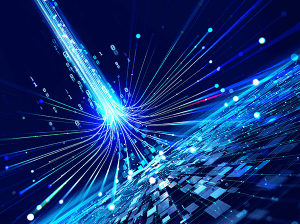
A modern data architecture is required to support the data-driven organization that every enterprise wants to be. Without a solid data architecture – composed of the models, policies, rules, and standards you set for how data is collected, stored, managed, and used – your ability to attain a holistic view of your business, make informed decisions, and quickly adjust to new developments can be compromised.
In a DATAVERSITY® webinar, Donna Burbank, managing director at Global Data Strategy, Ltd., shared survey insights about how defined data architectures help organizations. Benefits include better collaboration between IT and business teams; improved data quality, efficiency, IT productivity, and ROI; reduced operational costs; and increased speed to market.
As we approach the era of what McKinsey termed “data ubiquity” – where data will be embedded in systems, processes, channels, interactions, and decision points that drive automated actions – and as GenAI alters our data interactions across the board, it’s smart business to think about how you may need to adapt your data architecture to these new demands. Below are the data architecture trends that will shape the business landscape in the coming year.
Synergizing Data Mesh and Data Fabric
Businesses will increasingly align with the idea that they can – and perhaps should – implement and integrate data fabric and data mesh architectures, bringing together disconnected data sources to improve data governance, discoverability, and access with the former, and decentralizing data ownership with the latter so that teams can create and manage their own data as a product.
If you need further proof that the two architectural approaches can be harmonized to enhance your data infrastructure, consider this: Gartner has scheduled a session titled R.I.P. Data Fabric vs. Mesh Debate for its Data & Analytics Summit in March, which will discuss deploying the fabric design to unify data management and mesh operating model to distribute data management.
For many organizations it will make sense to embrace this hybrid approach in 2025 in order to unlock more value from their data. The question they’ll need to answer is how to accomplish the task.
DATAVERSITY advises that you start by understanding your business objectives and how a data strategy, with the overarching architectures, needs to support these goals. Your approach should be informed by an assessment of your data capabilities, use case candidates, available technical resources, and more.
“A data fabric actually supports building a data mesh because the fabric provides the underlying data management and integration framework that enables the data mesh’s core principles to function effectively,” explains Kaycee Lai, the CEO and founder of AI-powered data fabric vendor Promethium. “By leveraging both paradigms, organizations can build a comprehensive data infrastructure that promotes collaboration, data ownership, and efficient data sharing across domains.”
Retail grocery giant Kroger has already embraced the hybrid approach. To break down silos, improve data accessibility and quality, and enable more data-driven decision-making across the enterprise, Kroger deployed a data mesh supported by a data fabric and other technologies.
“Data mesh is really about how we organize and create decentralized teams within the business units. Data fabric is the connective tissue that allows us to interoperate,” said Nate Sylvester, VP of Architecture at 84.51°, a retail data science, insights, and media company owned by Kroger that is leading the effort.
Kroger’s implementation of the data mesh architecture reorganized teams and data around domains, such as supply chain, aligned to business capabilities. The data fabric provides standards and consistency for how domains interact and exchange data.
Overcoming Data Prep Hurdles for GenAI
A recent survey by Researchscape on the state of enterprise AI and modern data architecture found that the largest number of respondents (67%) have deployed generative AI over all other kinds of AI technology. According to a KPMG GenAI survey, 50% of leaders anticipate the greatest value creation from GenAI investments will come from enhancing existing products and services by analyzing customer data, enhancing efficiency to generate greater productivity (48%), improving product quality, efficiency, and innovation (42%), and improving supply chain efficiency and reducing cost (37%).
But there’s work to do in the new year for most companies to make significant progress toward meeting any of these goals. “Solving for data deficiencies has emerged as a crucial step in addressing the GenAI-specific demands of data architectures,” according to the Deloitte AI Institute.
To modernize their data-related capabilities, organizations are enhancing data security (54%), improving data quality practices (48%), and updating data governance frameworks and/or developing new data policies (45%).
GenAI lives on unstructured data – text, images, videos, audios – as the most abundant source for generating new insights. As pointed out by Rehan Jalil, CEO at Securiti, the sheer volume and variety of unstructured data makes it extremely difficult to govern, manage, and secure. “Enterprises are eager to harness the power of generative AI, but many underestimate the complexity of managing unstructured data,” he explained.
