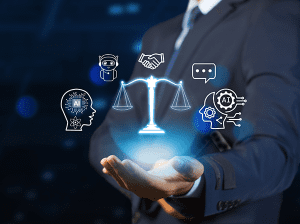
As AI adoption skyrockets, how can organizations harness its power responsibly? AI promises enhanced decision-making and customer interactions, but it comes with ethical, compliance, and infrastructure risks. Consequently, AI governance is crucial to address these risks.
In response, many businesses turn to their established data governance (DG), aiming to leverage existing controls and procedures. However, AI introduces complexities beyond traditional data management, necessitating an evolved approach.
Curtis Mischler, VP and Chief Data Officer (CDO) at Roosevelt Innovations, LLC, offers valuable insights on developing such an adapted approach. At a recent Data Governance & Information Quality (DGIQ) Conference, he explained how he integrated AI governance in Delta Dental of Michigan’s (DDMI) existing structures. He detailed the development of a tailored AI approval process.
He demonstrated how and why AI initiatives, built from DG, need to remain business-driven and aligned with organizational values. This article explores his experiences, starting at the beginning of the data governance program.
Assessing the Current Governance Landscape
DDMI started its data governance program in 2016. Mischler said, “We made a big investment. We knew building good data practices from the start would make a difference.“
At its core, Mischler’s data governance provides value through strong guiding principles.
Five guardrails support Mischler’s business strategy:
- Balance short- and long-term business needs: Mischler acknowledged practical business realities while building a long-term foundation. He said, “We did not want to wait for a ready data governance until dealing with the pressing business problems of the day.”
- Crawl, walk, run: “The process can feel slow. But continuous momentum is important,” he advised.
- Business-driven: Data governance planning and activities must meet business needs, defined as:
- Revenue growth
- Cost reduction
- Security compliance
- Business decision-making
- Risk reduction
- Have fun: Mischler stated, “We do things to liven data governance up a bit. It can be a boring topic.”
- Create a program that pays for itself: Mischler tracks deliverables and inputs. He makes sure the senior executive team understands the results and speaks on behalf of the DG program.
Building on these principles, DDMI evolved them for AI governance. Mischler emphasized, “Anything we do has to be business-driven.”
Evolving Decision-Making Structures
To keep AI focused on business, Mischler needed to know who to involve and who would make decisions. DDMI used its functional data governance structure as a starting point.
“Wherever possible we used existing groups,” recalled Mischler. That included leveraging a wide range of existing expertise for decision-making.
He went on to explain the different decision-making roles and responsibilities.
- AI Workgroups: Mischler explained that AI Workgroups consist of teams directly involved with AI technology. They come and go as needed, depending on the project.
- DSRC: DDMI had set up a Data Science Review Committee (DSRC) to oversee knowledge from the data science team. Mischler noted that this data science group develops and uses much of the AI products.
- ARC/ARB: The existing Architectural Review Council/Board (ARC/ARB), ensures alignment with the architectural principles. These groups work in real time and secure the infrastructure.
- DGPC: The Data Governance & Protection Council combines the data governance program with an existing Privacy and Security Council. See the “Assessing the Current Governance Landscape” section for more details.
- CCC: The Corporate Compliance Committee (CCC) reports to the board of directors. Mischler said, “We wanted to make sure that we had an avenue for an AI perspective, that would actually go to the board if we needed it.”
- CA&LO: The CCC also reports to the Chief Administrative and Legal Officer (CA&LO). These executives support the decision-makers and enforce their policies.
Even with these existing decision-making structures, Mischler needed one more: human resources (HR). He added HR because AI-specific policies have “a big people impact.” With the governance leaders in place, Mischler could focus on developing the approval process.
Developing a Tailored Approval Process
DDMI saw two steps to consider for AI approval: the proposed use case and the product.
During the first step, DDMI evaluates the AI use case to see if it is sound and ethical. Also, the DRSC assesses limits on if/where/how data may be used and any compliance considerations.
“If the request passes the first stage, it goes to the ARC/ARB to review,” said Mischler.
