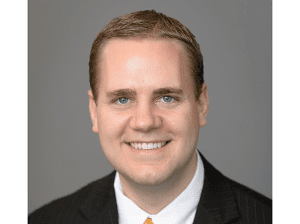
There’s nothing quite like gathering with data professionals – friends old and new – at a DATAVERSITY conference. Last month’s event was particularly special, combining the well-established Data Governance & Information Quality (DGIQ) East Conference with the inaugural AI Governance Conference (AIGov). Adding to the excitement was the expanded Women in Data Management and Governance (WDMG) program. With so much packed into the event, there was something for everyone, from newcomers to seasoned experts.
As DATAVERSITY founder and CEO Tony Shaw emphasized during the opening session, it is the culture of this data community that allows us to share critical insights, learn from industry leaders, and forge connections that support us in driving value within our organizations. Below are the key takeaways from this groundbreaking DGIQ East + AI Governance conference.
What Is AI Governance?
As is customary when data governance professionals gather together, aligning on some of the key definitions of the conference was of utmost importance. While foundational data governance terms were revisited for beginners in the field, the focus on curating clear definitions of AI and AI governance emerged as a key highlight. Tutorials, including Jimm Johnson’s session, unraveled the concentric circles of AI terminology. Below are definitions he shared from Andre Retterath:
- Artificial Intelligence (AI): Intelligence demonstrated by machines, as opposed to intelligence displayed by humans or by other animals. “Intelligence” encompasses the ability to learn and reason, to generalize, and to infer meaning.
- Machine Learning (ML): A branch of AI that focuses on the use of data and algorithms to imitate the way that humans learn, gradually improving its accuracy.
- Deep Learning (DL): Part of a broader family of ML methods, which summarizes algorithms that are structured in layers to create an “artificial neural network.” These algorithms can learn and make intelligent decisions on their own, just like the human brain.
- Generative AI (GenAI): A subset of DL models that can learn the patterns of their input training data and then generate high-quality text, images, and other content with similar characteristics.
- Generative Pre-Trained Transformers (GPT): A subset of GenAI models that leverage novel transformer architectures, which excel at capturing long-range dependencies and learning contextual relationships in sequences of data.
- Large Language Model (LLM): A GPT algorithm that can recognize, summarize, translate, predict, and generate text and other forms of content based on knowledge gained from massive datasets.
- GPT-4: The most recent GPT model version trained and provided by OpenAI. It’s the successor of GPT-3.5, GPT-3, and GPT-2.
- ChatGPT: A chatbot interface that allows users to interact with GPT-4 and other versions through natural language instead of code.

These technological advances have been among the fastest ever adopted with ChatGPT reaching 100 million users within just two months of launching. As AI’s adoption expands across both consumer and enterprise settings, organizations are tasked with implementing governance structures to ensure responsible use – hence the need for AI governance. The International Association of Privacy Professionals (IAPP) defines organizational AI governance as the internal guidelines and practices organizations follow to ensure responsible development, deployment, or use of AI by that organization.
The many facets of AI governance may include activities such as documenting third-party tools that have embedded AI that now penetrate and therefore expose risk to the organization. It also includes introducing and monitoring AI use cases within the organization by evaluating each use case’s value, effort, and risk. Then there’s managing the risk of the inputs, which primarily is considered data governance today; but now includes mitigating the risks of inappropriately training the models with inputs that lead to biases, hallucinations (better known as inaccuracies), and things like model drift. This leads to governing the algorithms and models themselves, with the help of AI experts. Lastly, there is the risk management of the outputs and how they affect things like copyright rules, commercial harm, and agency laws. For example, the outputs of AI agents have recently been upheld in court as truly acting on behalf of the company, even if it “hallucinates.”
The Current State of AI
Regardless of whether one believes that AI will eventually enable a single person to run a billion-dollar company one day or will soon hit the infamous “trough of disillusionment,” one thing is presently clear: 70-80% of companies are already using GenAI, as noted by Malcolm Hawker. Gartner has specified that the number one way companies consume GenAI today is simply through utilizing GenAI embedded in existing applications, such as Google Gemini, Microsoft 365 Copilot, Apple Intelligence, Adobe AI, or Zoom AI Companion. Other methods to fulfill Gen AI use cases include customized GenAI, training GenAI models, and using standalone GenAI tools.

AI Regulations
With AI projected to contribute $15.7 trillion to global GDP by 2030 (exceeding all of UK’s GDP in five years), discussions around regulations were front and center. Currently, there are about 35 AI-related regulations globally, with much more anticipated in the near future. The leading regulation currently is the EU AI Act with Article 5 starting to be enforced as soon as February 2025, likely setting a precedent similar to GDPR. In the United States, AI governance regulation is progressing through executive orders, state-level initiatives (e.g., California, Colorado, Maryland, and Illinois), and local measures like NYC 144. Industry-specific regulations are also anticipated, underscoring the need for organizations to stay ahead of compliance requirements.

