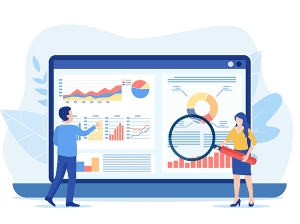
Data quality (DQ), which ensures that data is fit for business and consumer needs, remains a significant challenge and is growing more complex. According to a dbt Labs 2024 report, 57% of survey respondents identified data quality as a challenging aspect to data preparation, up from 41% in 2022.
To address these data quality challenges, companies increasingly turn to two approaches: data product or data as a product (DaaP). A data product defines something that achieves an end goal through data usage, such as dashboards, recommendation engines, or a generative AI model. In contrast, DaaP combines tools and a mindset that views and handles data as goods or a service for consumption.
Both options can solve data quality issues by improving data access and minimizing errors. However, each can exacerbate DQ issues if implemented poorly.
Choosing the best approach requires understanding the data product and DaaP differences and the specific DQ problems they address. These issues often extend beyond a single implementation.
The Data Quality Imperative: Why Guidance Is Needed
Data quality issues have many root causes, including integration issues, capturing inconsistencies, poor migration, data decay, or data duplication. Often, these challenges stem from a combination of these. For example, implementing a new data architecture upgrade with considerable changes to the business logic leads to inaccurate reports or dashboards.
While DQ problems can be addressed, achieving perfect data quality is nearly impossible. But organizations can focus on identifying and addressing their most critical and pressing needs.
In doing so, they will require well-defined business requirements, reproducible results and coverage of critical data elements (CDE). In essence, companies need a data quality program that clarifies what usable data looks like and establishes some general guidance to get there.
As organizations create and modify their data quality program, they need adaptable and comprehensible solutions. Here, a data product or DaaP methodology come into play. The data product takes a technological approach.
Data Product Defined
A data product describes a tool or application that delivers data for consumption to meet a specific business objective. For example, a data infrastructure upgrade may break reports and make them incomprehensible. A reporting data product, like a dashboard, may fix the issue.
Data products include multiple components (such as data sources, pipelines, models, and interfaces) that come together to leverage data for actionable insights and solutions, such as analytics dashboards and predictive models.
How Does a Data Product Address Poor Data Quality?
With an established definition of a data product, managers can now explore how it addresses poor data quality. Data products offer targeted solutions by leveraging their three strengths:
- Ensuring integrity is verified as part of data delivery
- Integrating databases and storage as part of system unification and accessibility
- Meeting targeted requests through the interface
For instance, consider a scenario where a data infrastructure upgrade breaks reports, making them incomprehensible. A high-level dashboard application could tackle this issue on multiple fronts.
It would guarantee data trust by automatically validating rules that transform and clean data. This would ensure data met requirements listed in DQ policies. Secondly, it would improve data integration by bridging gaps between new infrastructure and legacy data systems.
Also, a data product would also ensure accessibility through a user-friendly self-service interface. This functionality would allow for easy report customization and access, an elegant solution. While the data product provides a DQ fix, its complexity and culture can cause additional data quality issues.
Limitations of Data Product in Improving Data Quality
As mentioned, the adoption of a data product isn’t without its drawbacks. It can come with several key limitations:
- Focus Limitations: A data product may become too focused, addressing some issues but not the most important ones. Organizations might add more products to fix other DQ issues, leading to a fragmented approach.
- Complexity: Implementing multiple data products leads to cumbersome infrastructures. The data ecosystem then risks additional integration and scalability problems in data processing and storage.
- Cultural Resistance: Companies face cultural challenges in getting stakeholders to accept and use a data product. These issues can be compounded by poor communication about what data product(s) to build.
- Confusion About Requirements: Team members lack clarity on what to build. A survey uncovered misalignment among half about whether a data product includes analytics or AI capabilities
These drawbacks lead to wasted resources. For example, an organization brought a Tableau dashboard solution to display insights with good data quality. However, the organization faced cultural resistance.
The executives relied on email reports and asked for them back. But the critical DQ issue in the email reports remained. In cases where DQ has a large scope, as in a cultural change, organizations may find a data as a product (DaaP) is better suited.
Data as a Product (DaaP): A Paradigm Shift
Where a data product is a focused technique, data as a product (DaaP) is a methodology that views data as a stand-alone product. It focuses on its value, quality, and ability to meet the needs of various stakeholders within an organization. The DaaP methodology emerged as a part of a decentralized sociotechnical approach to data management, known as data mesh.
In this structure, domains (teams organized around a business function) own their data. They consider how data is collected and impacts end users, data consumers, and other stakeholders. This mindset combines people, technologies, and processes to create a stand-alone commodity with the data quality others need.
How the DaaP Approach Improves Data Quality
Taking a DaaP mindset naturally leads to data quality improvements. By treating data as an internal product, organizations are incentivized to maintain and improve its quality, much like they would with any other product they offer. Customers teams develop software products or services that internal teams trust and reuse.
Consequently, domains must continuously monitor their apps, using metrics to demonstrate accessibility, clarity, and security. Additionally, IBM defines a successful DaaP as: easily discoverable, addressable, well documented, and interoperable. This ensures data is findable, reliable, and usable across systems while maintaining protection.
For example, Netflix wanted to retain its subscribers and deliver high-quality, entertaining content. It developed a comprehensive data strategy that treated user behavior data as products.
Their culture embraces this data strategy and takes a multifaceted approach with people, technologies, and processes to make it happen. For example, Netflix has product analysts to dissect user behaviors, technologies to capture viewing patterns, and a sophisticated recommendation engine that makes entertainment data discoverable and accessible.
However, Netflix is a big enterprise with many resources. An organization tight on time and money would run into limitations with this kind of DaaP approach, demonstrating that the DaaP methodology has some drawbacks.
Limitations of a DaaP Approach
Some drawbacks of this methodology for a smaller organization include:
- Resource Intensity: DaaP implementation requires extensive skilled personnel for testing, validating, producing, and scaling products. Data scientists and engineers with these skills are scarce and expensive.
- Data Privacy and Literacy Challenges: Domains must address privacy concerns and data literacy gaps. Understanding automation technologies’ impact on access, security, and privacy is crucial for adherence to data laws and ethical processes.
- Organizational Resistance: Some domains in the organization may resist change to a DaaP approach and sharing of power in using data. Domains might compete for ownership of a critical data product, leading to data hoarding, silos, and poor data quality.
These drawbacks emphasize the importance of aligning DaaP with organizational culture. For example, overspending to implement DaaP when a targeted data product would suffice, in a more controlling environment, is counterproductive.
Conclusion
The decision between implementing a data product or adopting a data as a product (DaaP) approach is more than just a technical choice – it is a strategic decision that can significantly impact an organization’s data quality and overall data-driven capabilities. Such approaches rely on understanding DQ root issues, cultural alignment, and resource availability.
A data product tends to work best with:
- A centralized decision-making environment
- Clearly-defined DQ objectives
- Available resources to leverage data unification
In contrast, a data as a product (DaaP) adoption agrees with:
- Innovation and broad DQ concerns
- Work environment flexibility
- Cross-functional collaboration emphasis
Whatever solution(s) a leader decides to implement should meet the organization’s data quality imperative. In this perspective, organizations should view the implementation of data products or DaaP as an ongoing journey rather than a one-time decision. To stay ahead, companies must continuously learn about best DQ practices so their guidance can remain relevant.