His advice to properly manage and effectively use unstructured data for GenAI projects is to:
- Discover, catalog, and classify unstructured data
- Preserve access entitlements of unstructured data
- Trace the lineage of unstructured data
- Curate unstructured data
- Sanitize unstructured data
- Focus on the quality of unstructured data
- Secure unstructured prompts and responses with pre-configured policies
Putting Generative AI to Work for Data Management Tasks
Boston Consulting Group has proposed that GenAI has a specific application to the challenges of data management that it itself increases thanks to the massive amounts of unstructured data required to train models. “Data governance is rarely the poster child for efficiency and effectiveness. For many companies, it’s a pain point, the work too manual and tedious – a real headache, especially in industries that are highly regulated or incorporate vast amounts of personally identifiable information,” BCG stated.
GenAI, it explains, can actually eliminate the manual and tedious work. “GenAI’s key traits – an affinity for unstructured data and an ability to create content – make it a natural tool for boosting the efficiency and effectiveness of data management.”
BCG posits six use cases for embedding GenAI in data governance and management practices:
- Creating metadata labels such as data source and applicable usage rights
- Annotating lineage information such as capturing and maintaining cross-system lineage data
- Augmenting data quality such as accelerating and automating key tasks including removing duplicate records and standardizing data formats
- Enhancing data cleansing such as synthesizing missing training data and removing data that is meaningless, corrupt, or otherwise unusable
- Managing policy compliance such as GenAI-powered knowledge bases, compliance checks, and action recommendations
- Anonymizing data such as transforming data that contains sensitive or personally identifiable information
“AI brings a whole new set of challenges such as fairness, transparency, and AI ethics, and the need to comply with emerging new AI regulations. To address these challenges, DG frameworks must rapidly evolve to support both traditional AI and GenAI,” said Dharma Kuthanur, GTM Advisor at Dataworkz.
Investing in the Enterprise Data Lakehouse
While unstructured data makes up the lion’s share of data in most companies (typically about 80%), structured data does its part to bulk up business’ storage needs. Sixty-four percent of organizations manage at least one petabyte of data, and 41% of organizations have at least 500 petabytes of data, according to the AI & Information Management Report.
By 2028, global data creation is projected to grow to more than 394 zettabytes – and clearly enterprises will have more than their fair share of that.
Time to open the door to the data lakehouse, which combines the capabilities of data lakes and data warehouses, simplifying data architecture and analytics with unified storage and processing of structured, unstructured, and semi-structured data.
“Businesses are increasingly investing in data lakehouses to stay competitive,” according to MarketResearch, which sees the market growing at a 22.9% CAGR to more than $66 billion by 2033.
“The increasing demand for real-time analytics and decision-making is propelling the data lakehouse market forward. Companies seek to leverage data to gain a competitive edge by making informed decisions quickly,” MarketResearch stated.
The flexibility of the data lakehouse architecture enables it to be adaptive to business’ future analytical requirements. Data in a lakehouse can be stored in its raw form without any predefined schema or structure, so you can capture and store diverse datasets from various sources without worrying about upfront transformations or schema modifications.
Increasingly, data lakehouses are being used to accelerate new and emerging business cases such as Internet of Things (IoT) insights and real-time insights, as well as reduce costs and improve data governance. Forrester recommends that companies work with providers that leverage GenAI capabilities in the platform; deliver an end-to-end integrated lakehouse that includes streaming, transformation, workload management, integration, governance, and security; and deliver performance at the speed of business with features such as built-in automated performance optimization, advanced workload management, and parallel data processing and transformation.
Turning Attention to Data Observability
“Through 2026, two-thirds of enterprises will invest in initiatives to improve trust in data through automated data observability tools addressing the detection, resolution, and prevention of data reliability issues,” according to Matt Aslett, Director of Business, Research and Data at ISG and formerly VP and Research Director at Ventana Research, which ISG acquired in late 2023.
Ventana’s Analytics and Data Benchmark Research has shown that only 20% of participants were very confident in their ability to analyze the quantity of data needed to make informed business decisions.
Conclusion
The value to be gained from leveraging a well-defined data architecture as the foundation for decision-making will only continue to grow. So too will the complexity of maintaining it as data sources proliferate, data types diversify, real time processing demands expand, and cloud-based data and storage platforms flourish in an increasingly AI-powered world.
Start planning now for how you will accommodate these changes and accelerate business advantage.