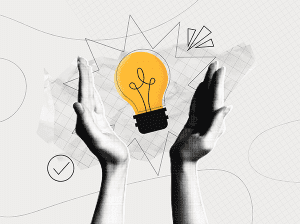
No matter how good you feel about the reliability of your company’s data operations, you can’t help wondering on occasion what the chances are that your firm will be one of the victims of the next data outage, malware scourge, or cyberattack. This is especially the case if your job entails ensuring the availability of your organization’s data systems and the usefulness of its data in support of its day-to-day operations.
By staying alert to the early warnings of shortcomings in an enterprise’s data processes and practices, managers are able to get the jump on little problems before they affect users. Close monitoring is also the key to locating opportunities to apply simple tweaks and upgrades that improve data operations. If you find any of these five common data management questions crossing your mind, read on to learn how – and why – to build an enterprise data strategy.
Top 5 Data Management Challenges Faced by Enterprises
The surefire way to guarantee a data failure is by doing nothing and allowing entropy to introduce disorder and uncertainty over time. Thankfully, not many IT managers wait for things to break before acting. Standard data monitoring and maintenance prevents most potential data glitches from occurring, but that doesn’t mean certain questions don’t keep IT staff awake at night. If you find these thoughts preventing you from enjoying a good night’s sleep, your enterprise data strategy could be overdue for a revamp.
“Where’s my data?” Data discovery plays a key role in helping organizations overcome data silos. The three-step process begins with data preparation to merge unstructured data from diverse sources and get it ready for use by your systems. Then, you can use analytics tools to visualize the data and identify patterns and relationships in the data. Finally, you can generate reports based on descriptive statistics to support business decisions.
“Is my data as useful as it needs to be?” Establishing and maintaining data quality requires defining and applying quality metrics. Three approaches to ensuring data quality are data profiling, data stewardship, and data preparation:
- Data profiling involves structure discovery, content discovery, and relationship discovery.
- Data stewardship oversees all aspects of data management: from creation to deletion. It requires knowing and documenting all data types, identifying where all data assets are located, and monitoring the data to ensure its quality and integrity.
- Data preparation cleans and transforms raw data so that it’s ready to be processed and analyzed. It involves gathering and assessing the data, removing faulty and extraneous aspects, validating it, transforming it to enhance its value, and safely storing it.
“Are we in compliance with data regulations?” The first step in data compliance is knowing which laws related to data collection, storage, and use apply to your company. Then you identify the tools and processes that will confirm that your data operations comply with all applicable regulations, as well as with your internal data policies. The three greatest challenges to ensuring compliant data systems are the indiscriminate use of personal information in generative AI, the lack of simple and trustworthy consent models, and security threats originating inside the company.
“Is my data safe?” Many regulations are intended to minimize the risk of sensitive data being stolen and misused, but data security entails protecting the integrity of the data itself to ensure that your company is able to realize its full value. The insatiable appetite of generative AI and large language models in machine learning highlights the need to have data integrity checks in place in all strategic data systems.
“How can we make our data processes more accurate and efficient?” Data automation practices now extend beyond standard extract, transform, load (ETL) to support alternative models of data access and ownership. Automation promises to enhance the accuracy of data by reducing human error and creating data pipelines that scale automatically. This avoids bottlenecks and protects against failures by applying redundancy, fault tolerance, and reliable recovery strategies.
Assembling the Components of a Winning Enterprise Data Strategy
Your data strategy is intended to serve as a roadmap that clearly describes the route to achieving the company’s data-related goals. The roadmap covers the roles played by individuals in the organization, the timeline for reaching its goals, and the money and equipment its data projects require. Devising the strategy begins with an assessment of the current status of all data systems and the identification of necessary upgrades and modifications to meet all three requirements.
