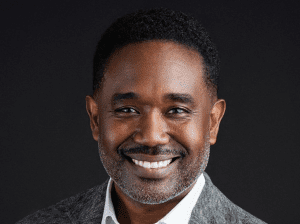
The widespread adoption of artificial intelligence (AI) and machine learning (ML) simultaneously drives the need for cloud computing services. Enterprises aiming to effectively train huge datasets and navigate advanced neural networks will need to rely on more flexible and dependable solutions for managing challenging computing tasks. That is why organizations should look to hybrid solutions – which combine dedicated servers and cloud resources – in place of a one-size-fits-all strategy.
However, to incorporate hybrid solutions adequately, enterprises must find a balance between reliability and adaptability. Elements of AI may need to be scaled up or down based on the state of the model, despite the core structure remaining unchanged throughout its existence. As technology continues progressing, it’s key for organizations to select data and hosting providers who can adapt and stay in step with their evolving requirements and infrastructure needs to enhance AI performance and outcomes.
Key Factors for Selecting Hosting Providers
Before selecting a hosting provider, organizations must have a clear understanding of the specific AI models in use, as these technologies vary greatly in computational demands. For instance, while simple algorithms such as linear regression may have modest requirements, the training of deep learning models involves processing vast amounts of data, requiring a robust computational infrastructure. It’s essential to assess the resource needs at each stage of development – whether it’s training, testing, or deployment. Selecting hosting providers that offer scalability, robust infrastructure, and specialized knowledge can lead to a more economical and effective foundation for an organization’s AI/ML initiatives.
Scalable solutions provide flexibility that allows for the adjustment of resources, such as computational power and storage, in line with the evolution of an AI model and ensuring that costs are kept to a minimum. For tasks demanding substantial computational resources, like training deep learning models, providers with strong high-performance computing (HPC) capabilities, including GPUs or TPUs, are a good choice.
Storage needs should also be considered, with high-speed storage being a must for frequently accessed training data. On the other hand, more cost-effective solutions might prove adequate for archived datasets.
Hosting providers should offer secure, low-latency networks to enable efficient data transfer and communication between processing units, ensuring smooth and secure operations. By choosing providers with experience and expertise in handling AI workloads, organizations rest assured that they can provide support and guidance tailored to their specific AI needs.
Data Readiness, Hosting, and Making the Most of Your AI Investments
To fully harness the potential of AI, a “data readiness” strategy is essential, with hosting playing a pivotal role. Data readiness refers to the preparedness of an organization’s data for use in AI models and involves the proper storage, processing, and management of data to ensure efficient use in AI/ML applications. AI models are dynamic systems that need to constantly ingest and adapt to new data, a process facilitated by hosting. As fresh data pours in, the hosting infrastructure ensures adequate storage and network capacity to manage the surge.
Organizations must find flexible solutions designed to provide customers with adaptive hosting throughout the AI lifecycle. With the ability to modify computational and storage capacity as needed with various hosting options, organizations can ensure cost-effectiveness by only paying for what they use at different stages of the AI lifecycle.
To account for evolving needs of the model, businesses can adjust their computing and storage requirements. By providing the right infrastructure for each step of AI investment, hosting aids businesses in crafting a robust “data readiness” strategy, which is crucial for effective AI utilization.
Overcoming the Challenge of AI/ML Storage and Computing
Clear and consistent communication between chief data officers (CDOs) and data engineers is vital for the successful implementation of AI/ML initiatives. CDOs need to articulate their business objectives and desired outcomes in straightforward terms, enabling data engineers to assess the processing needs of the chosen models. This assessment considers factors such as the characteristics of the data sets used, the models’ training requirements, and the anticipated workloads.
Once teams understand processing requirements, infrastructure planning can commence. Data engineers can leverage this understanding to devise a scalable solution that meets these needs, while CDOs can offer insights into acceptable latency levels and budget constraints. In this collaborative endeavor, considerations such as network performance, processing power, scalability, and high-capacity storage are all critical.
Post-setup, it’s essential for data engineers and CDOs to continue their collaboration, monitoring resource usage and model performance to identify optimization opportunities. This could involve exploring cost-cutting measures or innovative ways to reduce the models’ processing demands. Through this ongoing, transparent collaboration, CDOs and data engineers can ensure the necessary infrastructure is in place to support their AI/ML initiatives successfully.