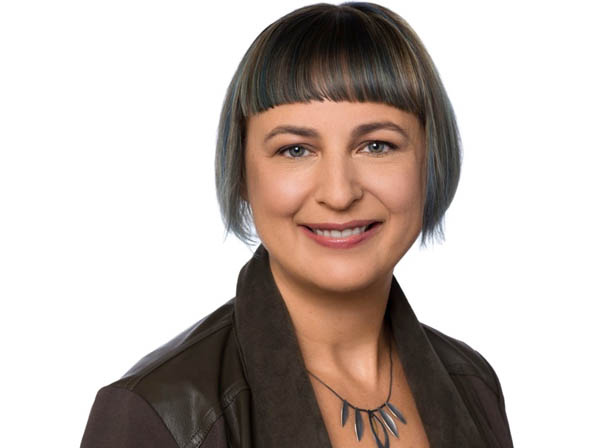
Click to learn more about author Karin Golde.
AI’s benefits have been touted broadly for years, but it has taken a bit longer for AI to reach the insurance industry. Over the past two years, the implementation of AI systems has seen a sharp uptick, however, particularly when applied to claims operations. Organizations realize greater efficiencies and better outcomes, even within the early days of adoption.
Now we have a baseline for what AI can do for carriers, and teams are getting more comfortable with the technology. Yet a number of needs remain if an organization wants to get the most out of its AI investments. Here’s a look at the ways I see AI advancing to further enhance claims operations.
Identity Management: Addressing the Need for Clean Data
We all want accurate predictions from our AI systems. Predictive capabilities represent much of the promise of AI after all. But, to get the best predictions, systems have to process accurate data. A key component is matching the right providers with the right claims, and that is itself a complex task.
Many factors can lead to the same provider being referenced multiple ways across different records. A provider’s name may be abbreviated or misspelled; they could move to another state, add specialties, or change practices. To ensure that AI systems make the correct matches and that the right provider accompanies a claim throughout its life cycle, identity management software is essential.
With advancements in software and API integrations, this is getting faster and easier to do. Some companies are even building their own identity management solutions. As the year progresses, expect to see identity management prioritized and prediction accuracy improve as a result.
Continuous Monitoring: Eliminating Bias for Better Datasets
Early on in a carrier’s adoption of an AI system, there was a bit of a “set it and forget it” mindset, whether it came from a third-party vendor or was built in-house. The model is done, it’s working great, let’s go. But over time, bias tends to creep into systems.
COVID-19 demonstrated the need for continuous monitoring processes; the environment changed dramatically in a short time, causing a shift in the nature and volume of claims. There was no way an AI system would not be impacted. With continuous monitoring, however, misleading signals can be spotted and addressed quickly.
Monitoring will continue to become more sophisticated and flexible in the days to come, particularly given new tools that enable data scientists to look for bias not only in the earliest stages of development but also in production environments. Changes in results can then be verified with domain experts – claims adjusters – and models adjusted accordingly.
Unstructured Data: Providing Deeper Insights
As powerful as AI is in claims operations today, we have just scratched the surface of what is possible. Data sources used in models to date represent only a small portion of data relevant to a claim. What about things like physicians’ notes, images such as X-rays or MRIs, or voice recordings? What about sentiment analysis that can shed light on what a claimant is thinking or feeling, revealing hints about the likelihood he or she will engage an attorney?
Fortunately, technology is advancing at such a rapid pace that these pieces of unstructured data are now being integrated into AI systems. Models are able to read and interpret them, which will make predictions richer, more accurate, and more useful than ever before. This is tremendously exciting and a massive time saver when you consider tasks like MSA submissions. Analysis of unstructured data sources will continue to improve over time as more data enters systems and machines learn from each claim.
Sharing Data: Entering a New Frontier
Each of the advancements above is deeply significant in increasing the value of AI in claims operations. Still, perhaps the most significant advancement is the ability to share data across the industry. This idea is a bit controversial, given that carriers are not wired to share information with their customers. But I’d like to lay the controversy to rest.
Cross-industry data sharing benefits everyone who wants to move forward with AI. To have a strong, accurate predictive system, companies need to draw from a wealth of sources. Imagine the difference if your system could draw relevant insights from 10,000 or 100,000 claims instead of 1,000. Think about how pooled information could help identify the best attorney for a claim when you’re looking across an exponentially larger body of work. The benefits are enormous. In fact, according to my company’s internal research, the accuracy of predictions jumped to greater than 90% when organizations shared data.
This does not mean tapping into another carrier’s system or sharing proprietary information. What I am talking about is the ability to leverage data that has been de-identified, anonymized, and segregated. It is housed and shared via a highly secure data lake that an AI vendor has access to so that when a carrier customer’s query is made about which provider in Omaha has the best outcomes with ankle reconstructions on female patients over the age of 40, the system can make recommendations with a much higher degree of certainty. This approach is incredibly useful for particular claims where the carrier has a limited sample to draw upon.
As I hope you can see, advances in AI are coming fast and furiously, and they will make a significant impact on the insurance industry. While we should not walk forward blindly, accepting the promise of any new solution, we should be ready to embrace change. The upside is simply too great if an organization hopes to get the most out of its AI system.