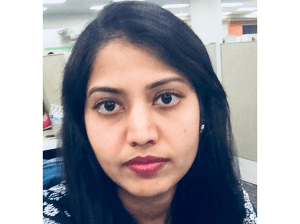
Climate change presents one of the most significant challenges of our time, demanding immediate and sustained action across all sectors of society. As temperatures rise, ice caps melt, and weather patterns become increasingly unpredictable, the urgency to understand, mitigate, and adapt to these changes becomes critical. In this context, innovative technologies, particularly artificial intelligence (AI), are emerging as essential tools in the battle against climate change. AI’s capacity to analyze vast datasets and model complex systems makes it uniquely suited to mitigate the effects of climate change and adapt to its inevitable impacts.
AI has carved out a pivotal role in environmental science, providing researchers and policymakers with previously unattainable insights. Through its advanced computational power, AI can process and analyze the extensive and complex datasets characteristic of environmental studies, from satellite imagery tracking deforestation to data from ocean buoys monitoring sea temperatures. This capability allows for a more nuanced understanding of environmental changes and aids in predicting future conditions more accurately.
Understanding AI’s Role in Climate Science
At its core, AI involves creating algorithms capable of processing information and making decisions or predictions, closely mirroring human cognitive functions. AI excels in data analysis and modeling intricate systems influenced by a myriad of interacting factors in the realm of climate science. By applying pattern recognition and predictive analytics techniques, AI systems can identify trends and anomalies that may elude human analysts. The types of AI models that are most prevalent in climate-related studies include machine learning and deep learning.
- Machine learning: Without explicit programming, these models learn from historical data to make predictions or decisions. Machine learning can be used in climate science to forecast weather events, predict solar and wind power generation based on weather data, and optimize systems for energy efficiency.
- Deep learning is a subset of machine learning that uses neural networks with many layers (hence “deep”) to analyze various factors in the climate system. These models are particularly effective at processing unstructured data, such as images and text, which are abundant in climate studies, such as satellite images for tracking storm paths or land-use changes.
Integrating these AI technologies into climate science has not only enhanced existing analytical tools. It has also enabled the development of new methods that can provide deeper insights into the complex dynamics of climate systems. This has opened up new avenues for both the mitigation of climate change effects and adaptation strategies, thereby illustrating the indispensable role of AI in addressing one of the most pressing issues of our age.
Innovative AI Models in Action
AI’s capabilities extend far beyond general data processing; they are actively reshaping how we understand and respond to the intricacies of climate dynamics. For instance, sophisticated AI models are now pivotal in predicting weather patterns and climate anomalies with greater accuracy and timeliness than ever before. These models harness vast arrays of meteorological data to forecast extreme weather events, such as hurricanes and heatwaves, allowing for more effective early warning systems and preparedness strategies.
A notable example is the use of machine learning techniques to enhance the predictive accuracy of hurricane tracking. AI models can predict a hurricane’s path and intensity by analyzing historical data, satellite imagery, and ocean temperature patterns, which are critical for timely evacuations and resource allocation. Similarly, AI has been instrumental in detecting and analyzing climate anomalies such as the unexpected El Niño and La Niña effects, providing insights crucial for agricultural planning and water resource management.
AI models are equally transformative in terms of the carbon cycle and broader environmental monitoring. For example, researchers have developed AI systems that can estimate atmospheric carbon flux by analyzing satellite and sensor data. This allows for more accurate measurements of how much carbon dioxide ecosystems absorb and release, informing climate policy and carbon trading markets.
AI in Climate Mitigation
AI’s role in climate mitigation is perhaps most visible in renewable energy management, according to action understanding. AI algorithms are at the forefront of optimizing the performance of power grids that integrate multiple renewable energy sources. These systems can forecast power generation from solar and wind installations through predictive analytics, adjusting grid operations to balance supply with fluctuating demand. Additionally, AI enhances energy storage systems, predicting the best times to store energy and feed it back into the grid, maximizing efficiency and stability.
Another critical area in which AI significantly impacts the industry is the reduction of transportation and industrial process emissions. In transportation, AI applications range from optimizing routes for electric vehicles to reduce energy consumption to managing traffic flows in urban areas to minimize idle times. For industries, AI-driven systems can monitor and adjust processes in real time to ensure maximum energy efficiency and minimum waste production. For instance, smart factories use AI models to predict machine failures and optimize maintenance schedules, thereby reducing downtime and energy waste.
These applications underscore AI’s pivotal role in direct climate action and highlight how integrating advanced technologies into environmental strategies can lead to substantial gains in our efforts to mitigate climate impacts. As we refine these technologies and expand their applications, the potential for significant change grows, marking a hopeful path forward in the ongoing battle against climate change.
AI in Climate Adaptation
Artificial intelligence is not just a tool for mitigation but is also pivotal in adapting to changing climate conditions. AI enhances disaster response and management by providing accurate and timely information, which is crucial for managing the effects of natural disasters like floods, hurricanes, and droughts. For instance, AI models that analyze weather data, historical event data, and geographical information can accurately predict where extreme weather events are likely to strike, facilitating better preparedness and resource allocation.
In agriculture, AI’s impact is transformative, helping to adapt to changing climatic conditions that affect food security worldwide. Through advanced imaging from drones or satellites, AI-enabled systems can monitor crop health, predict yields, and even detect plant diseases early. Furthermore, AI-driven robotic systems can provide targeted pest control, reducing the need for widespread pesticide use and minimizing environmental impact while maximizing crop productivity.
Challenges and Ethical Considerations
However, deploying AI in climate science is not without its challenges. Data quality remains a significant concern, as AI systems require large amounts of high-quality data to function optimally. Incomplete or biased data can lead to inaccurate models, which could lead to poor decision-making. Scalability is another challenge, as solutions proven in small-scale studies often encounter obstacles when scaled to regional or global levels.
Ethically, using AI in climate prediction and decision-making raises important questions about transparency and accountability. AI-influenced decision-making processes must be scrutinized to ensure they do not reinforce existing inequalities or bypass public scrutiny. Additionally, the potential for AI systems to make autonomous decisions in areas impacting human lives demands rigorous oversight and ethical considerations.
The Future of AI in Climate Science
Looking ahead, AI is poised to continue its role in climate science and expand it dramatically. Future developments could include more sophisticated neural networks that can model climate systems with greater accuracy, or new forms of machine learning capable of working with even smaller data sets to predict changes in climate patterns. AI’s ability to integrate data from disparate sources could lead to more comprehensive models that can better predict and manage the effects of climate change. International collaboration will be crucial in harnessing the full potential of AI in climate science. Cross-border sharing of research, data, and innovations can expedite the development of solutions and guarantee their availability where most needed. Such cooperation can also help to standardize data collection and analysis methods, making AI tools more robust and widely applicable.
AI’s potential to impact climate change mitigation and adaptation is growing. As this technology advances, it will become an even more integral tool in combating climate change. However, the success of AI in this field will heavily rely on continued research and development, alongside careful consideration of the ethical implications of its use. By addressing these challenges head-on, the scientific community can ensure that AI serves as a force for benefit in the urgent fight against climate change.