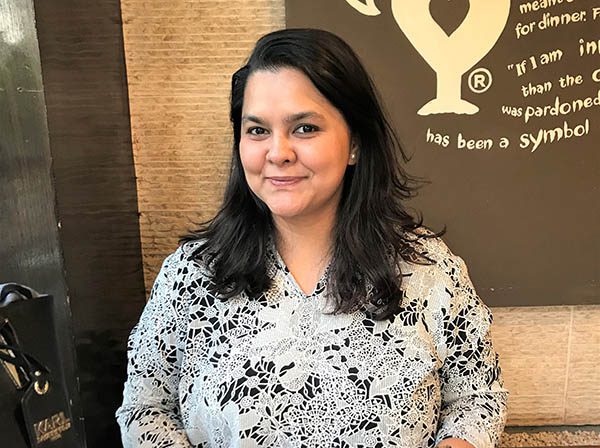
In a recent conversation with one of our customers, my team uncovered a troubling reality: Poor data quality wasn’t just impacting their bottom line but also causing friction between departments. In one instance, they explained how their marketing team could not run a campaign on time because they had to wait for the IT team to clean, dedupe data, and send over a clean contact list. Meanwhile, the IT team had other tasks to manage and so failed to prioritize marketing requests.
The result?
A junior marketing analyst had to manually clean the list, causing unnecessary delays and affecting the company’s bottom line.
This is just one example.
In another conversation, a company’s CEO shared how he lost trust in his sales team after discovering that nearly 20,000 of the 70,000 contacts they claimed to have were duplicates, and many others were incomplete or inaccurate. They only discovered the extent of the duplicates when they used AI-powered data matching to link records.
In the many years that I’ve worked with customers, both as a business consultant and now as a member of a data company, I’ve seen how data can create conflicts.
Some common scenarios that lead to conflicts:
- The data team delivers inconsistent reports to the finance department, causing errors in quarterly projections. This sparks conflict, and teams start blaming each other for missed revenue targets – until someone loses a job.
- An executive pulls real-time analytics during a meeting, only to find outdated data in the dashboard, embarrassing the leadership team. The conflict escalates as the data team scrambles to defend their process against accusations of incompetence.
- Product managers ask for customer insights but receive irrelevant or incomplete data from the analytics team, delaying product decisions. This sparks tension, with each side blaming the other for unclear requests and data mismanagement.
- A sales team relies on customer data to drive their outreach but finds multiple records for the same leads, leading to duplicate contact attempts and frustrated prospects. Tensions flare as sales blame the data team for the mess, while the data team points out that proper data entry protocols weren’t followed from the start.
These are real problems companies face every day in a business environment.
So, how do we solve them? Where do we begin?
Through our conversations with customers and industry experts, we’ve identified a few golden rules of management for businesses to minimize tensions caused by poor data.
Rule 1: Do not attempt to sweep the problem under the rug. As the saying goes, admitting you have a problem is the first step toward solving it. When it comes to data quality, this couldn’t be truer. Only by openly addressing data challenges can businesses begin to implement meaningful solutions. You can’t wish bad data away – you can only tackle it head-on.
Rule 2: Make data quality audits and correction plans a routine task. One of the biggest mistakes businesses make is treating data quality issues as a one-time problem rather than an ongoing process. When data issues are identified too late in the process, it can lead to significant setbacks for all stakeholders. Data teams often bear the brunt of the blame, as conflicts arise between them and data consumers when pipelines fail to provide accurate, timely information.
Establishing a regular audit routine and subsequent correction plans prevents the onset of sudden or unexpected challenges. Your team should not panic when a report is due, nor should they wait for other department members (like IT) to get accurate data.
Rule 3: Prioritize data quality as a key talking point in meetings. While it’s easy to focus on impressive charts and positive trends in team meetings, how often do we discuss the accuracy of the data behind those visuals? How many business users know concepts like data deduplication or record linkage? Sure, these are IT concepts, but then again, business users are the true custodians of customer data. They need to understand the issues affecting data accuracy and reliability – primarily since they rely on this data to drive campaigns, reports, and business-critical decisions.
Bringing data quality into regular conversations aligns everyone with potential challenges and warms up collaboration between tech and business teams – historically, which has always been disjointed and disconnected.
Rule 4: Be clear with job roles. For example, asking your marketing and salesperson to clean a contact list without providing the necessary data quality tools to do the job sets them up for failure. Or, asking your IT teams to create dashboards and presentations for marketing without giving them the context of the data is also setting them up for failure. Be clear with job roles and ensure your teams have the right tools, processes, and context to work on data.
Rule 5: Leadership teams need to understand data challenges. Leaders can’t afford to make decisions based on flawed data, but many do because they don’t fully understand the data issues at play – like duplicate IDs leading to potential security breaches, poor data practices leading to potential fines and penalties, or even missing records and outdated info that directly impact bottom lines. If leadership teams don’t get a handle on these problems, they risk steering the business in the wrong direction.
Remember the CEO who green-lit a major sales push based on what he thought was accurate customer data, only to find out half the contacts were invalid? The result: wasted budget, missed targets, and frustration across the team. If the leadership team had known about the data mess earlier, they could have fixed it before throwing money down the drain.

The Cost of Inaction
We know that ignoring data quality issues can lead to far more than just inefficiencies. The financial costs alone can be staggering – research shows that poor data quality costs organizations an average of $12.9 million annually. Beyond the monetary losses, companies risk damaging their reputation when poor data leads to customer dissatisfaction, missed opportunities, and errors in decision-making.
For example, in the retail sector, sending promotions to incorrect or inactive customer contacts not only wastes marketing spend but also risks losing potential repeat customers. Similarly, inaccurate data can lead to flawed forecasts, steering the company in the wrong direction and wasting resources on failed strategies.
Inaction isn’t just a technical oversight – it’s a business risk that can affect every department and, ultimately, the company’s bottom line. Leadership must recognize that failing to address data quality issues early can result in far bigger costs down the road, from financial losses to damaged customer trust. That leads us to the ultimate rule: Data quality is a top-down approach.
Data quality must be driven from the top. It’s not just an IT or operational issue; it’s an organizational responsibility that requires leadership to set the tone. When leadership prioritizes data quality, it sets the tone for the entire company. Without buy-in at the top, teams will continue to work in silos, cutting corners and ignoring the very issues that undermine their work. Only when leaders actively promote and invest in data quality will it become embedded in the culture, ensuring that every department treats data as the critical asset it truly is.