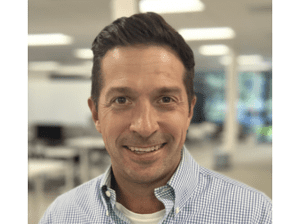
Around this time of year, many data, analytics, and AI organizations are planning for the new year, and are dusting off their crystal balls in an effort to understand what lies ahead in 2025. But like all predictions, they are only helpful if they are right.
If you are a fantasy sports fan like me, you know what it feels like to put a highly projected player in your lineup, only to have them make an impressive catch, outmaneuver the defense, and fumble the ball after so much effort. With this in mind, I wanted to reflect on my 2024 predictions to see if I hit it out of the park or if I got crushed by a rookie starter.
1. Data Lakehouses Will Become the Primary Architecture for Analytical Workloads
Prediction: “Data lakehouses will rise as the dominant platform for analytics, surpassing legacy data warehouses.”
What Happened: This prediction has largely come true. According to my company’s State of the Data Lakehouse Survey Report, 69% of respondents expect more than half of their analytics to be conducted on the lakehouse within the next three years, and 42% of lakehouse data is coming from cloud data warehouses. Data lakehouses offer significant benefits over legacy architectures such as cost efficiency, interoperability, and no lock-in. Much like the survey shows, and based on conversations with customers, the lakehouse is proving to be a leading architecture for the AI era. However, it has yet to overtake the combination of cloud and legacy warehouses in query volume.
2. Apache Iceberg Will Become the Most Adopted Table Format, Surpassing Delta Lake
Prediction: “Apache Iceberg will overtake Delta Lake as the most popular table format for lakehouses due to Iceberg’s flexibility and openness.”
What Happened: This prediction came true, as evidenced by Databrick’s purchase of Tabular and Snowflake open sourcing an Iceberg catalog, Apache Polaris (incubating). Apache Iceberg is the open standard for lakehouse table formats. Organizations are selecting Apache Iceberg for its open architecture, and recent surveys show it has become the preferred choice for data interoperability. As more and more businesses look for flexibility and avoid vendor lock-in and ownership over their data, Iceberg’s rise as the open standard makes sense and is exciting.
3. DataOps Will Move from Hype to Production with CI/CD, Git-Inspired Version Control, and Automated Data Quality Checks
Prediction: “DataOps, which integrates development best practices like continuous integration/continuous delivery (CI/CD) and version control into data management, will see widespread adoption, leading to more automated, efficient data pipelines.”
What Happened: DataOps as a trend has been subsumed by the rise of data products this year. The principles of what is needed to manage and version data to ensure data uptime and quality are still there, but the terminology has changed. While more of these practices did go into production in 2024, there is still a lot more change that organizations will need to go through to implement these practices into their organizations. The prediction was right, as these practices have moved into production, but the pace with which they move into production was wrong. dbt, SQL engines, and lakehouse catalog adoption are key elements of moving to data products and automated DataOps processes.
4. Data Mesh Pillars Will Become a Core Requirement for Data Teams to Spur Adoption and Improve Data Quality
Prediction: “Expect data mesh to continue growing, with organizations adopting its principles to decentralize data ownership and improve collaboration across data teams.”
What Happened: This 100% happened, but it should have said “One Data Mesh Pillar”: data products (see prediction #3 above). The organizations we have been speaking with have no interest in fully decentralizing data governance. If anything, they want to do away with extracts and shadow IT that makes governance difficult but ensure that business units aren’t blocked (and angry), because they can’t access the data they need to deliver AI and BI projects quickly. Data products reconcile both governance and agility. Central teams can apply enterprise-wide policies across all data, without extra copies and decentralized teams can add in additional governance, because they understand the semantics of the data. This unblocks business units and enables them to rock and roll without tickets. Central data teams hate tickets as do business units. Data products proved to be the preferred method to a ”no-ticket” future.
5. Generative AI Will Be Used by Data Engineers on Nearly Every Project, Improving Productivity by a Third
Prediction: “Generative AI will become an integral tool for data engineers, improving productivity by automating data tasks and driving user interaction through AI-powered interfaces.”
What Happened: This prediction also has largely materialized. Generative AI has transformed the way data teams work, especially with tasks like semantic search, data discovery, and pipeline automation. The integration of generative AI in data tools has led to significant productivity boosts, and as expected, AI has become embedded in data workflows. The foundation for self-service data products, which generative AI helps power, is also paving the way for more democratized and accessible data in organizations. That said, we have no idea if teams have seen a full 30% productivity gain, as many teams are not yet thinking “AI-first” in their projects and pipeline work.
Measuring Up
In aggregate, I’d give us a B, maybe a B+, on last year’s predictions. They were useful to the market, and if used on the starting lineup, we would have won. For those that went all in and established an Iceberg lakehouse and leveraged DataOps best practices to generate data products with GenAI, chances are they would win the 2024 championship.
Looking forward, it will be exciting to see what new trends evolve and continue to shape the future of data and analytics. Stay tuned for more updates and new predictions as we move into 2025!