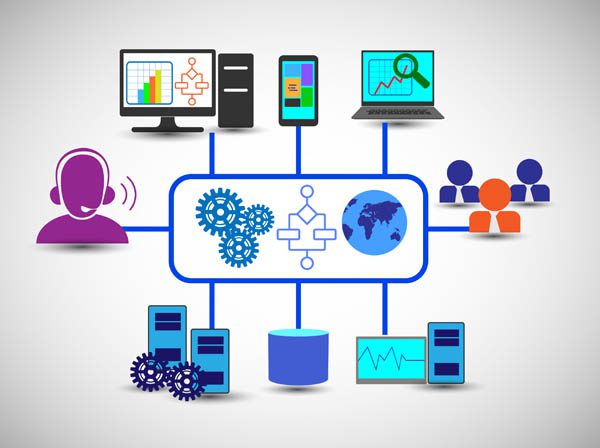
The purpose of a Master Data Management program is to organize an organizations most important data assets, allowing them to be used efficiently and creatively. The choice of organizational styles determines how the data performs during an analysis, when building new relationships, and while making decisions that increase profits.
There are key factors that should be taken into account when researching effective Master Data Management (MDM) strategies for 2020 and beyond. By taking the time to reconsider and revamp the business’s MDM strategy, data collection and delivery times can be streamlined, and management can make changes that previously only the IT staff could do.
Doug Kimball, the VP of Global Solution Strategy at Stibo Systems stated:
“The role of Master Data Management (MDM) has never been more critical and strategic to the overall success of today’s business.”
Master Data Management can no longer be considered a product, or a simple piece of software. MDM has evolved from a simple organizational tool into something that should be thought of as a long-term project that requires constant refining and adjustment.
Modern MDM ensures customer data is always clean, complete, and reliable. To create a fully functional MDM program, businesses are now using multiple technologies, including:
- Transform and load (ETL) tools
- Data matching tools
- Data Quality tools
- Real-time synchronization tools
The Multi-Domain Competitive Edge
Gartner has determined a large number of businesses are switching to multi-domain Master Data Management strategies. These strategies can help to resolve problems more quickly, discover new relationships, and develop new growth opportunities. Multi-domain solutions have the potential to speed up the time-to-market process as new products are connected with customers and suppliers in more efficient ways.
Historically, the selection of MDM strategies was focused on specific business requirements. The most popular solution was “one” data domain that performed a single business process, such as customer data or product data. However, a single-domain MDM solution can no longer handle today’s data-driven challenges. Data has continuously become more interconnected and interdependent. While a customer’s data would once have contained a simple demographic, or a transaction history, now it includes a customer’s preferences and buying habits. Other useful domains include digital assets, supplier data, hierarchies, and reference data.
Financial services, retail, and healthcare were the first industries to use multi-domain MDM solutions, primarily in response to compliance requirements, products, risk management, and information rationalization. The healthcare industry, for example, has begun implementing these strategies to simplify a physician’s job by linking a patient’s identity data with reference data, tying multiple IT systems into one single point of reference. The financial industry benefited from multi-domain MDM by augmenting data regarding their existing customer information. The retail industry uses it to improve the customer’s experience. Multi-domain MDM has allowed consumer packaged goods companies to develop a holistic view of their operations, boosting revenues and setting new standards for inventory management. (And multi-domain MDM is becoming less expensive).
MDM and Artificial Intelligence
Harshad Keskar predicts data lakes will become the most efficient means of storing data in the future, with the help of AI (artificial intelligence) and ML (machine learning). And it will all be coordinated with an MDM. Data lakes hold large amounts of raw, unstructured data, but their implementation can be a complicated process. For example, loading the data from a legacy system, without any sort transformation, into a system such as Hadoop, can periodically cause chaos. Using the right strategies in delivering and organizing the data can prevent the data lake from turning into a data swamp. Artificial intelligence and machine learning can be used to resolve this problem.
Artificial intelligence can also be used to coordinate the Data Governance and Metadata Management aspects of MDM. Historically, only larger businesses could initiate successful Data Governance programs. They could afford the technology and the manpower. However, AI and ML now make it possible for smaller businesses to benefit from Data Governance as well.
Metadata Management
The use of quality metadata within an MDM program is a necessity, but can be complicated to setup. This is because tool-specific metadata normally uses different and, very often, proprietary standards. The result is incompatibility problems and dramatic slowdowns, as the data is translated from one format to another. This kind of slowdown can have a devastating effect on project delivery timelines.
However, a company called Octopai has come up with a reasonable solution. Octopai offers a cross-platform with automated and centralized metadata management solutions. It allows data and analytics teams to research and discover shared metadata.
Octopai scans metadata, automatically gathering data from the ETL, reporting tools, and databases. The metadata is stored and processed in a central repository, while a smart engine uses hundreds of crawlers to search all metadata, and then quickly presents the results.
Octopai is designed to gather business intelligence, and handle Data Governance and data cataloging. According to the CEO/co-founder of Octopai, Amnon Drori:
“Metadata Management can be used to improve quality and minimize duplication at source systems. These systems and processes can help an organization streamline the discovery and documentation of data related to the data in other systems, such as definitions of customers, products, or other topics of interest.”
Integrating MDM with the Workflow
Many businesses must deal with complex supply chain issues that now require “near-real-time synchronization” of their partners and contractors. Both the “upstream” process (opening an account/client on-boarding) and the “downstream” process (which includes Data Quality issue resolution, data stewardship, and data generation) improve significantly after being integrated with MDM software. The integration of Master Data Management software with workflow technologies and a business rules engine can significantly improve the customer experience.
These are some of the expectations customers now have:
- Accuracy: Delivering the wrong product or the wrong size has the potential to permanently alienate a customer. There are few issues more damaging than delivering the wrong item to a customer. (This is an area where Data Quality and MDM intersect. MDM can definitely improve data accuracy).
- Consistency: While advertising is useful, customers like consistency, particularly when placing an order. This is, in part, so they can get through the process quickly and efficiently, but consistency also supports a sense of trust. Anything disrupting or slowing the process, such as advertising, gimmicks, or general confusion, will irritate the customer, and discourage future purchases. Improving Data Quality improves consistency.
- Business Intelligence: When customer data is incomplete or flawed, important trends can be missed. Stocking the wrong style of clothes or discontinuing a favorite product line will definitely have a negative impact on profits. MDM improves Data Quality, so this shouldn’t happen.
- Privacy: Customers, with good reason, are concerned about the accumulation and sale of their personal information. As a consequence, privacy laws have been enacted in some areas, ranging from the European Union’s General Data Protection Regulation (GDPR) to the California Consumer Privacy Act (CCPA).
MDM and Data Security
While the security of customer background data is certainly a concern, so is the business’s other confidential information. Security becomes an issue after an MDM integration process, as the data becomes accessible to a variety of staff across the entire business, including salespeople with laptops and smartphones.
A modern organization’s IT infrastructure now includes the traditional network — desktops, employee laptops, storage platforms, various kinds of operating software, and cloud storage.
Modern cybersecurity issues are more challenging than ever before. To achieve the goal of seamless end-to-end data security, the MDM applications must ensure compliance with the company’s regulatory and security policies. Determining who in the organization can access or change data is a good first step.
Image used under license from Shutterstock.com