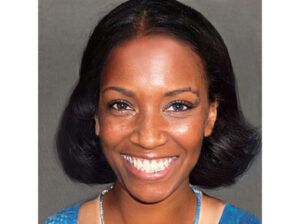
Data-driven decision-making is essential in all organizations, but using low-quality data can potentially be more harmful than using no data at all. Unreliable or outdated data can have huge negative consequences for even the best-laid plans, especially if you’re not aware there were issues with the data in the first place. That’s why data observability is so important: It’s a proactive approach to ensuring your data remains accurate, consistent, and trustworthy. But how does one achieve it? Read on to find out.
Understanding Data Observability
In general, data observability is when you comprehensively monitor the health and state of your data systems in real time. It goes much further than traditional monitoring, which often relies on rigid, pre-set thresholds to flag obvious failures. Instead, observability provides deeper insights into the inner workings of your data environment, allowing you to see the root causes of errors.
It does this by collecting and analyzing your telemetry data, like metrics, logs, and traces, to reveal the why behind any anomalies. For example, if a revenue dashboard suddenly shows a 50% drop, observability doesn’t just flag the error, it can show the actual cause, like a broken data pipeline, or an unexpected schema change.
The Pillars of a Robust Observability Framework
Data observability is effective because it’s built on several pillars that work together to provide a holistic view of your data ecosystem. When they work in concert, they provide the in-depth insights that let you know the actual quality of your data.
Freshness
Keeping your data fresh and up to date means you can make decisions that are more responsive and accurate to your current needs. Observability helps keep data fresh by setting up continuous checks that alert you and your teams when data hasn’t been refreshed at the regularly expected times.
It’s simple: Minimize the use of stale data, and you reduce the risk of basing decisions on outdated information.
Quality
For your data to maintain high quality, it needs to be verified and checked that it’s accurate, complete, and consistent. Data observability does this by identifying any issues such as missing values, duplicates, or unexpected anomalies.
These continuous quality checks help you and your teams correct these errors quickly, which will improve the reliability of your downstream analytics and reporting.
Volume
Inconsistent data volumes can be a strong indicator of problems with your data pipeline, which can impact the quality of your data. Sudden changes in your data volume, whether it’s a surge or a drop, are signs that you could be experiencing data loss or system errors.
Observability not only tracks these metrics, it can show you where the errors are coming from, allowing you to quickly isolate and resolve any discrepancies.
Schema
Your data schema controls the logic and structure of your data platforms, making it critical for keeping your data accessible and accurate. Even minor inconsistencies and unintended changes can cause far-reaching issues in your data processing and analysis.
Proper data schema observability ensures your schema’s structure and format remains consistent over time. It monitors any schema changes and flags anomalies, allowing you to catch issues early and avoid downstream errors.
Lineage
Data lineage provides a detailed map of how data flows from its source to its final destination. This traceability is invaluable for diagnosing issues, as it allows teams to pinpoint exactly where an error originated. With clear lineage information, the process of debugging and remediation becomes much more efficient.
For further reading on these pillars, see this detailed discussion on data observability best practices.
Enhancing Data Quality Through Observability
Implementing data observability transforms your data feed management from reactive troubleshooting into proactive quality assurance. The continuous monitoring of data streams allows you to detect and address issues before they cascade into larger problems. For example, real-time alerts enable your teams to quickly identify when a critical metric falls outside its normal range, allowing them to investigate and resolve the issue before it impacts your end users.
Observability can improve your overall data trust by providing transparency into the entire data lifecycle. When your stakeholders know your data quality is being rigorously maintained and that issues are promptly addressed, they can have greater confidence in that data. This trust is vital for making informed, data-driven decisions.
For a practical example, think about how integrating observability can improve your revenue reporting systems. If a disruption occurs in the data ingestion process, real-time monitoring quickly flags the issue, and lineage tracking pinpoints the source. As a result, your teams can fix the problem promptly, which minimizes downtime and ensures decision-makers always have access to accurate data.
Implementing a Data Observability Strategy
For your data observability strategy to be successful, you need both a technical framework and a supportive organizational culture. You need to develop an environment that values transparency, where data insights are shared across teams. This type of cultural shift ensures your data isn’t being siloed and is made accessible to everyone who needs to access it.
This doesn’t mean your data management becomes a free-for-all. You need to establish clear data management and governance policies. These policies set the standards for data quality, define key performance indicators (KPIs), and ensure you’re compliant with regulatory requirements. With a robust governance framework in place, your data observability processes are more likely to be effective and sustainable.
When it comes to tech and tools, the most important step is to integrate the right data extraction tools into your existing workflows. Tools that continuously assess data pipelines can trigger alerts when anomalies occur, while detailed logging provides the context needed to diagnose issues. Regular audits of these systems ensure that quality standards are met consistently, and ongoing training for data teams is essential to interpret observability metrics and act on them effectively.
For more details on developing a comprehensive observability strategy, consider reading this guide to implementing data observability.
Having the Right Toolkit at Hand
While there isn’t a one-size-fits-all solution, successful data observability often involves a combination of tools you can tailor to your specific needs. Most modern observability platforms integrate features such as automated anomaly detection, customizable dashboards, and data lineage tracking. These tools help reduce the manual overhead of monitoring complex data ecosystems and allow your teams to focus on proactive problem-solving.
You should set up robust alerting mechanisms that notify your teams as soon as issues are detected. Couple this with maintaining detailed logs that capture the full context of any data processing events, and you’re golden.
It’s also beneficial to adopt solutions that automatically document data lineage, thereby simplifying the task of tracing errors to their source. Once you fully streamline these processes, you should see significant cost savings and reduce the risk of prolonged downtime if issues do occur.
Conclusion
When you can ensure your data is fresh, accurate, and fully traceable, you can minimize downtime, enhance data quality, and build a foundation of trust across all your operations. Remember, embracing observability means transitioning from a reactive approach to data issues to a proactive model where problems are anticipated and resolved before they escalate.