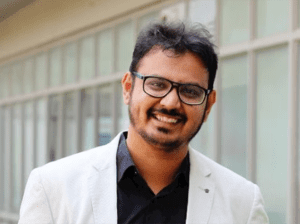
In today’s digital age, vast amounts of business data are gathered from different sources. Even when organizations strategically invest in analytics tools, they still face challenges in the form of data silos, unstructured data management, and failure of business-driven insights from tools.
To combat these challenges, more businesses are revamping with data strategy to seamlessly integrate AI and ML solutions to enhance their business operations. The AI adoption market is expected to rise to 1.8 trillion dollars by the end of 2030.
Dissecting the Term “Data Strategy” and Its Impact on AI Adoption
A data strategy is a long-term plan that includes technologies, tools, and processes, enabling an overall positive cultural shift. Businesses gather a large corpus of data that needs to be analyzed and managed effectively to generate relevant insights.
The concept of creating a comprehensive data strategy involves using relevant technologies and tools that align with long-term business objectives. Understanding what a data strategy is and its role in implementing successful AI business initiatives is important for profitable ROI metrics. A solid data strategy can accelerate AI adoption in the following ways:
- Spam detection
- Predicting future market trends or scenarios
- Improving customer experience and loyalty
- Drawing real-time insights
- Retrieving data as an actionable plan
- Enhancing security
- Fraud detection
- Facilitating new business model
- Effective data governance
- Data compliance and regulations
Major AI Adoption Challenges Faced by Enterprises
The stats reveal the bright future of artificial intelligence technologies and their use cases for enterprises across the globe. However, implementing AI successfully into business operations will require them to resolve certain data challenges. Also, partnering with a skilled AI developer to address any AI challenges while proactively resolving the complexities.
1. Data Availability
AI needs to be trained upon a large set of quality data to derive business insights in real time. However, inadequate, unprocessed, and unstructured data can often lead to inappropriate training of AI models. Furthermore, to enable accurate results from AI models, you need to implement data cleaning and preprocessing of them.
2. Data Quality Management
Gathering data from various sources like the web, social media platforms, and backend systems leads to inefficient data management, as feeding unstructured data from traditional tools to AI models leads to data silos. This may lower the data quality, making it less adequate for AI/ML model training.
3. Efficient Tools and Resources
Obtaining AI expertise plays a crucial role in executing data strategy. On the other end, the rigidity of traditional data management tools makes it challenging to integrate them with modern-day data analytics tools.
4. Data Privacy and Security
Efficient data governance is essential for organizations to adhere to compliance regulations. Businesses need to keep intact the privacy of any users. Simultaneously, lack of governance can lead to financial or reputational loss if businesses fall prey to data breach incidents or online fraud. Hence, incorporating of GDPR compliance can increase the overall complexity of your solutions.
5. Scalability Issues
Managing large amounts of data can be a highly tedious task. Moreover, old legacy systems cannot handle complex datasets while meeting the parameters of performance and security. Hence, opting for cloud infrastructure for your data strategy makes it scalable and easy to integrate with modern BI tools.
How to Adapt Your Data Strategy for Successful AI Adoption
1. Conduct a Thorough Data Assessment
Separate all data according to its source. Recognize whether the data is coming from a structured source (database) or an unstructured source (image and files). Analyze the nature of the data and work on refining and processing it further.
2. Define Data Alignment with Business Objectives
Define the business use cases to develop your AI application.
Try to ask relevant questions such as:
- What problems is your business trying to solve with AI?
- What opportunities can AI adoption bring to your business?
- Are you trying to develop an AI chatbot, whether for predictive analytics to reduce customer churn rate or extensive research on future trends?
Align the data that serves your AI use cases and your long-term business goals. Demonstrate how a particular set of data will serve your business vision and goals. Data alignment to embrace business will set the stage for successful AI adoption in 2024.
3. Optimize the Data Architecture
Data-driven architecture involves data storage format, data analysis, and data processing in real time. With increasing AI adoption for enterprise use cases, designing a robust architecture is a crucial factor in improving data quality. Design your data architecture as microservices to improve its usability and performance.