AI Tools
With even more regulatory compliance for AI (and data) on the horizon, it’s also important to note the many tools and technologies available today for different capabilities of AI and AI governance. Sunil Soares categorized these into various vendor groups (in no particular order):
- Hyperscalers: Microsoft, Google Cloud, AWS, Meta, IBM
- Data Privacy: Dastra, DataGrail, OneTrust, TrustArc, Transcend, TrustWorks, Zendata
- Data Science: DataRobot, Dataiku, SAS
- Data Cloud: Snowflake, Databricks
- Data Governance & Catalog: Alation, Atlan, Collibra, Data.world, and Informatica
- AI Governance Focused: anch.AI, BreezeML, Credo AI, Enz.AI, Fairly AI, Fairnow, Holistic AI, Monitaur, Modulos AI, Prodago, QuantPi, Relyance AI, Saidot, Trustible, YOOI, 2021.AI
- Transparency & Explainability:
- Explainability: causaLens, LIME, parabole.ai, SHAP
- Content Provenance: Content Credentials, Google SynthID, Nightshade
- Fairness: Fairlearn
- FinOps for AI: Finout
- Conformity Assessments: AI Verify Foundation
- Data Labelling: CloudFactory, Innodata, Scale AI
- Governance, Risk, & Compliance: Archer, MetricStream, ServiceNow
- AI Development: Athina AI, BigML, Glean, HoneyHive, Humanloop, LatticeFlow AI, MLflow, neptune.ai, Patronus AI, PromptBase, PromptLayer, SilkFlo, Weights & Biases
- AI Observability: Arize AI, Arthur AI, Deeploy, Fiddler AI, WhyLabs
- AI Security & Privacy Enhancing Technologies (PETs):
- AI Security Posture Management: Protect AI, Palo Alto, Cranium AI, Securiti, BigID, Immuta
- Federated Learning: Curatio, Sherpa.ai, TensorOpera
- Red Teaming: Adversarial Robustness Toolbox (ART), Azure PyRIT
- Synthetic Data: Synthetic Data Vault (SDV), MOSTLY AI, Synthea
- Guardrails: Guardrails AI, Credal, Lakera AI, Robust Intelligence

Frameworks for AI Governance
As is helpful with data governance, there are existing frameworks that enable organizations to implement AI governance leveraging the expertise of industry practitioners, large organizations, and public entities. Sunil Soares provides a 13-component framework for AI governance in his free, downloadable book: AI Governance Comprehensive: Tools, Vendors, Controls, and Regulations. Nicole Janeway Bills and John O’Donovan also provided a comparison of seven prominent AI governance frameworks for enterprise use including those from Singapore, Microsoft, the Council of Europe, OECD, NIST, Google, and AWS. This can be found on their Data Strategy Professionals website.
Where Is the Value for Organizations and My Career?
The Use Cases for AI Governance
The time-saving efficiencies and valuable insights that AI can provide have been well known and documented for years now. Therefore, the greatest use case discussed for enabling such lower costs and higher revenues is the managing of the risk that AI presents to an enterprise – primarily in the form of avoiding fines from current and future regulations such as the EU AI Act, which have the potential to be greater than GDPR, per Sunil.
However, Sunil also added another business driver for AI governance. He has found that as companies are articulating the reasons why they should adopt AI in the enterprise, AI governance itself was actually found to be among the top three of those reasons. This is because with an AI governance program that is set up correctly within an organization, it is a competitive advantage for accelerating even more AI adoption, use cases, and therefore value for that organization.
The Use Cases for Utilizing GenAI for Data Governance
After understanding what data governance can do for AI in the form of AI governance, another discussion point was: What can AI do for data governance? Junaid Farooq shared a more comprehensive list of 40 specific GenAI use cases and the mapping of their relative value, effort, and risk. Some of the greatest use cases included: automatic data classification, duplicate detection, data profiling, reference data crosswalks, and text parsing for data ingestion.

Leveraging This Opportunity for Career Development
As the New York Times observed, “Many feared that artificial intelligence would kill jobs. But hospitals, insurance companies, and others are creating roles to navigate and harness the disruptive technology.” With the NYT claiming that the executive in charge of AI could in fact be the hottest job in corporate America, Sunil found that over 2,500 people already have the title of Chief AI Officer, per LinkedIn Sales Navigator (as of February 9, 2024). Lastly, in a panel discussion, William A. Tanenbaum observed that for lawyers, and Johna Till Johnson applied the statement for all employees: “It’s not that AI will replace your job; it’s that an employee that understands and leverages AI will replace an employee who doesn’t.”
With all of this being said, it was no wonder that nearly every presenter stated in their own way, and a simple summary of the greatest takeaway from conference was this: It is time for us as data governance professionals to rise up and seize this moment. Scott Taylor encouraged us to achieve “truth before meaning” by effectively telling our data story to our organizations, utilizing the vision and words of the business itself. Melissa Harrington encouraged us to be more authentic in our leadership, which research shows increases employee engagement (a $500 billion problem globally) by an astounding 76%! Irene Dirks illustrated creative ways using LEGOs to implement an engaging data governance program. Lastly, Malcolm Hawker encouraged us to become the heroes of the organization because our companies need us as data professionals to usher in AI-enabled business.
Conclusion
Mathias Vercauteren likened our current AI environment to driving cars in the early 20th century without seatbelts, guardrails, and speed limits. Laura Madsen astutely noted that although revolutions are realized as such historically, the two things we know about our time is that 1) we are definitely in the middle of an AI revolution and 2) although nobody knows what exactly will happen with AI in the future, we know disruption is on its way.
Data governance professionals already have the multi-domain skills needed to drive AI governance in organizations. Therefore, although this was the first introduction to AI governance for a majority of the attendees, it was encouraged by many to responsibly increase our utilization of AI, help general counsel by focusing on corporate policies, prepare the data for future AI use cases through effective data governance, become more familiar with existing and upcoming regulations, and – above all – become the leaders in this space and heroes within our organizations!
Want to learn more about DATAVERSITY’s upcoming events? Check out our current lineup of online and face-to-face conferences here.
