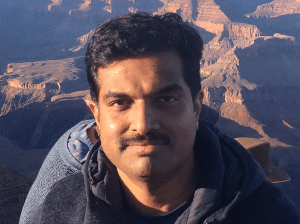
Imagine a world where AI can accurately predict earthquakes, giving us precious time to save lives. Yet, the same technology struggles to understand essential voice commands through your home assistant during a noisy family dinner. This striking dichotomy between the potential of cutting-edge AI research and its often underwhelming real-world applications underscores a significant yet frequently overlooked gap. As we stand on the brink of technological revolutions capable of profound societal impacts, this divide poses a severe challenge to the utility and acceptance of AI technologies.
The necessity to bridge this gap is more than a technical obligation – it is a critical step toward harnessing the full transformative power of AI. The technologies that dazzle in the lab should not falter in our living rooms and workplaces. They should be tools that enhance daily operations, improve quality of life, and solve intricate real-world problems. However, all too often, the complexity and brilliance of AI research fail to translate into practical solutions that work reliably outside controlled environments.
In recent years, the field of AI has witnessed remarkable advancements, with breakthroughs in quantum computing, deep learning, and neural networks promising revolutionary changes across various sectors. These technologies, underpinned by massive computational power and sophisticated algorithms, have demonstrated the potential to solve complex problems, from drug discovery to climate modeling, with unprecedented speed and accuracy. However, the transition of these cutting-edge developments into real-world applications often reveals a stark contrast. For instance, despite the prowess of deep learning in recognizing patterns and making predictions from vast datasets, many consumer applications like voice assistants or recommendation systems frequently suffer from errors and inefficiencies, struggling with the nuances of human language and behavior.
This disconnect between theoretical capability and practical usability primarily stems from several barriers. Technical barriers, for example, include the inherent complexity of state-of-the-art AI models, which, while powerful, require significant adaptation to function effectively outside the controlled conditions in research labs. These models often demand vast amounts of data and computing resources, which are not always feasible in everyday business environments. Economic and infrastructural barriers also play a significant role; there is often a considerable discrepancy in the funding and resources available for research versus those allocated for implementation. The development of infrastructure to support the deployment of advanced AI technologies – like data centers and network capabilities – lags considerably behind the pace of research innovations.
Moreover, cultural and organizational barriers further widen the chasm. There is a notable hesitance within many organizations to adopt new technologies, driven by fear of the unknown, lack of understanding of AI capabilities, and the disruption it represents to traditional workflows. This resistance is compounded by a workforce that may not be trained to integrate new AI tools effectively, resulting in a slow adoption curve that fails to leverage AI technologies’ benefits fully. The synergy between cutting-edge AI research and its application in real-world settings thus requires not just technological solutions but a holistic approach that addresses these multifaceted barriers.
Efforts to bridge the gap between AI research and real-world applications are underway, with industry initiatives and academic-industry partnerships playing pivotal roles. Leading tech companies and innovative startups are at the forefront of this endeavor, channeling cutting-edge AI into products and services that impact everyday life. For example, some tech giants have developed AI-driven platforms that enhance medical imaging techniques, significantly improving diagnostics in healthcare settings. Similarly, startups have leveraged machine learning algorithms to revolutionize everything from personalized education plans to efficient energy management systems in smart homes, demonstrating the practical utility of advanced AI.
Collaborations between academia and industry represent another critical avenue for addressing the divide. These partnerships often focus on combining the theoretical prowess of university researchers with companies’ pragmatic, market-driven approaches. Initiatives like joint research centers and technology hubs facilitate direct knowledge transfer and shared resources, accelerating the development of scalable AI solutions. Such collaborative projects expedite the translation of theoretical research into tangible products and ensure that innovations are aligned with real-world needs and constraints.
On a personal note, my connection to this issue is deeply rooted in my early experiences in the tech field, where I observed a striking disconnect between theoretical AI models and their practical applications. This gap stifled innovation and prevented potentially transformative technologies from reaching the people who needed them most. My journey in the tech industry has only deepened my conviction that without a concerted effort to bridge this divide, the true potential of AI may remain just out of reach for most of society.
Therefore, I propose specific measures to enhance the integration of AI research into practical applications. First, there should be increased cooperation between academia and industry to foster an environment where innovative ideas can be tested and refined in real-world scenarios. Second, funding for application-driven AI research must be significantly expanded to cover theoretical exploration and the development and scaling of technologies. Finally, it is crucial to cultivate an organizational culture that values and encourages continuous innovation and the adoption of new technologies.
In conclusion, the journey from AI labs to everyday life is fraught with challenges, from technical and infrastructural hurdles to cultural resistance. However, the ongoing efforts by tech companies, the burgeoning partnerships between academia and industry, and a growing recognition of the need for more practical focus in AI research are all positive signs that we are moving in the right direction.
As we continue to push the boundaries of what AI can achieve, it is essential to remember that the ultimate measure of this technology’s success will be its impact on society. How can we, as a collective of innovators, researchers, and everyday users, contribute to reducing the gap between AI potential and its practical benefits? This question should guide our efforts as we strive to ensure that AI serves as a force for good, enhancing people’s lives worldwide.