Implement real-time data integration to draw status insights about a business – for example, in the case of fraud detection, data breach incidents, or fault detection in real time. You can choose platforms like Apache Kafka, AWS Kinesis, Apache Storm, and multiple others to design an optimized architecture. Consider techniques like data warehousing to collect and process structured data to train AI/ML models. A data-driven architecture allows the workforce to adapt to certain market changes and enables responsiveness in real time.
4. Prioritize Quality with Data Governance
Any AI-based solution thrives on quality data. However, ensuring quality data management remains a top business priority for AI adoption.
- Data management tools are a consistent process that relies on filtering inappropriate data and using adequate data to support AI adoption. Regular data audits ensure that the data is of high quality while meeting the standardized needs. Tools like Python Panda, TensorFlow, Numpy, and Airflow help in cleaning, processing, and automating the data pipelines. These open-source tools have an array of pre-built functions that monitor the data and detect any errors or missing values.
- GDPR Compliance: Ensure that any data governance methods or processes do not violate any GDPR laws. Make sure that the data used for processing and training is not under the surveillance of any third-party tools.
5. Opt for Scalable Data Infrastructure
As the data continues to grow, businesses should choose a robust infrastructure that is easy to scale and optimized for performance. Cloud is a cost-efficient solution that ensures that your enterprise is future-ready for AI adoption.
- Opt for a secure cloud-based Infrastructure. Opting for robust cloud platforms like Microsoft Azure, AWS, and Google Cloud platforms GCP provides a secure infrastructure that eliminates any risks or data vulnerabilities. By partnering with the right cloud platforms, you ensure scalability, flexibility, and security for your AI enterprise solution.
- Integration with existing systems: Make sure you seamlessly integrate your databases on your cloud setup.
6. Prioritize Data Security
Any mishaps in the system’s security can lead to reputational and financial loss for your business. Hence, prioritizing security is a foundation that can ultimately make or break your data strategy.
- Encryption Techniques: Incorporate a layer of encryption techniques in the AI algorithms to protect it from threats. Discover privacy-protecting AI techniques to maximize the potential of your AI and its implications for your systems.
- Access Control: Limit access to authorized people in the organizations. To protect sensitive information, grant access to only the relevant workforce in your business. The strategic move will maintain the GDPR norm under the Data Privacy Act.
7. Metadata Management Practices
- Data Catalog: The data catalog is a modern-day data inventory that manages metadata in an easily discoverable and usable way. This makes it easy to identify the source of the data and ways to leverage it for AI adoption.
- Metadata management practices: By adhering to metadata management practices, you improve context awareness of the data in the inventory. By enabling understanding between the datasets, you can leverage the data for better and more timely decision-making.
8. Develop a Data-Driven Cultural Shift
Building a data-driven culture is not merely limited to data analytics tools, frameworks, and techniques. Of course, tools play a major role in leveraging data, but it is impossible without the active participation of individual professionals embracing the cultural shift.
- To facilitate successful AI adoption, you need to train a workforce with initiatives and programs that upskill their data literacy. Also, adequate training programs will allow them to leverage analytics tools and leverage their purpose for AI adoption.
- Cross-platform collaboration can enable the team to plan and strategize data management for AI initiatives. By fostering team collaboration, data scientists, data analysts, and project managers can contribute their ideas for a successful AI adoption into their operational flow.
By incorporating modern-day tools in your databases and optimizing the process, you create a data strategy that works in your favor.
The Future of AI Adoption: Trends and Practices
The future of AI adoption is promising and contributing to sustainability initiatives and energy optimization. Machine learning will continue to dominate, contributing to the growing AI market for businesses. However, training the ML model on a large corpus of text can increase the explainability of AI.
Furthermore, algorithmic bias and security vulnerabilities need to be actively addressed to leverage the tangible value of AI. Training the AI/ML models on a fair dataset and adding a layer of security can resolve the complexities involved in the overall process.
Conclusion
Data strategy is the foundational base for successful AI adoption. Designing a robust architecture, opting for scalability infrastructure, and following GDPR laws will allow you to scale your AI application. Moreover, it will allow you to actively address any threats and take proactive measures in real time.