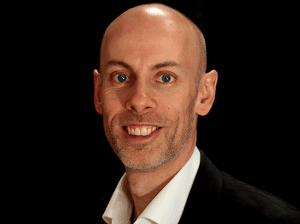
Imagine a world where AI doesn’t just predict the future – it lives in it. A world where machine learning models aren’t stuck reminiscing about last year’s data, but are instead surfing the cutting edge of now. This isn’t science fiction; it’s the new frontier of artificial intelligence, and it’s being shaped by an unlikely hero: streaming data.
In an age where information moves at the speed of light, our AI systems are facing a critical challenge. They’re brilliant, sure, but they’re also a bit like that friend who’s always a step behind on the latest news. It’s time for an upgrade, and that upgrade is happening right now at the intersection of generative AI and real-time data streams.
This isn’t just another tech buzzword or a minor improvement. We’re talking about a fundamental shift in how AI operates, learns, and interacts with the world. It’s a change that promises to address some of the most pressing issues in artificial intelligence today, from embarrassing “hallucinations” to outdated recommendations.
So buckle up, data enthusiasts. We’re about to dive into a world where AI thinks as fast as we do, where machines don’t just learn from the past but actively engage with the present. Welcome to the real-time revolution – a journey that’s reshaping the very foundations of artificial intelligence as we know it.
The Achilles’ Heel of Traditional AI Models
Traditional AI models, including large language models (LLMs) from OpenAI, Anthropic, and others, are trained on vast amounts of historical data. While this approach has yielded impressive results, it comes with a significant limitation: the potential for outdated or irrelevant outputs.
If you only train your AI on historical data, the AI model can spit out the wrong answer as a “hallucination.” It’s as if these models are stuck quoting movies from the ’90s – entertaining, perhaps, but not exactly cutting-edge. This phenomenon occurs because the training data may not reflect current reality or domain-specific knowledge, leading to responses that are inaccurate or outdated.
Streaming Data: The Firehose of Truth
Enter streaming data – the continuous flow of information from various sources in real time. By integrating streaming data into AI systems, we can create models that are not just knowledgeable, but also current and contextually aware.
The key lies in the ability to process and analyze data as it’s generated. Real-time analytics and AI are driving streaming data adoption. A recent survey found that real-time analytics is the leading current use case (71%) for adopting streaming data systems. Nearly three in four respondents cited the development of AI/ML systems as the biggest driver of streaming data adoption. This shift toward real-time data generation and consumption is driving the need for AI systems that can keep pace.
RAG: The AI’s Fact-Checking Sidekick
One of the most promising approaches to integrating streaming data with AI is through retrieval-augmented generation (RAG) databases. RAG systems allow AI models to query external knowledge bases for the most up-to-date information before generating a response.
These RAG databases help deal with the fact that the models were trained on old or generic data, and in some cases, old data will not give you the best recommendation based on the current data that’s coming in. You can think of RAG as an AI’s personal fact-checker, always ready to double-check information before giving a result.
By implementing RAG, organizations can significantly reduce hallucinations and improve the accuracy of their AI outputs. This is particularly crucial in fast-moving industries where decisions need to be made based on the most current information available.
Real-World Applications: Where Streaming AI Shines
The integration of streaming data and AI is already being applied across various industries with remarkable results:
- Financial Services: Real-time fraud detection systems can analyze transaction patterns as they occur, flagging suspicious activity before it results in significant losses. Every bank has a sophisticated fraud detection engine that’s correlating location and purchase history to evaluate the likelihood of any financial foul play.
- Manufacturing: Quality control processes are being revolutionized by AI-powered visual inspection systems that detect defects in real-time around tolerances. These systems can self-regulate based on streaming data, maintaining optimal production quality without human intervention by also evaluating more than just the part being manufactured. Real-time systems can also look at temperature, viscosity, and the weather, and not just give an error when a part is out of compliance but help diagnose the core issue in the system.
- Ad-Tech and Retail: Personalized recommendations can be generated based on a customer’s real-time behavior, significantly improving conversion rates. A study by McKinsey found that personalization can deliver five to eight times the ROI on marketing spend and lift sales by 10% or more.
- Cyber Security: Real-time anomaly detection and pattern matching are now the gold standard for cyber security. Systems that can evolve their threat detection engines as quickly as their adversaries have a significant competitive advantage in the marketplace, leading to fewer false positives and higher detection rates. Streaming of threat data and real-time model tuning are key to unlocking best-in-class results.
The Rise of Sovereign AI: Keeping Data Close to Home
As organizations recognize the value of their data, there’s growing concern about sharing it with third-party AI providers. It is already untenable for companies to send customer data or intellectual property to the big AI companies en masse. This has led to the concept of sovereign AI – the ability to run AI models on-premises or in controlled environments to maintain the highest levels of data privacy and security.
Sovereignty is very important for privacy and business protection, and will likely be mandated in many industries, as companies want to hang on to their proprietary data, use that specific information to make their AI models better, and ensure that an AI model gives the most accurate results.
The advent of sovereign AI allows companies to leverage their proprietary data streams without compromising security or competitive advantage, especially for financial and healthcare services.
Conclusion: Embracing the Real-Time AI Revolution
The marriage of streaming data and AI represents a significant leap forward in our ability to make informed, real-time decisions. By addressing the limitations of historical data and embracing the power of real-time information, organizations can create AI systems that are not just intelligent but also highly relevant and responsive to the world around them.
The idea that AI is going to apply to real-time events is truly important, and something many companies may not grok yet when they’re still trying to get accurate results from a stagnant, pre-trained LLM. But streaming data is going to become a super common, important thing for generative AI.
For businesses looking to stay ahead in the AI race, the message is clear: Start exploring how you can integrate streaming data into your AI strategies today. The future of AI is real time, and it’s happening now.