These teams “hone and narrow down on” a tool.
As the DSRC and ARC/ARB contemplate how to respond to the request for AI, they communicate with each other frequently. So, they use a governance, risk, and compliance (GRC) tool to manage their AI initiatives.
This app ensures transparent and structured communication of issues and status, providing an audit trail. The tool is also used for licensing, and Mischler’s teams filter this data, conveying the relevant request status through SharePoint.
Implementing Guardrails to Judge AI Requests
Building on their established decision-making structures and approval process, DDMI recognized the need for specific guidelines to ensure responsible AI implementation.
These guardrails are:
- People First: A human is involved in all AI decisions.
- Ethical Use of Data and AI: Ethical impact is considered in any use of AI.
- Data Privacy: Member information is secured.
- Transparency and Explainability: Any AI usage is acknowledged and explained in the best way possible. This includes where the AI is used, and how.
- Legal and Regulatory Compliance: AI usage adheres to current and anticipated federal and state laws.
- Security Measures: The company implements audits, assessments, and data loss prevention measures.
- Accountability and Responsibility: Humans take responsibility and understand this mandate.
- Continuous Monitoring and Evaluation: DDMI gathers continuous feedback about AI usage and adjusts activities as needed.
- Training and Awareness: All users understand AI and its implications.
- Data Usage and Location: Whenever possible, DDMI protects data by using onshore and closed models.
With these guardrails in place, DDMI was ready to put its new AI governance framework to the test.
Applying the New AI Governance Framework
Mischler talked about how DDMI applied the various AI governance components and came to a decision. Here are two of his six examples:
Analyzing customer service calls to flag grievances: When considering this request, decision-makers expressed concern about exposing private data and inaccurate analyses due to AI hallucinations – incorrect information outputs. To mitigate these problems, DDMI built a closed AI model for the pilot. The AI operated in a controlled environment and was checked through existing QA processes.
The AI tool worked very well and caught grievances that would have been missed. The customer service team also reported a better experience.
Identifying data in forms entered through the robotic process automation (RPA): DDMI had licensed an RPA tool from an outside vendor to automate repetitive, rule-based tasks. That vendor thought the company needed its AI tool add-on. The AI decision-making teams were concerned about data leaving the company and ending up in the vendor’s cloud.
Mischler and his teams denied this request. So, DDMI continues to use the RPA tool without the AI extension. The company did see an opportunity to improve RPA efficiency and may investigate an internal solution.
These examples demonstrate how DDMI’s AI governance framework operates successfully.
Key Takeaways
Several critical insights emerge from DDMI’s implementation of AI governance structures:
- The organization clearly and formally communicates concerns and the benefits of each request.
- The important and relevant people are involved in the decision-making process.
- Each conclusion leads to either successful AI implementation or concrete learning with a path forward.
Mischler developed this successful AI governance program by applying continuous momentum to a solid foundation:
- Assessing existing data governance at DDMI for guidance and what was working
- Keeping the existing decision-making roles, processes, and technologies in place with good outcomes
- Evolving this framework and formalizing who would oversee and collaborate with the AI approval process
- Keeping existing decision-making roles, processes, and technologies when they had previously led to good outcomes
- Creating and implementing an approval process for AI usage based on the use case and tool
- Developing guardrails for decision-makers and AI work groups to use
- Trying out this new AI governance and seeing it work
Mischler says that DDMI gets one to two requests a week and is hitting a lack of resources. To address this problem, he continually adapts governance to keep pace with the marketplace.
Where to Next
Mischler and DDMI are committed to ongoing progress, continuously addressing emerging business challenges and adapting their goals accordingly. He concentrates on smart AI usage from a business perspective.
He left with these parting words:
“We just want to keep crawling, walking, and running. We want to keep making progress and keep learning. And eventually, over time, I think we’ll hit the sweet spot in harnessing AI technologies.”
Want to learn more about DATAVERSITY’s upcoming events? Check out our current lineup of online and face-to-face conferences here.

Here is the video of the Data Governance & Information Quality Conference presentation: