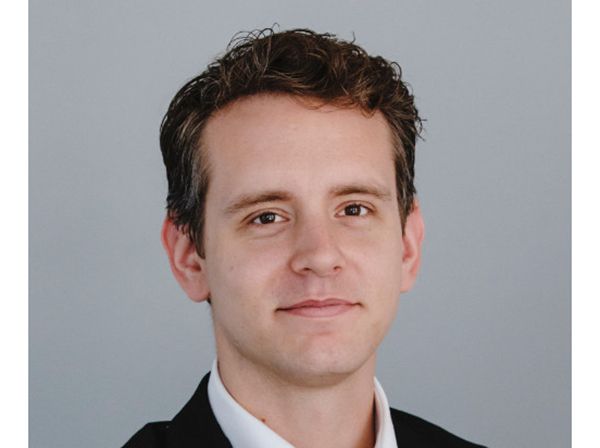
Artificial intelligence (AI) technologies like machine learning (ML) have changed how we handle and process data. However, AI adoption isn’t simple. Most companies utilize AI only for the tiniest fraction of their data because scaling AI is challenging. Typically, enterprises cannot harness the power of predictive analytics because they don’t have a fully mature data strategy.
To scale AI and ML, companies must have a robust information architecture that executes a company-wide data and predictive analytics strategy. This requires businesses to focus their data application beyond cost reduction and operations, for example. Fully embracing AI will require enterprises to make judgment calls and face challenges in assembling a modern information architecture that readies company data for predictive analytics.
A modern data warehouse is the catalyst for AI adoption and can accelerate a company’s data maturity journey. It’s a vital component of a unified data and AI platform: It collects and analyzes data to prepare the data for later stages in the AI lifecycle. Utilizing your modern data warehouse will propel your business past conventional Data Management problems and enable your business to transform digitally with AI innovations.
What is a Modern Data Warehouse?
On-premise or legacy data warehouses are not sufficient for a competitive business. Today’s market demands organizations to rely on massive amounts of data to best serve customers, optimize business operations, and increase their bottom lines. On-premise data warehouses are not designed to handle this volume, velocity, and variety of data and analytics.
If you want to remain competitive in the current landscape, your business must have a modern data warehouse built on the cloud. A modern data warehouse automates data ingestion and analysis, which closes the loop that connects data, insight, and analysis. It can run complex queries to be shared with AI technologies, supporting seamless ML and better predictive analytics. As a result, organizations can make smarter decisions because the modern data warehouse captures and makes sense of organizational data to deliver actionable insights company-wide.
How Do Modern Data Warehouses Work with Machine Learning?
A modern data warehouse operates at different levels to collect, organize, and analyze data to be utilized for artificial intelligence and machine learning. These are the key characteristics of a modern data warehouse:
Multi-Model Data Storage
Data is stored in the warehouse to optimize performance and integration for specific business data.
Data Virtualization
Data that is not stored in the data warehouse is accessed and analyzed at the source, which reduces complexity, risk of error, cost, and time in data analysis.
Mixed Workloads
This is a key feature of a modern data warehouse: Mixed workloads support real-time warehousing. Modern data warehouses can concurrently and continuously ingest data and run analytic workloads.
Hybrid Cloud Deployment
Enterprises choose hybrid cloud infrastructure to move workloads seamlessly between private and public clouds for optimal compliance, security, performance, and costs.
A modern data warehouse can collect and process the data to make the data easily shareable with other predictive analytics and ML tools. Moreover, these modern data warehouses offer built-in ML integrations, making it seamless to build, train, and deploy ML models.
What are the benefits of using machine learning in my modern data warehouse?
Modern data warehouses employ machine learning to adjust and adapt to new patterns quickly. This empowers data scientists and analysts to receive actionable insights and real-time information, so they can make data-driven decisions and improve business models throughout the company.
Let’s look at how this applies to the age-old question “How do I get more customers?” We’ll discuss two different approaches to answering this common business question.
The first methodology is the traditional approach: Develop a marketing strategy that appeals to a specific audience segment. Your business can determine the segment to target based on your customers’ buying intentions and your company’s strength in providing value. Coming to this conclusion requires asking inductive questions about the data:
- What is the demand curve?
- What product does our segment prefer?
- When do prospective customers buy our product?
- Where should we advertise to connect with our target audience?
There is no shortage of business intelligence tools and services designed to help your company answer these questions. This includes ad hoc querying, dashboards, and reporting tools.
The second approach utilizes machine learning within your data warehouse. With ML, you can harness your existing modern data warehouse to discover the inputs that impact your KPIs most. You simply have to feed information about your existing customers into a statistical model, then the algorithms will profile the characteristics that define an ideal customer. We can ask questions around specific inputs:
- How do we advertise to women with annual income between $100,000 and $200,000 who like to ski?
- What are the indicators of churn in our self-service customer base?
- What are frequently seen characteristics that will create a market segmentation?
ML builds models within your data warehouse to enable you to discover your ideal customer via your inputs. For example, you can describe your target customer to the computing model, and it will find potential customers that fall under that segment. Or, you can feed the computer data on your existing customers and have the machine learn the most important characteristics.
Conclusion
A modern data warehouse is essential for ingesting and analyzing data in our data-heavy world. AI and predictive analytics feed off more data to work effectively, making your modern data warehouse the ideal environment for the algorithms to run and enabling your enterprise to make intelligent decisions. Data science technologies like artificial intelligence and machine learning take it one step further and allow you to leverage the data to make smarter enterprise-wide decisions.