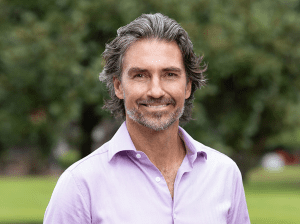
Generative AI (GenAI), specifically as it pertains to the public availability of large language models (LLMs), is a relatively new business tool, so it’s understandable that some might be skeptical of a technology that can generate professional documents or organize data instantly across multiple repositories. On the one hand, AI-powered data preparation sounds too good to be true. On the other hand, can an organization really trust the work that AI produces, and that AI will protect its sensitive data?
These doubts are valid – without proper data preparation, AI can produce inconsistent, unreliable results that do not mitigate risk or encourage trust. Unfortunately, hearing concerns about the potential risks of AI is all too common. However, there is an immediate and real-world solution to achieving responsible and reliable AI adoption: using AI to meticulously prepare your organizational data. Yes, I understand the irony of using AI to prepare your enterprise for a wider AI rollout, but there are AI-powered tools that can make the wider integration more successful.
In my experience, understanding, organizing and de-risking an organization’s data are the first steps in preparing it for other GenAI use cases. Before an organization can confidently leverage its data, it must know where this information resides across enterprise repositories, understand what the information is, be able to identify its business purpose, and identify any applicable access controls.
That’s where AI-powered data preparation capabilities come into play. Adopting AI early on in this process can deliver significant advantages, as AI can organize and de-risk an organization’s enterprise data at a scale that humans can’t.
Use Case 1: AI-Powered Data Preparation
The main challenges that organizations face with AI adoption involve poor data quality, which stems from a lack of data management. However, organizations can proactively work to employ AI to find, enrich, organize, and de-risk enterprise data, protecting it against possible vulnerabilities.
In these cases, AI can sort through an organization’s data, quickly and accurately de-risking and properly categorizing it. This includes finding the relevant information across repositories, organizing this data into a singular, normalized repository, applying data governance policies at scale, and feeding the enriched data into AI systems. In other words, it takes applying AI to intelligently prepare your data to use AI for other business objectives.
The now high-quality data can be used to either train or supplement AI models that the organization will employ, enabling swift progression from AI pilots to production. It also enables responsible AI deployment by identifying and protecting sensitive data and leveraging previously untapped value that was lost amidst unstructured data. This means your organization can feel secure in future AI initiatives because of the transparency, auditability, and accuracy stemming from adopting AI the right way.
Use Case 2: AI-Powered Document Generation
Once you have used AI to prepare a foundation of high-quality data, another valuable use case is to utilize it to automate and expedite the creation of documents critical to your business, like requests for proposals (RFP). Intelligent data management can distinguish between types of documents within a repository, identifying key information and metadata within a document and allowing GenAI to use this data to generate new documents with greater accuracy.
For example, a manager might spend hours cross-referencing past RFPs to produce a new one for a prospective client. GenAI, however, can almost instantaneously generate a new RFP in a specific service area based on the past RFPs within a repository.
By eliminating a lot of the time and heavy lifting involved in RFP production, staff can take on more strategic tasks for their teams, furthering the organization’s business goals and creating a competitive edge with greatly expedited time to information. Staff can still take time to review the AI-generated RFP to ensure human oversight, but as long as the data that the GenAI used is highly relevant and specific, the information in the RFP will be reliable and accurate.
Without prior data preparation and management, though, GenAI can produce documents full of hallucinations that result in inaccuracies and require additional attention from managing personnel. Scenarios like these deteriorate the trust between users and GenAI. However, AI-powered data preparation can prevent these discrepancies and produce high-quality, dependable results.
Use Case 3: AI-Powered Metadata Extraction
Another valuable use case for GenAI is utilizing it to expedite the time that it takes for staff to identify and extract important information from high volume, complex documents.
Using AI to enhance data search, classification, and in-depth analysis of important metadata entrenched in documents such as contracts, variations, and master agreements, for example, can help identify commercial risk, hidden information value, and opportunities in real time.
If there is a question or conflict between an organization and its client, instead of spending hours sifting through emails and reading through legal terms within the relevant contract, managers can leverage AI to extract key details that provide almost instant clarity so you can quickly find a resolution. This accelerates time to information, saves valuable resources, and reduces the risk of human error that might occur in identifying the discrepancy. The time saved can then be better spent on proactive, strategic tasks, furthering an organization’s competitive edge.
Conclusion
The bottom line is that AI’s most valuable first role is data preparation for further AI applications. It can sort through your data, categorize it, and de-risk it with incredible speed and accuracy. This crucial first step is vital, as it prepares your data for other AI use cases in a safer, faster, and smarter way. Taking this approach will drive long-term value, competitive advantage, and a greater chance of AI success.