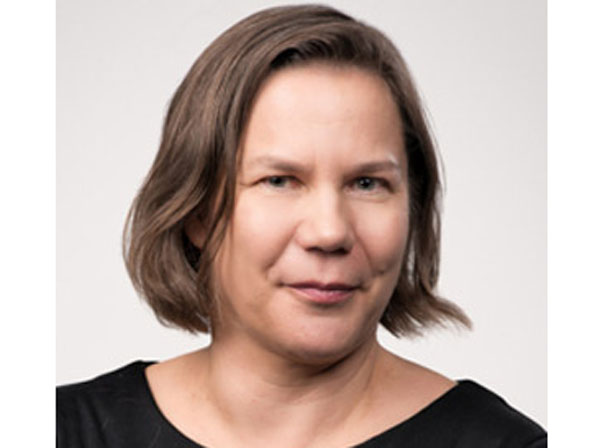
In my third article about the ethics of artificial intelligence (AI), I look at operationalizing AI ethics. Human intelligence remains a key factor – to keep a watchful eye on potential biases.
Amazon caused a stir in late 2018 with media reports that it had abandoned an AI-powered recruitment tool because it was biased against women. Conceived as a piece of in-house software that could sift through hundreds of CVs at lightspeed and accurately identify the best candidates for any open position, the application had acquired one bad habit: It had come to favor men over women for software developer jobs and other technical roles. It had learned from past data that more men applied for and held these positions, and it now misread male dominance in tech as a reflection of their superiority, not social imbalances.
Such anecdotes about stupid machines are grist to the mill of tech skeptics. If an AI system can’t properly vet a stack of CVs, how could we ever expect one to safely drive a car? But the AI ethics I’ve discussed in the previous two articles allows for a more constructive approach. What is the intent of using AI in recruitment? To make the hiring process quicker, while ensuring that every CV submitted gets a fair appraisal. How can this goal be achieved? By making sure AI recruitment isn’t marred by biases. So, there are risks, but there are also opportunities – and ethically founded, enforceable rules can make the opportunities prevail.
The ethics of intent and implementation and the ethics of risk and opportunity lead to the assessment that bringing AI to recruitment is a goal worth pursuing – when adequately policed by rules (and, yes, regulations). The theoretical framework elaborated in the previous articles is very useful in identifying ethically acceptable goals. But now we want to take ethics from theory and to practice – we want to put AI ethics into action. How do we operationalize AI ethics? How do we make sure good intentions aren’t undermined?
Beyond any Schadenfreude about Amazon’s 2018 brush with artificial stupidity, we must give the company full marks for spotting the problem and reacting to it. Whether by accident or design, it had people looking at the results of the AI recruitment software and asking whether they were plausible. They will have compared data coming out with data going in and, their suspicions piqued, they will have had a look at the mathematical model at the heart of the application. More men than women shortlisted, even though the application split was roughly equal? Goodness, the model is operating on false assumptions!
AI ethics in action requires humans to keep an eye on the data and the models central to the task in hand. Humans need to get a feel for the data in order to be able to determine when it’s no longer right. At that point, they need to look at the model driving the algorithm: Is it using proxies for variables that are discriminatory? Has the model been tested to make sure it treats all subgroups in the data fairly? Are the defined metrics really the best? Can the decisions of the model be explained in understandable terms? Have limitations to the model been communicated in ways they understand to the people who use it and rely on it?
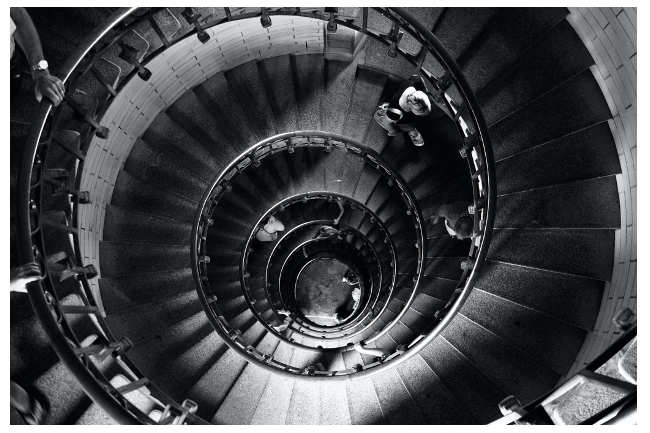
This is just one part of an ethics checklist that AI developers need to follow as a matter of course. Monitoring and evaluation are the final step that comes after the deployment of the AI application. If the data begins to look skewed, experts need to look at the model driving the AI, or they must go back to first principles and re-appraise the business model or function that is being automated. Putting AI ethics into action means treating AI like pilots treat their autopilots – they are very happy to use them, but there is always a human pilot on hand to take over if something seems amiss. AI should similarly never be left to its own devices – there should always be a human around to ensure the AI is functioning according to plan.
The test of whether the AI is doing its job is explainability. Any decision made by the algorithm should be explainable to the data scientist and the “end user” alike. The former will be happy to find out about the data used to train the model – its characteristics, distributions, biases – and how the model works (and where it stops working). The end user will be happy to discover the reason for any decision made by the model. What input was it based on and why did it make the choice it made? Why can we trust this result?
Explainable AI is based on the premise that the data points for every AI decision can be identified and explained at any given time. It takes the black box that was AI for so long and makes it transparent – such an understanding is the precondition for trust. Data scientists, domain experts, end users, politicians, regulators, and consumers are all demanding AI that can stand by its decisions because each decision can be explained. This new and evolving path to explainable AI is ethics in action. In the future, AI recruitment will be accountable recruitment.