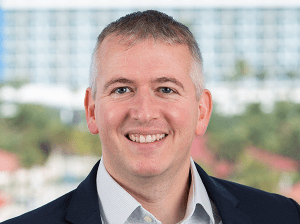
Connected intelligence (CI) and end-to-end quality are two concepts that, when combined, have a transformative impact on the future of drug development and clinical trials, marking a significant change in how pharmaceutical companies manage the process of bringing new products to market. By combining different technologies and methods to build a smooth, data-focused environment that improves quality, efficiency, and decision-making in drug development, traditional silos can be broken down to create a seamless data-driven ecosystem.
Defining a New Era
At its core, CI refers to the interconnection of various data sources, systems, and processes that can connect clinical data with quality, regulatory, and safety data. This connection enables immediate sharing, analysis, and insight generation, eliminating barriers between drug development stages and departments in pharmaceutical companies as new products move into production. In drug development, CI enables researchers and decision-makers to access and analyze data from multiple sources simultaneously. This might include preclinical studies, earlier clinical trials, real-world evidence, manufacturing processes, and even data from wearable devices used in ongoing trials. Integrating these varied data streams helps pharmaceutical companies better understand a drug’s effectiveness, safety, and possible challenges.
The power of end-to-end quality lies in its holistic approach to quality management. Instead of viewing quality as isolated checkpoints, this method considers quality an essential part at each stage of drug development, including research, clinical trials, manufacturing, distribution, and post-market monitoring (pharmacovigilance).
CI and end-to-end quality are dismantling traditional silos and fostering a seamless, data-driven ecosystem. The use of CI, potentially with data lakes as a way of consolidating vast amounts of data from disparate sources, removes silos that exist between independent systems sitting with siloed departments. The movement of data, for example clinical data that is needed in regulatory submissions, or safety data that is needed alongside regulatory data for regulatory reports, brings a level of fluidity to data management and helps companies optimize time and resources to generate product quality and safety insights. Data management is still needed to maintain data congruency, quality and minimize data bias.
Key Technology Drivers
Interconnectivity is enabled by many technologies, including artificial intelligence (AI), machine learning (ML), blockchain, and the Internet of Things (IoT). These various technologies are tools that can bring improved efficiencies and effectiveness to industry professional and drug development/ commercialization activities by connecting a variety of structured and unstructured data that sit in differing internal and external sources in differing structures and with differing quality, congruence, and bias. In effect, these tools allow data to be connected, searched, and scaled to drive efficiencies, effectiveness, and insights that enable professional human activities in the development and commercialization processes.
The integration of AI and ML is crucial in realizing the full potential of CI. These technologies can process and analyze vast amounts of data, identifying patterns and insights that might be missed by human researchers. In the context of end-to-end quality, AI can predict potential quality issues before they occur, allowing for professional teams to conduct further investigations which may lead to proactive interventions.
Blockchain technology can play a vital role in ensuring data integrity and traceability within this connected ecosystem. By creating an immutable record of all data transactions, blockchain enhances transparency and trust in the data used for decision-making throughout the product lifecycle.
IoT is another key enabler of CI, whereby devices can provide real-time data from manufacturing processes to patient diagnostics and monitoring in clinical trials. This continuous stream of data supports the end-to-end quality approach by allowing for immediate detection and correction of any deviations from quality standards. Naturally, any use of such data is subject to global regulations.
Cloud computing and advanced data analytics platforms are crucial for enabling CI. They provide the necessary computational power and storage capabilities to handle the massive amounts of data generated throughout the drug lifecycle. These platforms also enable real-time collaboration among geographically dispersed teams, further enhancing the efficiency of drug development efforts and monitoring of end-to-end quality.
AI Applications in Early-Stage Molecular Research
AI applications are currently in use in early-stage drug development and molecular research. The application of AI in pharmaceutical development extends to fundamental molecular research, particularly in protein folding and receptor interaction analysis.
These systems can rapidly analyze countless molecular configurations, helping identify promising drug candidates through a process like shape matching between molecules and cellular receptors. What’s more, the use of generative AI is bringing predictive analytics into early-stage clinical activity that is enabling the shapes of key molecules to be predicted, which can then lead to professional-led verification activities in the bounds of clinical studies.
