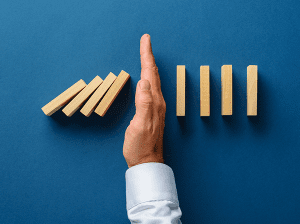
In today’s data-driven business landscape, data quality – the availability of usable and business-ready information – remains a significant and worsening challenge for many organizations. To mitigate these effects, businesses need swift resolution of data issues with transparent and trustworthy information.
However, in our fast-paced digital environment, complex data architectures with more system variables make it difficult to understand the problems. Enter data lineage, a comprehensive audit trail that traces data origins, movements, and transformations. It plays a crucial role in explaining and clearing up these data problems.
Fueled by the organization’s metadata, data lineage must be clear, timely, and accurate. Achieving this goal requires an enterprise’s data governance (DG) to take charge, providing clean metadata definitions, production, and usage in a unified view.
In response to these challenges, advanced data lineage methodologies and technologies have emerged as essential tools for modern businesses. Advanced data lineage (ADL) enables organizations to move from reactive to proactive problem-solving, supporting a more agile data governance response. This shift represents a significant evolution in how companies handle their data assets, promising improved efficiency, compliance, and decision-making capabilities.
What Is Advanced Data Lineage?
Advanced data lineage (ADL) provides a powerful set of tools for understanding data’s history. It is proactive and preventative, addressing data issues at that moment or before they happen.
Advanced data lineage represents a significant evolution where historically, traditional data lineage tracks data movement and transformations linearly. Consequently, organizations often receive static reports that quickly become outdated in fast-changing data environments.
The advanced data lineage mindset supports a dynamic environment with a unified view.
ADL advantages enable organizations to:
- Predict how data changes will impact downstream systems
- Control changes through versioning
- Keep track of data and processes
- Handle a complex data architecture with at least one cloud component
- Integrate their data lineage module with other tools
- Comply with emerging legislation
- Facilitate collaboration and communication through a shared understanding
To leverage these benefits, organizations need a data governance program to coordinate lineage tools with roles, responsibilities, and processes.
The Intersection of Advanced Data Lineage and Governance
A data governance (DG) program supports the business by formalizing and controlling data activities It harmonizes data roles, practices, and technologies across the enterprise, leading to better data quality. Stakeholders collaborate and agree on what the data means and its usage, breaking down departmental data silos.
To achieve this outcome, people across the organization must have transparency and trust. ADL provides a foundation for this confidence by providing an accurate and clear history. With a single view of this evidence, teams can identify the root of a data quality issue and agree on an approach to a solution.
The speed at which a data quality issue is resolved using ADL also reduces risks. Typically, DG programs must ensure their data handling complies with laws, like the General Data Protection Regulation (GDPR) and the Health Insurance Portability and Accountability Act (HIPPA). Accurate data lineage guides compliance and assures the business that it handles data legally.
The longer a data quality issue is unaddressed, the more likely someone will notice it downstream, with the potential for a fine or loss of business. To avoid this impact, DG uses ADL when developing policies.
Impact on Data Governance Practices
As ADL transforms how organizations understand and manage their data, it requires a corresponding evolution in data governance practices. This transformation requires more than selecting the right software; it applies an adaptive framework that supports efficient assessments and actions on lineage information. An adaptive Data Governance framework is flexible enough to respond quickly to new insights provided by ADL, while still maintaining a structured approach to data management.
With this shift comes increased and frequent interactions between adaptive DG teams and other departments to resolve issues. To do this well, a framework should clearly define roles, responsibilities, and escalation paths when addressing issues identified by ADL. This approach is agile while maintaining a solid methodological foundation.
Additionally, organizations need to back up any ADL app with skills and standardization. Workers need data literacy – the capability to read ADL visualizations, interpret them, and respond well to that information. The ADL platform needs high-quality metadata management so that the lineage information is standardized. Adaptive DG needs to endorse both.
Coordinating all these adaptive DG components requires a culture and practices that embrace greater data transparency and accountability. Building this foundation requires maturation, which takes time.
Challenges to Leveraging ADL in Governance
Not every organization has the maturity to benefit from an ADL approach. Instead of considering data quality issues as educational opportunities for improvement, some companies foster blame and cover-ups. Then the ADL visualizations and output by the software become fodder to explain why one group is at fault.
For example, a health insurance company hired a development team to create a health survey for its customers. When testing the application, the developers received an error upstream: the customer accounts system would not send the data. The survey would not work.
During a call, the engineers blamed customer accounts for the problems. However, customer accounts accused the engineers of not understanding data regulations and requesting personal health information unnecessarily. Both use the ADL visualizations as evidence.
On the other hand, an organization with a more mature adaptive data governance program may have invested in building relationships between engineers and customer accounts through data management. It may have established concrete guidance on how to generate data that the developers could use when securing the personal health information in the accounts system.
These capabilities evolve, slowly, with proven data governance results. Building this solid foundation of trust, through data governance, needs to happen before implementing the ADL approach. The ADL success stories demonstrate this reality.
ADL Success Stories
The examples below show how each company took steps to first build a solid foundation. Then they applied ADL.
JP Morgan
In 2020, JP Morgan highlighted a commitment to digitize their operations and build a data-driven business. With these resources, in 2021, the enterprise took a step-by-step approach, creating a solid foundation for a data mesh architecture.
The data mesh strengthens data accessibility and shareability. One of its components includes a federated data governance program, empowering the experts in the data to control data decisions.
By 2023, the financial institution was leveraging advanced data lineage capabilities. It had an internal platform that moved and managed data. It provided visibility into data sharing and lineage.
Since successfully implementing data mesh, JP Morgan had the guidance, roles, and processes to make the best use of this solution. Consequently, the company improved its capabilities with its federated governance and in addressing data issues in real-time.
CHRISTUS Health and Advanced Data Lineage
JP Morgan is not the only use case that demonstrates the success of advanced data lineage built from a sound foundation. CHRISTUS health has a similar story.
CHRISTUS Health’s commitment to transparency happened during a shift to a centralized data governance model. In 2015, the IT Finance team implemented a solution that gave the business better visibility into the spend for technology services.
The company continued its digital transformation by implementing a flexible learning platform in 2020. This approach increased organizational data literacy. The first training was for an Enterprise Resource Planning (ERP) tool managed by IT.
By 2023, CHRISTUS Health implemented automated data lineage tools to manage EHR system impacts and to proactively identify and resolve data issues. It was able to leverage the MANTA automated lineage solution and support it with a strong demonstration of data transparency. Since implementing an advanced data lineage, CHRISTUS has used a proactive approach to resolving data quality issues.
Conclusion
Advanced data lineage offers powerful tools for resolving data quality issues. It provides unified visualizations that foster transparency and trust. By enabling quick identification and resolution of data problems, ADL equips data governance teams with the means to maintain data integrity and compliance.
However, the successful implementation of ADL requires a solid foundation of adaptive data governance structures. Organizations must first cultivate an environment of transparency and trust before they can fully leverage the benefits of ADL.
The examples above demonstrate the creation of a solid, adaptive DG foundation. After some time and proven success, JP Morgan and CHRISTUS Health added the advanced data lineage approach.
So, companies need to evaluate their data governance maturity. Some need to foster trust across teams and develop clearer guidance. For others, who are more mature, ADL technologies and methodologies provide a good resource to better their data quality and resolve issues efficiently.