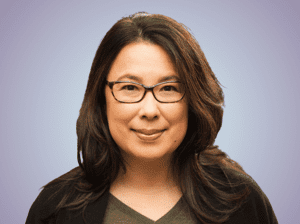
One of the most important questions about using AI responsibly has very little to do with data, models, or anything technical. It has to do with the power of a captivating story about magical thinking. There’s a set of beliefs driven by over-exuberant AI hype – that AI is going to revolutionize everything!
How can we set realistic expectations about AI in our organization?
I hear this question often, primarily from people who work on data teams or who are tasked with implementing AI solutions. Typically their tales involve a senior executive becoming enamored with the idea of AI in ways that are not necessarily reflective of reality and don’t fully account for costs or risks. When the organization attempts to implement the solution, the results are either lackluster or in some cases, a complete failure. The gap between AI hype and operational reality is a wide and deep chasm. Yet, by fostering discussions about what is actually possible and proactively weighing the benefits, costs, and risks, data leaders can help executive leadership to make better decisions.
Saying the Quiet Part Out Loud
If those who understand the realities could say the quiet part out loud, it might sound like this …
Dear Senior Executive,
It’s apparent that you have recently become a true believer in the power of AI to transform the business. It’s a message you’ve likely heard from “respected” sources that inform your perspective, like research firms, business schools, management consultants, venture capitalists, and tech leaders. The gospel of AI has swept through management circles like a virus on a cruise ship.
You are now fully onboard in believing that AI will make our organization more efficient and productive in every way. Not only will it drive down costs, it will also open up exciting new growth opportunities. Opting out is not an option because AI is inevitable. The choice is obvious – we must implement AI now or be left behind.
This is why you are determined to make our organization “AI-first,” and by AI, you mostly mean generative AI and chatbots. All of this is clearly outlined in your recent email about Project Gamechanger. The marketing team has already done its work to support this vision by supplying compelling copy and dazzling slide decks. Now, it’s up to the data and technology teams to actually make it happen.
BUT, before we sink millions into this strategy and make plans to replace the entire customer service team with a chatbot, there are some things you should consider …
Now that the scene is set, here are some actual messages you can bring to the conversation with your organizational leadership to help drive more realistic expectations around AI.
Determine the ROI for AI
Most business people are at least a little familiar with the Gartner hype cycle – the idea that technologies move from early promising results to a peak of “inflated expectations” into a trough of “disillusionment” before leveling off to some useful stable state. A recent report from Goldman Sachs captures the idea that we might be in the early stages of descending the peak and heading into the trough. Jim Covello, the head of global equity research at Goldman Sachs, shared with Business Insider that:
- “AI technology is exceptionally expensive, and to justify those costs, the technology must be able to solve complex problems, which it isn’t designed to do,”
- “The starting point for costs is also so high that even if costs decline, they would have to do so dramatically to make automating tasks with AI affordable,”
- “In our experience, even basic summarization tasks often yield illegible and nonsensical results.”
In essence, the ROI for AI is questionable. Gartner’s Chief of Global Research, Chris Howard, also shares this sentiment in this explainer video Decoding the AI Hype Cycle. Both Covello and Howard point toward the link between the incredibly high compute and data center costs to make today’s AI work, with Howard noting that it’s not just a financial cost but also an environmental cost.
This does not mean that all AI is a waste of time and money. It does point out, though, that hype-fueled adoption without a clear business case other than “speed and greed” is going to yield bad results. That should not be surprising.
Generative AI Does Not Equal All of AI
It’s foolish to think that a hammer is equivalent to having a full set of tools. It’s simply one tool in the toolbox. Similarly, AI is an umbrella term that represents a range of technologies – a toolbox full of different tools. Generative AI is just one approach or type of AI. As the name suggests, it is useful for generating novel (but not always accurate) content. If your use case is not a fit for what this technology can do, why use it? Why spend time and money trying to use a hammer like a saw? If what you need is to segment data to understand customer behavior, to have an automated system monitor for anomalies or to predict sales trends for your products – generative AI is not a good choice.
Even if content generation fits your use case, the risks of generative AI might be unacceptable. Hallucinations – the propensity of generative AI to provide inaccurate, offensive, or dangerously wrong information – are a known risk and should not come as a surprise. It’s not guaranteed that techniques like retrieval augmented generation (RAG) or fine tuning will solve all of these problems. There are also privacy and cybersecurity risks to consider. Conducting a full cost benefit and risk assessment is essential.
Use Data Effectively
“‘AI-powered’ is tech’s meaningless equivalent of ‘all natural.’” –Devin Coldewey, TechCrunch
AI-powered is a vaguely applied and meaningless marketing term. It can mean whatever a vendor wants it to mean, and increasingly it translates to “we sell chatbots – you should get one.” There are also vendors who claim their system is fully automated when really the work has just been outsourced to other humans that are paid less to do it.
If we look beyond the AI hype, we see that organizations really want to use data in ways that can benefit their business. Sometimes simple data analytics might be what is most effective. In other cases, perhaps machine learning is required, or maybe even a chatbot. The point is that there are too many misaligned incentives with AI hype to induce leaders to just rush out and sign up for whatever an “AI-powered” vendor happens to be selling. Instead, leadership should go back to basics to consider how to use data most effectively in their organization:
- Start with the business problem that needs to be solved
- Realistically assess the role that data and automation (aka “AI”) can play
- Determine the ROI on AI – don’t assume it’s all upside
- Understand organizational readiness and capacity for using data and for AI adoption
- Ensure risks are clearly assessed and accounted for in the analysis, including AI governance as part of ongoing risk mitigation
- Set realistic time frames and dispense of FOMO-driven deadlines
Exploring these topics and having these kinds of conversations can help people see beyond the AI snake oil to the real opportunities and challenges for your organization. Leverage these messages about AI realities to have this conversation with your organization’s leadership.
Send Me Your Questions!
I would love to hear about your data dilemmas or AI ethics questions and quandaries. You can send me a note at hello@ethicallyalignedai.com or connect with me on LinkedIn. I will keep all inquiries confidential and remove any potentially sensitive information – so please feel free to keep things high level and anonymous as well.
This column is not legal advice. The information provided is strictly for educational purposes. AI and data regulation is an evolving area and anyone with specific questions should seek advice from a legal professional.