The five primary components of an enterprise data strategy are data governance, infrastructure, analytics, talent management, and security and privacy:
- Data governance serves as the foundation of the strategy by providing guidelines for data accuracy, reliability, and consistency. Governance defines the policies and best practices applied to data collection, storage, and application. It also delineates the roles, responsibilities, and permissions of individuals to ensure compliance with regulations and with the organization’s internal controls for accountability and transparency.
- The data framework’s infrastructure encompasses all hardware, software, and network equipment used to collect, process, analyze, and protect the large data stores that the company relies on. Scalability and flexibility are built into the infrastructure by tapping the benefits of onsite and cloud resources, whether in an in-house, cloud, or hybrid environment.
- Analytics are key to unlocking the value of your organization’s data assets in supporting management decisions. Companies choose a mix of descriptive, predictive, diagnostic, and prescriptive analytics techniques based on the unique characteristics of their short-term and long-term goals. Machine learning and other AI techniques have their greatest impact in driving data-based business decision-making.
- Talent management ensures that the data professionals charged with defining, implementing, and monitoring the enterprise’s data strategy possess the tools and skills required to fulfill their duties. Continuous learning and professional development become increasingly important as effective implementation of AI and other technologies continue to evolve at an ever-increasing pace. Equally important is providing data workers with an environment that fosters collaboration and innovation.
- Security and privacy face the hurdles of skyrocketing data volumes, growing reliance on advanced analytics, and new threats to the data. The enterprise data strategy lays out required data security measures to protect against breaches, unauthorized access, and misuse. In addition to standard encryption, access controls, and regular security audits, the strategy focuses on maintaining the trust of customers and business partners.
When it comes to managing the data at the heart of the strategy, SAS Institute identifies five requirements related specifically to data storage: identify, store, provision, integrate, and govern:
- Intelligent data storage begins by understanding the meaning and significance of data elements whatever their structure, origin, or location. Metadata plays a key role in identifying data and making data easy to discover and access.
- Many organizations focus their storage management efforts on data when it’s created and downplay the impact of storage on data sharing and usage. The transactional aspect of data sharing between discrete applications is giving way to bulk data sharing in large volumes to support advanced analytics and machine learning projects.
- A new approach is required to provision data so that it can be shared easily across applications and entire data systems. Conventional application development frequently locks data inside and creates hurdles to sharing. Making application data easy to package and share must become the standard approach in application development.
- Similarly, the techniques developers use to integrate data values across multiple sources and systems rely on building logic on a case-by-case basis, which is inefficient and time-consuming. Existing efforts to reuse code and promote collaboration in app development suffer from lack of a single, overarching approach to data integration for developers.
- Current data governance initiatives promoting data accuracy, business rules, and standard terminologies are limited to tactical approaches for specific apps or data systems. What’s needed are uniform information policies, rules, and methods that apply across and beyond the enterprise.
Putting Your Enterprise Data Strategy into Practice
After completing the assessment of your current data management efforts and defining your objectives and priorities, you can begin to assemble the data governance framework by defining roles, responsibilities, and procedures for the entire data lifecycle. This includes data ownership, access controls, security, and compliance as well as data consistency, accountability, and integrity.
The next step is to establish the processes and tools used to manage data quality, which include data profiling, cleansing, standardization, and validation. Determine the mechanisms for integrating data to create a seamless and coherent data environment encompassing the entire enterprise. Data lifecycle management covers data retention policies, archival storage, and data purging to ensure efficient storage management.
The glue that keeps the many moving pieces of an enterprise data strategy working together harmoniously is your company’s culture of data literacy and empowerment. Employees and managers must be trained to recognize the value of data to the organization, and the importance of maintaining its quality and security. They must also understand how their roles in data management intersect with those of others, directly and indirectly.
It’s no coincidence that “lack of data culture” tops the list of the most common data strategy pitfalls. Data may be driving modern businesses, but it’s the people in your company who transform data into revenue. A successful enterprise data strategy optimizes the value of your data assets and your human ones, too.