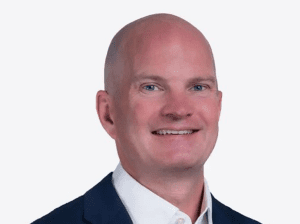
Organizations are racing to adopt AI for its promise of efficiency and insights, yet the path to successful AI integration remains fraught with obstacles. Despite advancements in tools like ChatGPT and Google’s Gemini, fundamental issues with data governance – such as high costs, poor data quality, and security concerns – continue to hinder progress.
Stop me if you’ve heard this one before: Even the most cutting-edge tools are only as effective as the data that fuels them. Herein lies the crux of the issue. Data governance is the cornerstone of many successful digital initiatives – especially AI. Without it, organizations and federal agencies are at the mercy of poor data quality, inadequate security measures, and siloed data ecosystems.
These issues not only delay AI adoption but also jeopardize the return on investment (ROI) for the companies that have already poured financial and employee resources into these technologies. As we look ahead, it becomes clear that, for AI to truly thrive, organizations must first tackle the age-old challenges of legitimate data management.
The True Cost of AI Adoption
The financial burden of adopting AI cannot be overstated. Training and deploying AI models, particularly large-scale ones, requires immense computational power, often necessitating multimillion-dollar investments in storage and GPU-based infrastructure. This hardware is not only expensive but also energy-intensive, raising operational costs even further. Moreover, the human resources needed to develop, train, and maintain AI systems add another layer of expense.
The return on investment (ROI) for AI remains uncertain, despite significant investments. It’s like taking a leap of computational faith. Will the costs of training, computing, storage, and energy end up being more than the benefits? Many companies are hesitant to fully commit to AI adoption due to the unpredictability of its outcomes. If manual intervention is constantly required to check the AI model’s work, how can you achieve a positive ROI from it?
The cost of failure is high, and without a clear, measurable return, it becomes increasingly difficult for organizations to justify the ongoing expenses associated with AI.
The Perils of Bad Data
One of the biggest challenges to AI success is the quality of the data it relies on. AI systems depend on well-structured, up-to-date, and accurate datasets for effective training. Unfortunately, many organizations struggle to access large, high-quality datasets, which can severely hinder AI performance and lead to inaccurate insights and decision-making.
Even more concerning is the possibility of using flawed AI-generated data to train future models. Trust is an essential aspect of AI adoption that is often overlooked. Organizations risk entering a cycle of nonsensical AI output and misguided decision-making, creating a harmful feedback loop that can perpetuate and amplify errors over time, ultimately diminishing the quality of insights generated by AI systems.
The Importance of Testing
For AI to deliver value, it must align with organizational goals and produce reliable results. However, validating AI outcomes is challenging, often requiring rigorous testing protocols that many organizations struggle to implement due to limited resources or expertise.
Testing AI typically involves two approaches: manual human validation and using AI to benchmark other AI systems. Yet again, this creates a recursive loop – AI testing AI – raising the critical question: How do you trust the tester? Defining truth is a fundamental challenge. While some truths are straightforward, others, like political opinions or socially nuanced situations, are more complex.
This complexity can be mitigated within a company. Organizations can define their own standards of truth and enforce clear expectations across operations. Once these standards are set, testing becomes more manageable, whether through human validation or automated systems – AI-driven or traditional – ensuring alignment with organizational goals.
The Ever-Present Threat of Data Security
As AI systems become more integrated into business processes, they also become more attractive targets for cyber-attacks. The stakes are high, especially when AI is used to handle sensitive information. Data breaches and cyber threats pose significant risks not only to the integrity of AI systems but also to the organizations that rely on them.
Safeguarding AI systems from these threats is a major concern for businesses and governments alike. Encryption, access controls, and regular security audits are essential to protecting AI systems from potential vulnerabilities. If you monitor your data quality effectively, you can identify anomalies caused by natural changes or malicious actors. Yet, many organizations are still playing catch-up in this area, leaving their AI deployments exposed to significant risks.
User Adoption and Change Management
Even when the technical challenges of AI adoption are addressed, organizations must still contend with human factors. Integrating AI with existing systems, processes, and people can be a daunting task. Compatibility and scalability issues often arise, making it difficult to achieve seamless integration.
Moreover, the impact of AI on the workforce cannot be ignored. Potential job displacement, the need for reskilling, and managing stakeholder expectations are significant challenges that organizations must navigate. Successfully driving user adoption requires a thoughtful approach to change management, one that considers the needs and concerns of all stakeholders.
Technology evolves at lightning speed, creating a mismatch between the pace of innovation and the speed of governance. To fully capitalize on AI’s potential, organizations must ensure that their data governance processes keep pace with technological advancements. Addressing these foundational issues is essential for unlocking AI’s true value and achieving long-term success.