artificial intelligence (AI) adoption surges, modern organizations face increasing pressure from all angles. Stakeholders demand the implementation of AI, media headlines hype its boundless potential, and employees are expected to leverage AI-driven efficiencies. Yet, despite the excitement, many companies struggle to realize the promised value of AI investments. The reason? There is a critical lack of guidance on how to overcome tech challenges and drive real value from these AI investments.
According to Gartner, by 2025, generative AI (GenAI) will be a workforce partner in 90% of companies worldwide. And yet, according to McKinsey, 70% of digital transformation projects fail to meet their stated goals, resulting in significant financial losses. As AI shifts to an enterprise initiative, organizations should take a deliberate and focused approach to implementing it.
Specifically, organizations must diligently avoid the common technical pitfalls that prevent AI projects from going into production. Addressing these issues early on, with a well-structured strategy and close collaboration between technical and business teams, can help ensure that AI initiatives move beyond the experimental stage and deliver real value in production.
Common Flaws Blocking AI Success
Despite AI’s potential, organizational and technical challenges can hinder the outcomes of AI projects. One of the most critical barriers is data readiness. Organizations frequently underestimate the importance of preparing data to meet AI’s demanding requirements. Without clean, well-structured, and properly governed data, AI algorithms can produce inaccurate or biased results, making investments ineffective and unproductive.
A common flaw is failing to thoroughly assess existing technology and data infrastructure before integrating AI. Attempting to embed advanced AI systems into outdated or incompatible environments can hinder efficiency and lead to missed opportunities for optimization. This oversight often results in fragmented projects and unnecessary spending – pitfalls that thorough planning and infrastructure alignment can prevent.
Another significant flaw lies in organizational preparedness. Successful AI integration requires skilled personnel, such as data scientists and AI specialists, who can develop and manage AI projects throughout their entirety. Yet, too often, companies lack these resources, leading to the mismanagement of AI initiatives and a failure to achieve long-term scalability. Additionally, many organizations turn to external partners to fill skill gaps in AI, but selecting the wrong partner can be costly. Some vendors may misrepresent their expertise, leading to poorly executed projects that don’t meet business goals. Vetting partners carefully for demonstrated capabilities and alignment with project needs is essential.
Additionally, decision-makers often need help deciding whether to develop AI solutions in-house, purchase pre-built platforms, or leverage open-source tools. Each approach has challenges, so it’s essential to make decisions that match core business objectives. Without clear guidance, the complexity of choosing the right path can leave teams stalled or pursuing AI initiatives that fail to align with their goals.
How to Overcome AI Challenges
Data preparation is the first step to ensure good outcomes. Data should be ready for consumption in AI models, machine learning, and LLMs. Making data AI-ready extends its lifecycle beyond that of traditional pipelines. Democratized access to data prepared for consumption, along with tools and training to help teams understand and use the data, is the foundation of AI readiness. Proper governance, which synchronizes AI outputs with organizational values, is equally important to ensure ethical and trustworthy results.
To scale and productize GenAI, teams must model data to be usable and consumable. Targeted outcomes and considerations include improved accuracy, minimized API calls, and mitigation of hallucinations. This modeling requires a concerted effort toward data labeling, data enrichment, and bias mitigation.
Next, companies must invest in their team to support AI adoption. Hiring or upskilling professionals with AI and data science expertise is essential for driving AI initiatives. These experts will play a pivotal role in designing AI systems, managing data pipelines, and ensuring the technology aligns with the overall strategy. Trusted partners can also help define and validate AI use cases, ensuring that AI is used effectively and scaled appropriately. Depending on the company’s goals, resources, and industry context, the trained team can provide insight into whether building, buying, or using open-source tools is the best option for their organization.
Rather than being distracted by AI’s limitless potential, companies should identify high-value use cases where AI can deliver the most meaningful results. It’s best to start with an AI prioritization assessment framework that quantifiably evaluates business outcomes and gains against build and runtime costs.
For example, with power demands soaring due to large-scale AI model training, a data center company turned to our company to address rising operational costs. Working together, the company leveraged AI to monitor and predict power consumption, identifying erratic usage patterns that allowed the company to negotiate power hedging and price protection plans with utility providers. The company anticipates this strategy will save hundreds of millions of dollars over time, offering critical cost stability amid increasing energy demands.
Similarly, a weather company with 17,500 weather stations and sensors across six continents partnered with our company to enhance its forecasting capabilities. By accelerating data collection and transformation, they prepared real-time weather data for AI-driven analysis, improving forecasts for lightning and severe storms. The upgraded service delivers more precise predictions to organizations like schools, airports, utilities, and government agencies, significantly elevating the company’s service quality and safety.
Strategic Scaling and Long-Term Success
AI implementation is not a one-time event; it evolves continuously as technology advances and business needs shift. Companies should maintain a flexible strategy, making necessary adjustments as they learn from AI implementations. Regularly revisiting and refining AI strategies ensures that AI investments remain aligned with long-term goals and continue to deliver value.
Organizations can cut through the AI hype and make intelligent, focused decisions by addressing these common tech flaws, preparing data, and building the right internal capabilities. Establishing a well-thought-out framework for AI adoption is the key to unlocking the full potential of generative AI, turning it from a buzzword into a practical tool for driving real value and innovation across industries.
While AI offers endless opportunities for innovation and efficiency, success depends on overcoming technical barriers and adopting a strategic, focused approach. Companies that prepare their data, invest in talent, and identify high-value use cases will be in a stronger position to unlock AI’s full potential. Organizations can harness AI to drive long-term, sustainable success in a rapidly evolving digital landscape by partnering with trusted experts and maintaining a flexible, scalable framework.