While AI significantly speeds up the initial discovery phase of the typical 11-year development cycle, human experts remain essential for validating predictions and guiding development. This combination of AI efficiency and human oversight could lead to faster, more cost-effective drug development across therapeutic areas.
Improving Patient Outcomes, Reduced Trial Times, More Robust Data
For clinical trials, CI and end-to-end quality can significantly enhance patient recruitment and retention. Advanced analytics can identify suitable candidates more efficiently, while real-time monitoring through connected devices can provide continuous data on patient responses and the identification of potential adverse events. This improves the quality of data collected, enhances patient safety and reduces trial time and cost.
Efficiencies and effectiveness gains drive operational timelines, and insights help in the identification of data bias / bias reduction through suggesting data gaps in clinical activities. Insights also help identify unforeseen failure mode, through signal detection identify potential risks that need addressing to reduce the impact on global populations.
The use of AI to data mine populations/demographics can provide insights to where a population is under/over recruited. Using AI to mine cohorts of trial volunteers can help maximize the uptake of patients for the right trial in terms of timeline and trial quality benefits.
In process controls, AI can help identify trends (product composition, packaging dimensions, production environmental controls, and so on) and provide in process calibration prior to a deviation beyond tolerance, or to identify early where a trend to a tolerance breach will occur. This can optimize manufacturing processes, reduce waste, minimize the impact of global remediations and, in turn, this pulls through to reducing risk of supply, quality, and safety issues. A simple CI/end-to-end quality activity is the use of digital tools to execute quality control reviews and record documentation in digital format for consumption across the organization.
Aligning and Shaping Regulatory Requirements
The implementation of CI and end-to-end quality has significant implications for regulatory compliance. By providing a comprehensive, real-time view of all quality-related data, these approaches can streamline regulatory submissions and inspections. They also support the growing regulatory emphasis on quality-by-design principles and continuous process verification.
CI and AI-driven regulatory intelligence, in the context of quality-controlled procedures, can support the gathering of global submission requirements and the creation of global submission content, which will then be subject to human review as part of QC. How these technologies are used is also shaping the regulations themselves as seen with the EU AI Act and other global acts that are in development.
Looking at the Implications for clinical trials, regulatory alignment, and industry adoption, ultimately industry, regulators, and patients alike are focused on ensuring the provision of safe and effective healthcare solutions to global markets. The balance between allowing controlled innovation (with appropriate monitoring for safety events and the like as safeguards) and ensuring regulatory controls to hold industry to standards before product solutions are released to market, will continue to be debated with the potential for continued global variance and, in some cases, divergence. This variation will drive cost pressures on industry as they look to navigate a maze of global complexity, potentially using CI and end to end quality to reduce the pressures of financial constraints and optimize quality, regulatory and safety professional activities.
However, the adoption of these approaches is not without challenges. Data privacy and security concerns, particularly in the context of clinical trials and the use of data from connected devices, must be carefully addressed. There is also a need for standardization of data formats and integration protocols to ensure seamless communication between different systems and organizations.
The shift toward CI and end-to-end quality requires a cultural change within pharmaceutical organizations. It necessitates breaking down traditional departmental silos and fostering a more collaborative, data-driven decision-making culture.
Despite these challenges, the potential benefits of CI and end-to-end are immense. They promise to accelerate drug development timelines, reduce costs, improve product quality, and bring safe and effective treatments to patients in global markets. As these approaches continue to evolve and mature, they are likely to become increasingly central to the future of pharmaceutical research, development, and distribution.
Concluding Thoughts
There is no doubt that the integration of CI and end-to-end quality represents a significant and fundamental shift in pharmaceutical development, offering a compelling vision for the industry’s future. By leveraging advanced technologies within a unified ecosystem, pharmaceutical companies can dramatically improve their efficiency, data analysis capabilities, and decision-making processes across the entire drug development lifecycle. While challenges around data privacy, security, and organizational culture change must be carefully managed, the potential benefits – from accelerated drug development timelines to enhanced patient outcomes and streamlined regulatory compliance – make this transformation not just desirable but essential. As these technologies continue to mature and industry adoption grows, they will likely become fundamental to delivering safe, effective treatments to global markets more efficiently than ever before.